On the Role of Supervision in Unsupervised Constituency Parsing
Haoyue Shi, Karen Livescu, Kevin Gimpel
Syntax: Tagging, Chunking, and Parsing Short Paper
You can open the pre-recorded video in a separate window.
Abstract:
We analyze several recent unsupervised constituency parsing models, which are tuned with respect to the parsing F1 score on the Wall Street Journal (WSJ) development set (1,700 sentences). We introduce strong baselines for them, by training an existing supervised parsing model (Kitaev and Klein, 2018) on the same labeled examples they access. When training on the 1,700 examples, or even when using only 50 examples for training and 5 for development, such a few-shot parsing approach can outperform all the unsupervised parsing methods by a significant margin. Few-shot parsing can be further improved by a simple data augmentation method and self-training. This suggests that, in order to arrive at fair conclusions, we should carefully consider the amount of labeled data used for model development. We propose two protocols for future work on unsupervised parsing: (i) use fully unsupervised criteria for hyperparameter tuning and model selection; (ii) use as few labeled examples as possible for model development, and compare to few-shot parsing trained on the same labeled examples.
NOTE: Video may display a random order of authors.
Correct author list is at the top of this page.
Connected Papers in EMNLP2020
Similar Papers
XL-WiC: A Multilingual Benchmark for Evaluating Semantic Contextualization
Alessandro Raganato, Tommaso Pasini, Jose Camacho-Collados, Mohammad Taher Pilehvar,
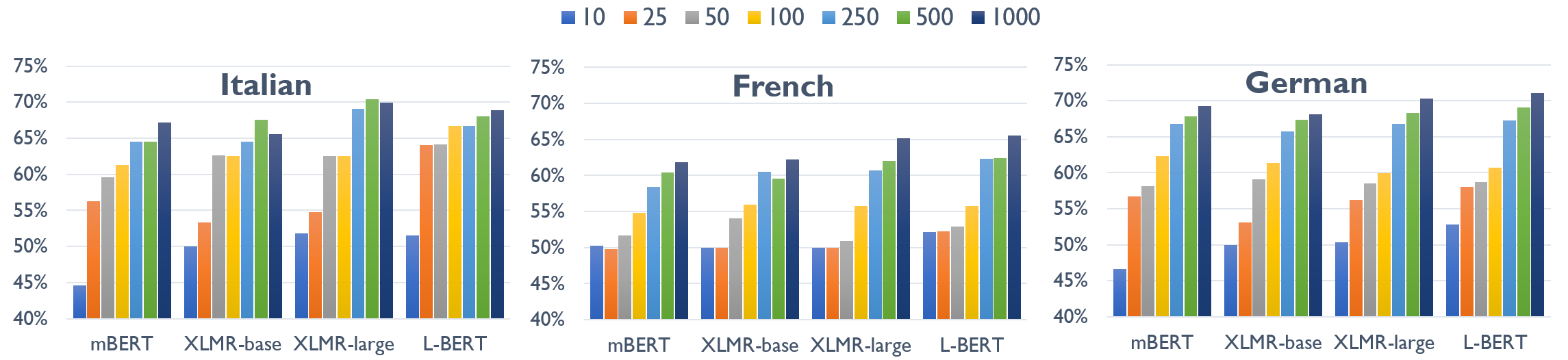
Syntactic Structure Distillation Pretraining for Bidirectional Encoders
Adhiguna Kuncoro, Lingpeng Kong, Daniel Fried, Dani Yogatama, Laura Rimell, Chris Dyer, Phil Blunsom,

Combining Self-Training and Self-Supervised Learning for Unsupervised Disfluency Detection
Shaolei Wang, Zhongyuan Wang, Wanxiang Che, Ting Liu,

Structural Supervision Improves Few-Shot Learning and Syntactic Generalization in Neural Language Models
Ethan Wilcox, Peng Qian, Richard Futrell, Ryosuke Kohita, Roger Levy, Miguel Ballesteros,
