Adversarial Self-Supervised Data-Free Distillation for Text Classification
Xinyin Ma, Yongliang Shen, Gongfan Fang, Chen Chen, Chenghao Jia, Weiming Lu
Machine Learning for NLP Long Paper
You can open the pre-recorded video in a separate window.
Abstract:
Large pre-trained transformer-based language models have achieved impressive results on a wide range of NLP tasks. In the past few years, Knowledge Distillation(KD) has become a popular paradigm to compress a computationally expensive model to a resource-efficient lightweight model. However, most KD algorithms, especially in NLP, rely on the accessibility of the original training dataset, which may be unavailable due to privacy issues. To tackle this problem, we propose a novel two-stage data-free distillation method, named Adversarial self-Supervised Data-Free Distillation (AS-DFD), which is designed for compressing large-scale transformer-based models (e.g., BERT). To avoid text generation in discrete space, we introduce a Plug & Play Embedding Guessing method to craft pseudo embeddings from the teacher's hidden knowledge. Meanwhile, with a self-supervised module to quantify the student's ability, we adapt the difficulty of pseudo embeddings in an adversarial training manner. To the best of our knowledge, our framework is the first data-free distillation framework designed for NLP tasks. We verify the effectiveness of our method on several text classification datasets.
NOTE: Video may display a random order of authors.
Correct author list is at the top of this page.
Connected Papers in EMNLP2020
Similar Papers
Effective Unsupervised Domain Adaptation with Adversarially Trained Language Models
Thuy-Trang Vu, Dinh Phung, Gholamreza Haffari,
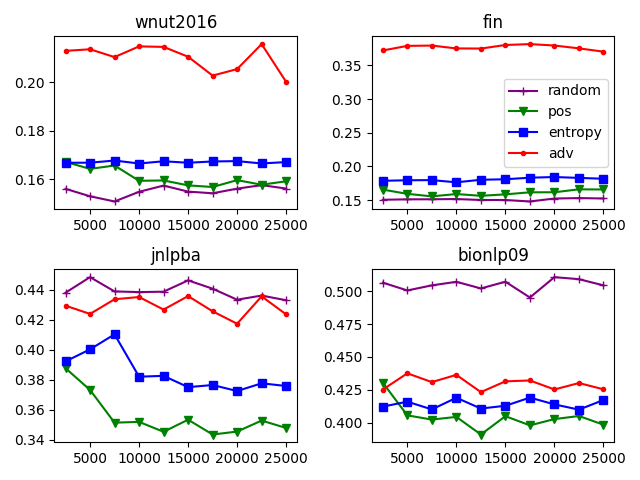
CAT-Gen: Improving Robustness in NLP Models via Controlled Adversarial Text Generation
Tianlu Wang, Xuezhi Wang, Yao Qin, Ben Packer, Kang Li, Jilin Chen, Alex Beutel, Ed Chi,

Why Skip If You Can Combine: A Simple Knowledge Distillation Technique for Intermediate Layers
Yimeng Wu, Peyman Passban, Mehdi Rezagholizadeh, Qun Liu,
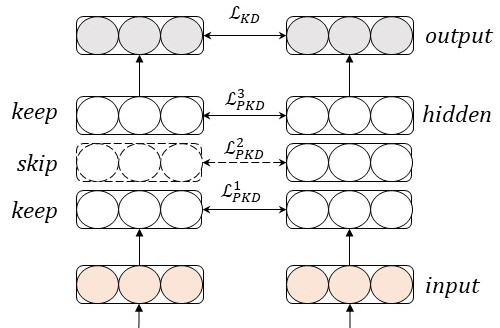
Local Additivity Based Data Augmentation for Semi-supervised NER
Jiaao Chen, Zhenghui Wang, Ran Tian, Zichao Yang, Diyi Yang,
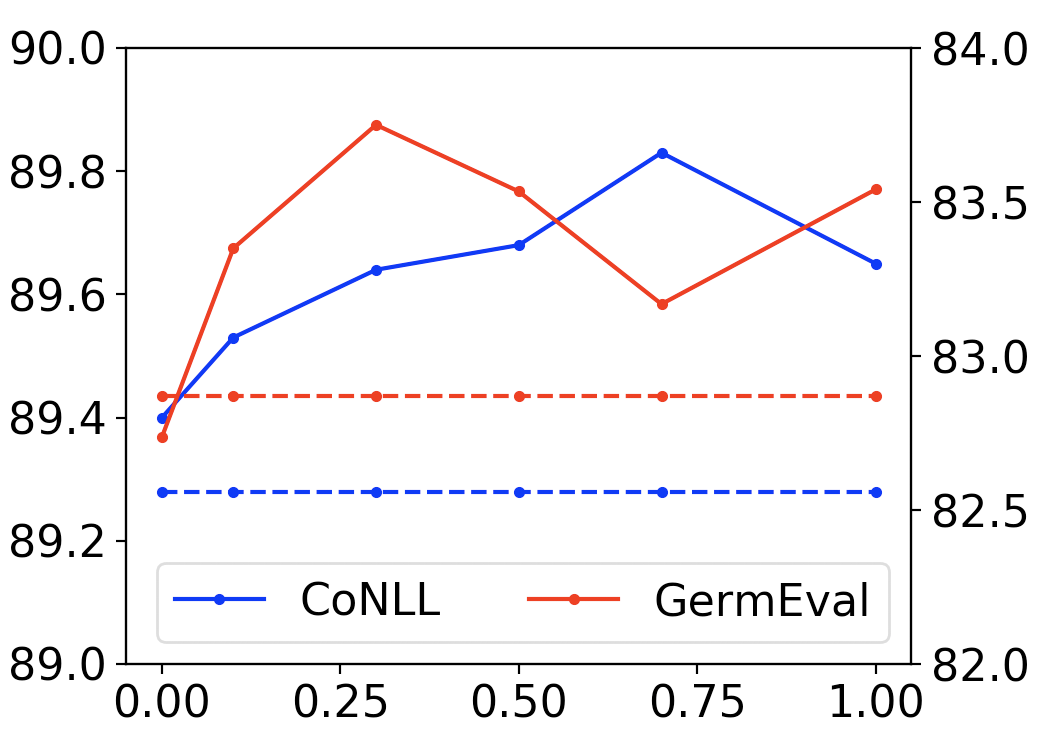