STL-CQA: Structure-based Transformers with Localization and Encoding for Chart Question Answering
Hrituraj Singh, Sumit Shekhar
Language Grounding to Vision, Robotics and Beyond Long Paper
You can open the pre-recorded video in a separate window.
Abstract:
Chart Question Answering (CQA) is the task of answering natural language questions about visualisations in the chart image. Recent solutions, inspired by VQA approaches, rely on image-based attention for question/answering while ignoring the inherent chart structure. We propose STL-CQA which improves the question/answering through sequential elements localization, question encoding and then, a structural transformer-based learning approach. We conduct extensive experiments while proposing pre-training tasks, methodology and also an improved dataset with more complex and balanced questions of different types. The proposed methodology shows a significant accuracy improvement compared to the state-of-the-art approaches on various chart Q/A datasets, while outperforming even human baseline on the DVQA Dataset. We also demonstrate interpretability while examining different components in the inference pipeline.
NOTE: Video may display a random order of authors.
Correct author list is at the top of this page.
Connected Papers in EMNLP2020
Similar Papers
Unsupervised Question Decomposition for Question Answering
Ethan Perez, Patrick Lewis, Wen-tau Yih, Kyunghyun Cho, Douwe Kiela,

Stepwise Extractive Summarization and Planning with Structured Transformers
Shashi Narayan, Joshua Maynez, Jakub Adamek, Daniele Pighin, Blaz Bratanic, Ryan McDonald,
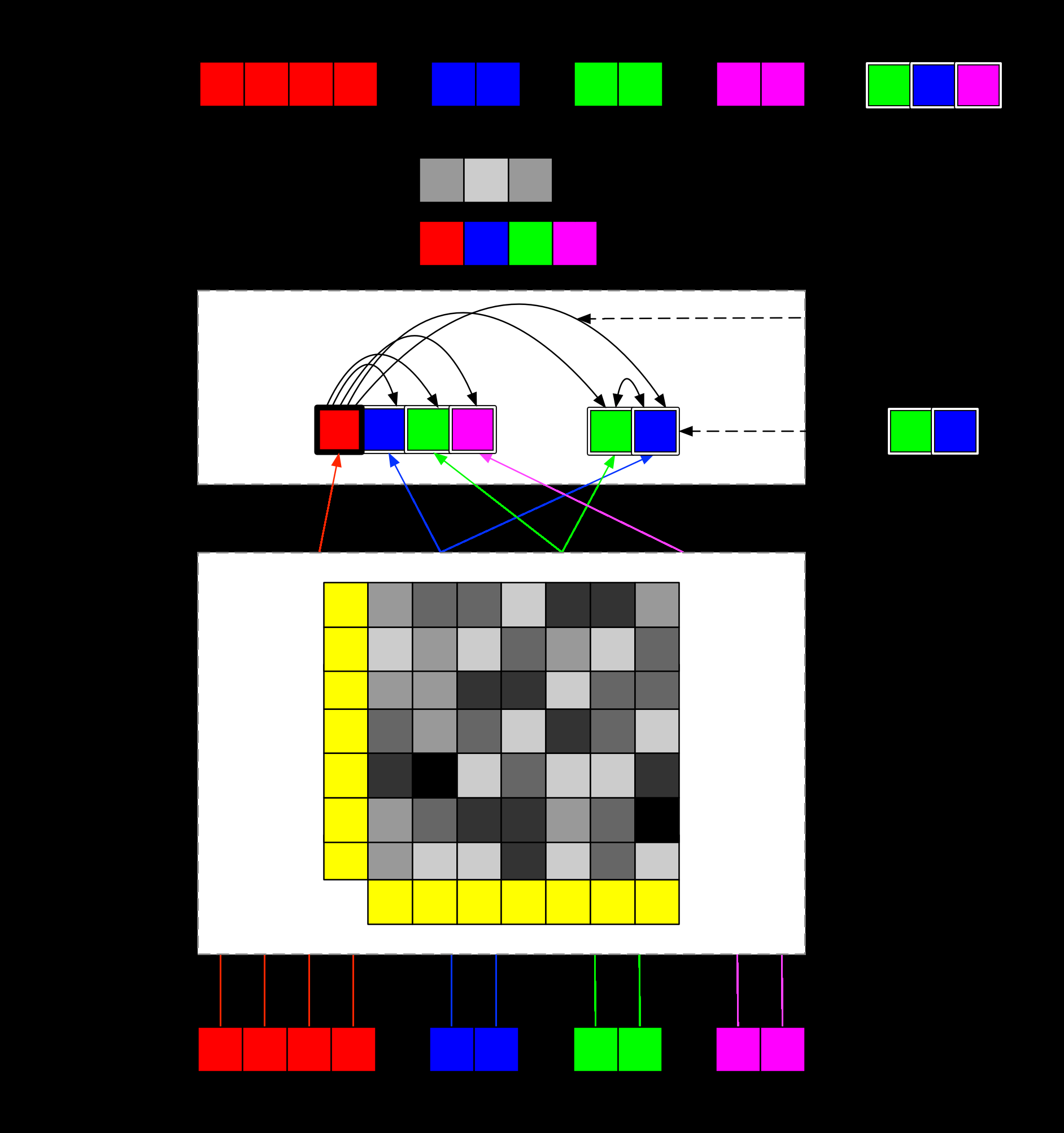
PathQG: Neural Question Generation from Facts
Siyuan Wang, Zhongyu Wei, Zhihao Fan, Zengfeng Huang, Weijian Sun, Qi Zhang, Xuanjing Huang,
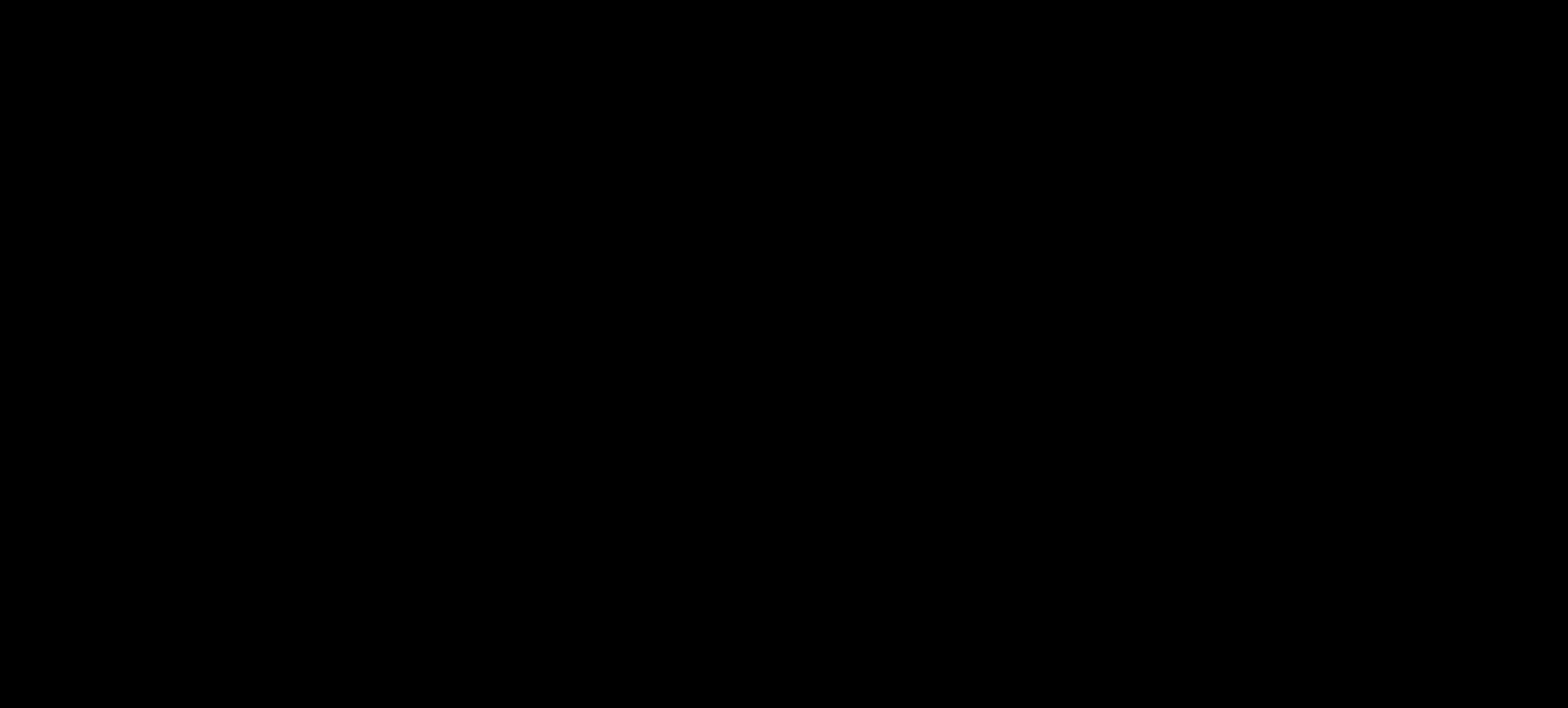