Analogous Process Structure Induction for Sub-event Sequence Prediction
Hongming Zhang, Muhao Chen, Haoyu Wang, Yangqiu Song, Dan Roth
Semantics: Sentence-level Semantics, Textual Inference and Other areas Long Paper
You can open the pre-recorded video in a separate window.
Abstract:
Computational and cognitive studies of event understanding suggest that identifying, comprehending, and predicting events depend on having structured representations of a sequence of events and on conceptualizing (abstracting) its components into (soft) event categories. Thus, knowledge about a known process such as ``buying a car'' can be used in the context of a new but analogous process such as ``buying a house''. Nevertheless, most event understanding work in NLP is still at the ground level and does not consider abstraction. In this paper, we propose an Analogous Process Structure Induction (APSI) framework, which leverages analogies among processes and conceptualization of sub-event instances to predict the whole sub-event sequence of previously unseen open-domain processes. As our experiments and analysis indicate, APSI supports the generation of meaningful sub-event sequences for unseen processes and can help predict missing events.
NOTE: Video may display a random order of authors.
Correct author list is at the top of this page.
Connected Papers in EMNLP2020
Similar Papers
Weakly Supervised Subevent Knowledge Acquisition
Wenlin Yao, Zeyu Dai, Maitreyi Ramaswamy, Bonan Min, Ruihong Huang,
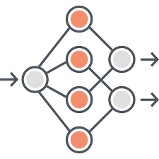
Connecting the Dots: Event Graph Schema Induction with Path Language Modeling
Manling Li, Qi Zeng, Ying Lin, Kyunghyun Cho, Heng Ji, Jonathan May, Nathanael Chambers, Clare Voss,

Reasoning about Goals, Steps, and Temporal Ordering with WikiHow
Li Zhang, Qing Lyu, Chris Callison-Burch,
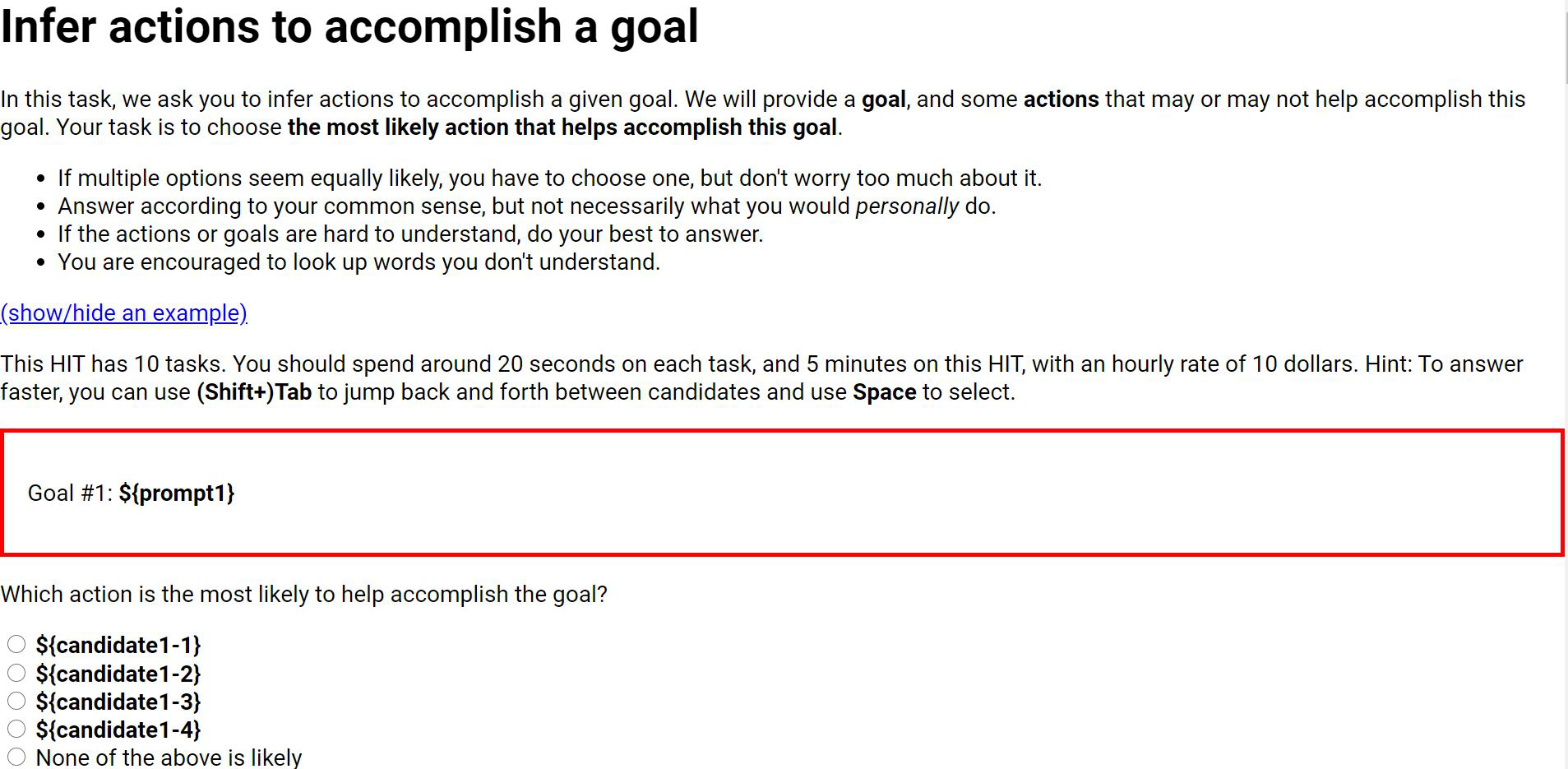