Joint Constrained Learning for Event-Event Relation Extraction
Haoyu Wang, Muhao Chen, Hongming Zhang, Dan Roth
Information Extraction Long Paper
You can open the pre-recorded video in a separate window.
Abstract:
Understanding natural language involves recognizing how multiple event mentions structurally and temporally interact with each other. In this process, one can induce event complexes that organize multi-granular events with temporal order and membership relations interweaving among them. Due to the lack of jointly labeled data for these relational phenomena and the restriction on the structures they articulate, we propose a joint constrained learning framework for modeling event-event relations. Specifically, the framework enforces logical constraints within and across multiple temporal and subevent relations of events by converting these constraints into differentiable learning objectives. We show that our joint constrained learning approach effectively compensates for the lack of jointly labeled data, and outperforms SOTA methods on benchmarks for both temporal relation extraction and event hierarchy construction, replacing a commonly used but more expensive global inference process. We also present a promising case study to show the effectiveness of our approach to inducing event complexes on an external corpus.
NOTE: Video may display a random order of authors.
Correct author list is at the top of this page.
Connected Papers in EMNLP2020
Similar Papers
Connecting the Dots: Event Graph Schema Induction with Path Language Modeling
Manling Li, Qi Zeng, Ying Lin, Kyunghyun Cho, Heng Ji, Jonathan May, Nathanael Chambers, Clare Voss,

Analogous Process Structure Induction for Sub-event Sequence Prediction
Hongming Zhang, Muhao Chen, Haoyu Wang, Yangqiu Song, Dan Roth,
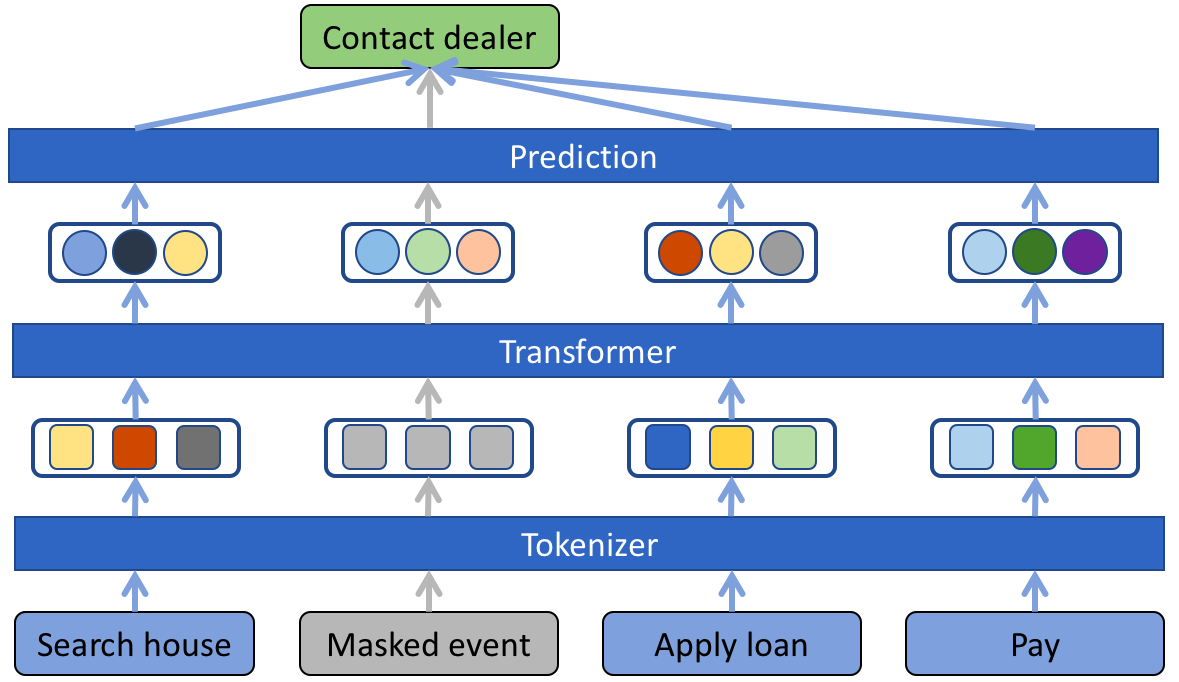
Weakly Supervised Subevent Knowledge Acquisition
Wenlin Yao, Zeyu Dai, Maitreyi Ramaswamy, Bonan Min, Ruihong Huang,
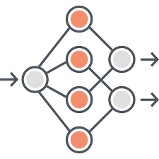
Exploring Contextualized Neural Language Models for Temporal Dependency Parsing
Hayley Ross, Jonathon Cai, Bonan Min,
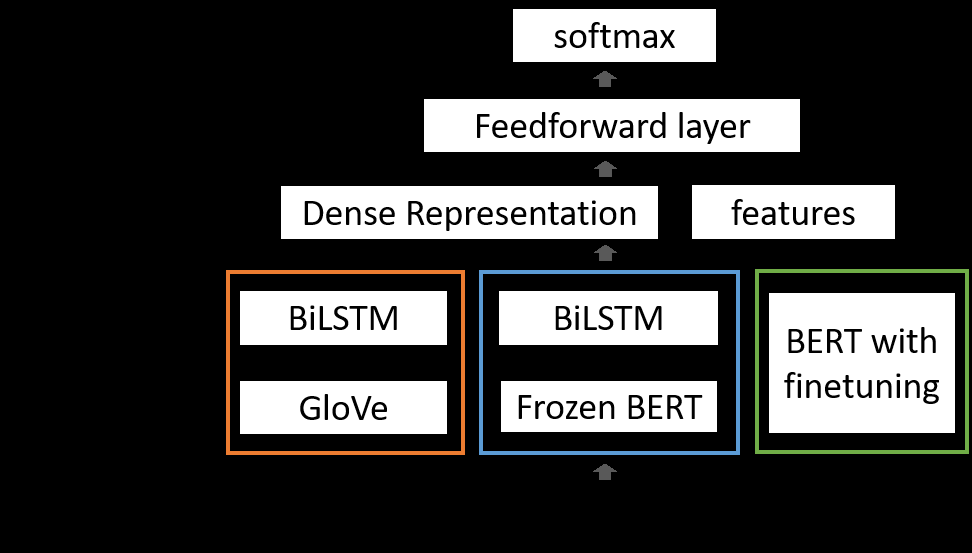