Recurrent Event Network: Autoregressive Structure Inferenceover Temporal Knowledge Graphs
Woojeong Jin, Meng Qu, Xisen Jin, Xiang Ren
NLP Applications Long Paper
You can open the pre-recorded video in a separate window.
Abstract:
Knowledge graph reasoning is a critical task in natural language processing. The task becomes more challenging on temporal knowledge graphs, where each fact is associated with a timestamp. Most existing methods focus on reasoning at past timestamps and they are not able to predict facts happening in the future. This paper proposes Recurrent Event Network (RE-Net), a novel autoregressive architecture for predicting future interactions. The occurrence of a fact (event) is modeled as a probability distribution conditioned on temporal sequences of past knowledge graphs. Specifically, our RE-Net employs a recurrent event encoder to encode past facts, and uses a neighborhood aggregator to model the connection of facts at the same timestamp. Future facts can then be inferred in a sequential manner based on the two modules. We evaluate our proposed method via link prediction at future times on five public datasets. Through extensive experiments, we demonstrate the strength of RE-Net, especially on multi-step inference over future timestamps, and achieve state-of-the-art performance on all five datasets.
NOTE: Video may display a random order of authors.
Correct author list is at the top of this page.
Connected Papers in EMNLP2020
Similar Papers
Temporal Knowledge Base Completion: New Algorithms and Evaluation Protocols
Prachi Jain, Sushant Rathi, Mausam, Soumen Chakrabarti,
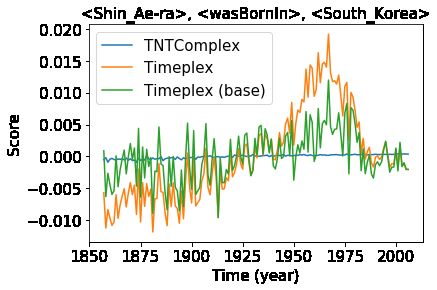
Domain Knowledge Empowered Structured Neural Net for End-to-End Event Temporal Relation Extraction
Rujun Han, Yichao Zhou, Nanyun Peng,
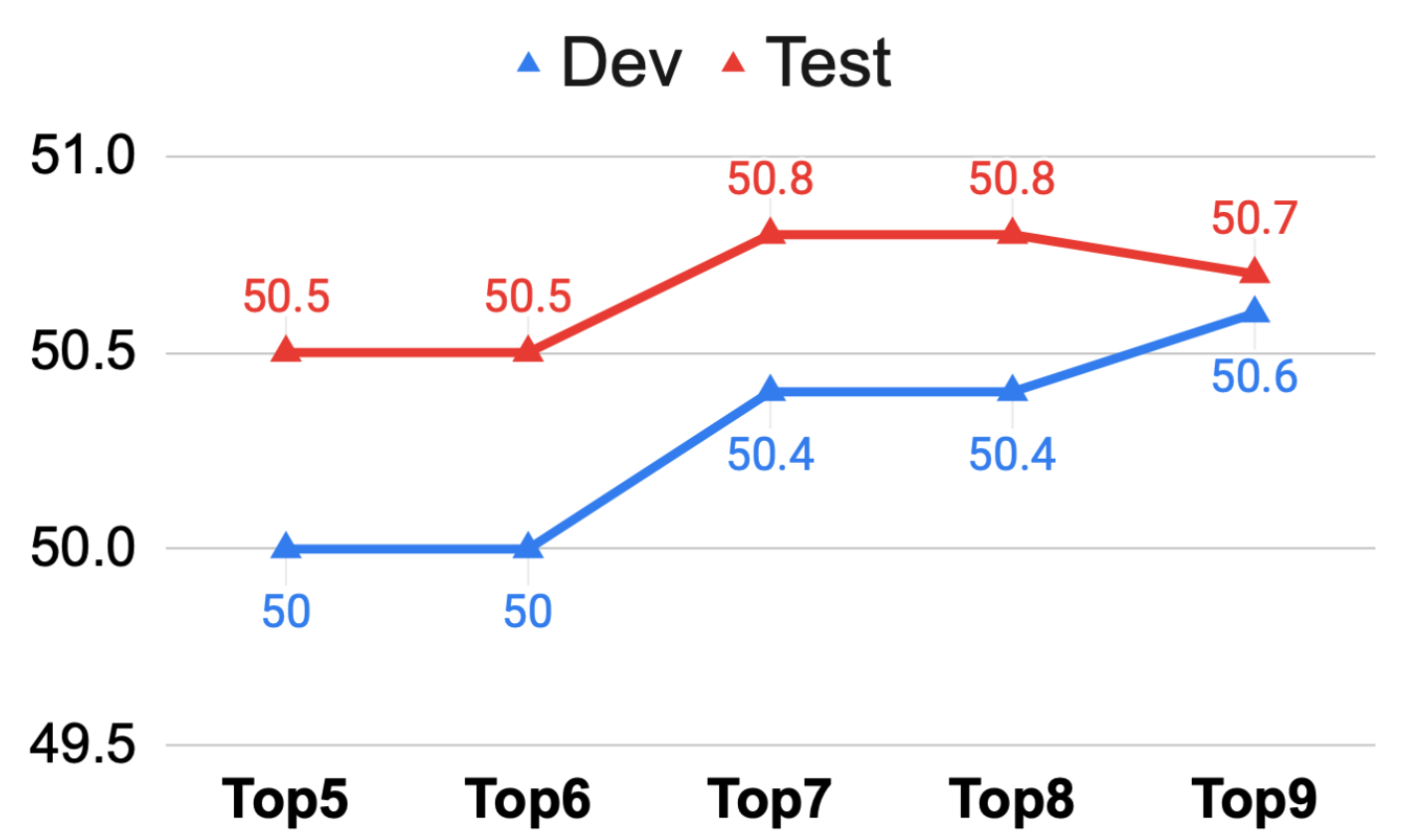
Connecting the Dots: Event Graph Schema Induction with Path Language Modeling
Manling Li, Qi Zeng, Ying Lin, Kyunghyun Cho, Heng Ji, Jonathan May, Nathanael Chambers, Clare Voss,

Joint Constrained Learning for Event-Event Relation Extraction
Haoyu Wang, Muhao Chen, Hongming Zhang, Dan Roth,
