Exploring Contextualized Neural Language Models for Temporal Dependency Parsing
Hayley Ross, Jonathon Cai, Bonan Min
Information Extraction Short Paper
You can open the pre-recorded video in a separate window.
Abstract:
Extracting temporal relations between events and time expressions has many applications such as constructing event timelines and time-related question answering. It is a challenging problem which requires syntactic and semantic information at sentence or discourse levels, which may be captured by deep contextualized language models (LMs) such as BERT (Devlin et al., 2019). In this paper, we develop several variants of BERT-based temporal dependency parser, and show that BERT significantly improves temporal dependency parsing (Zhang and Xue, 2018a). We also present a detailed analysis on why deep contextualized neural LMs help and where they may fall short. Source code and resources are made available at https://github.com/bnmin/tdp_ranking.
NOTE: Video may display a random order of authors.
Correct author list is at the top of this page.
Connected Papers in EMNLP2020
Similar Papers
Domain Knowledge Empowered Structured Neural Net for End-to-End Event Temporal Relation Extraction
Rujun Han, Yichao Zhou, Nanyun Peng,
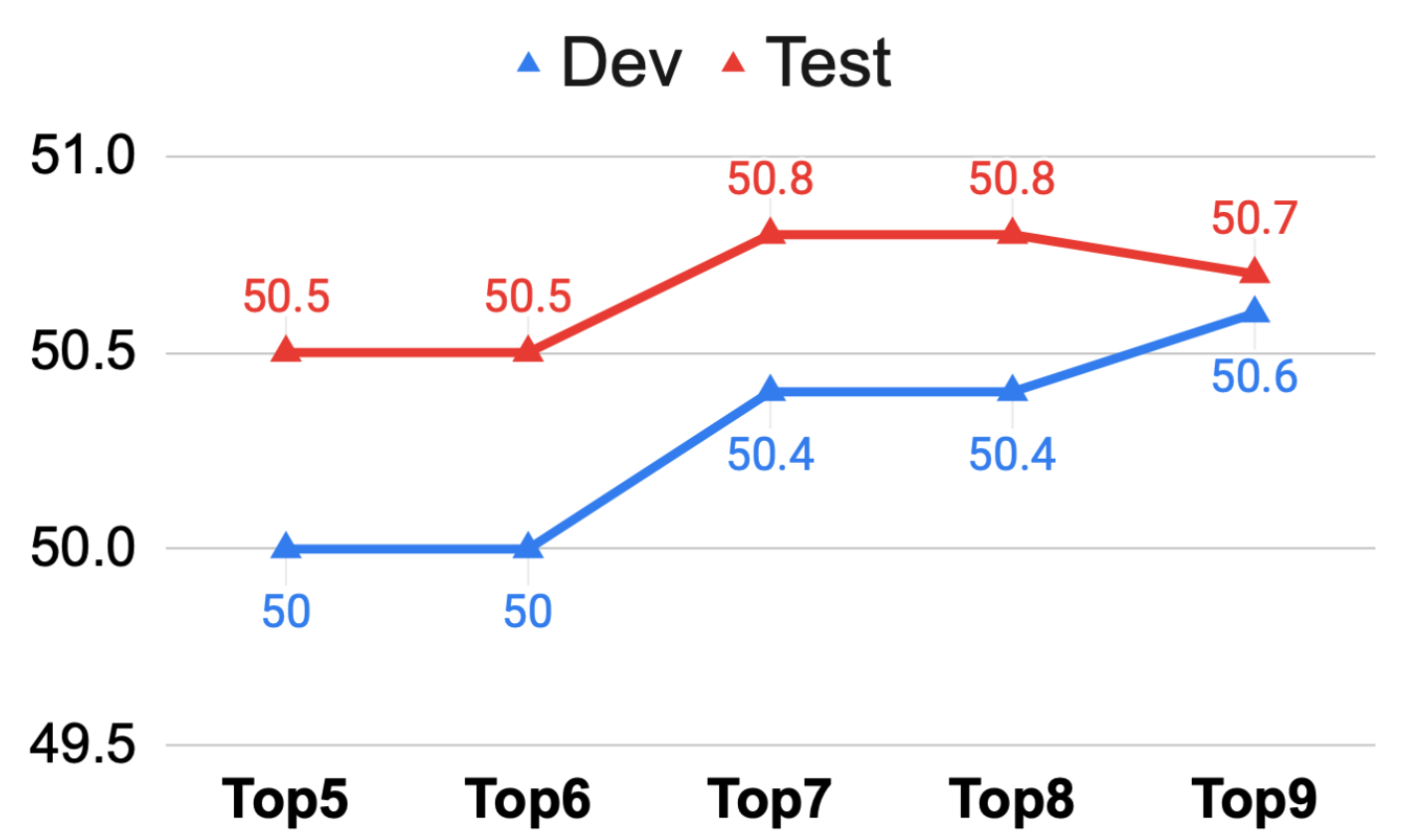
Coreferential Reasoning Learning for Language Representation
Deming Ye, Yankai Lin, Jiaju Du, Zhenghao Liu, Peng Li, Maosong Sun, Zhiyuan Liu,

Conversational Semantic Parsing for Dialog State Tracking
Jianpeng Cheng, Devang Agrawal, Héctor Martínez Alonso, Shruti Bhargava, Joris Driesen, Federico Flego, Dain Kaplan, Dimitri Kartsaklis, Lin Li, Dhivya Piraviperumal, Jason D. Williams, Hong Yu, Diarmuid Ó Séaghdha, Anders Johannsen,
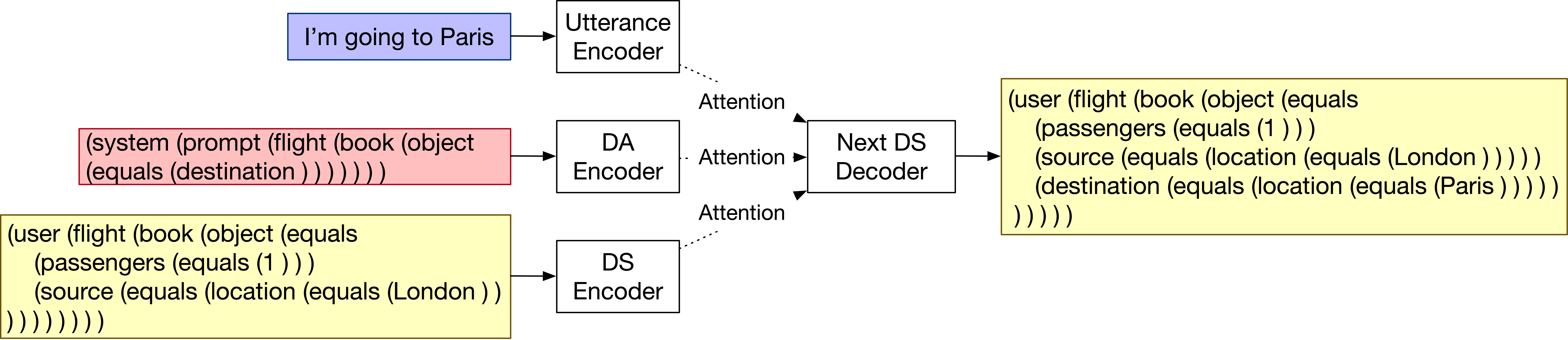