Severing the Edge Between Before and After: Neural Architectures for Temporal Ordering of Events
Miguel Ballesteros, Rishita Anubhai, Shuai Wang, Nima Pourdamghani, Yogarshi Vyas, Jie Ma, Parminder Bhatia, Kathleen McKeown, Yaser Al-Onaizan
Information Extraction Short Paper
You can open the pre-recorded video in a separate window.
Abstract:
In this paper, we propose a neural architecture and a set of training methods for ordering events by predicting temporal relations. Our proposed models receive a pair of events within a span of text as input and they identify temporal relations (Before, After, Equal, Vague) between them. Given that a key challenge with this task is the scarcity of annotated data, our models rely on either pretrained representations (i.e. RoBERTa, BERT or ELMo), transfer and multi-task learning (by leveraging complementary datasets), and self-training techniques. Experiments on the MATRES dataset of English documents establish a new state-of-the-art on this task.
NOTE: Video may display a random order of authors.
Correct author list is at the top of this page.
Connected Papers in EMNLP2020
Similar Papers
Systematic Comparison of Neural Architectures and Training Approaches for Open Information Extraction
Patrick Hohenecker, Frank Mtumbuka, Vid Kocijan, Thomas Lukasiewicz,

Incremental Neural Coreference Resolution in Constant Memory
Patrick Xia, João Sedoc, Benjamin Van Durme,

Joint Constrained Learning for Event-Event Relation Extraction
Haoyu Wang, Muhao Chen, Hongming Zhang, Dan Roth,

Learning from Context or Names? An Empirical Study on Neural Relation Extraction
Hao Peng, Tianyu Gao, Xu Han, Yankai Lin, Peng Li, Zhiyuan Liu, Maosong Sun, Jie Zhou,
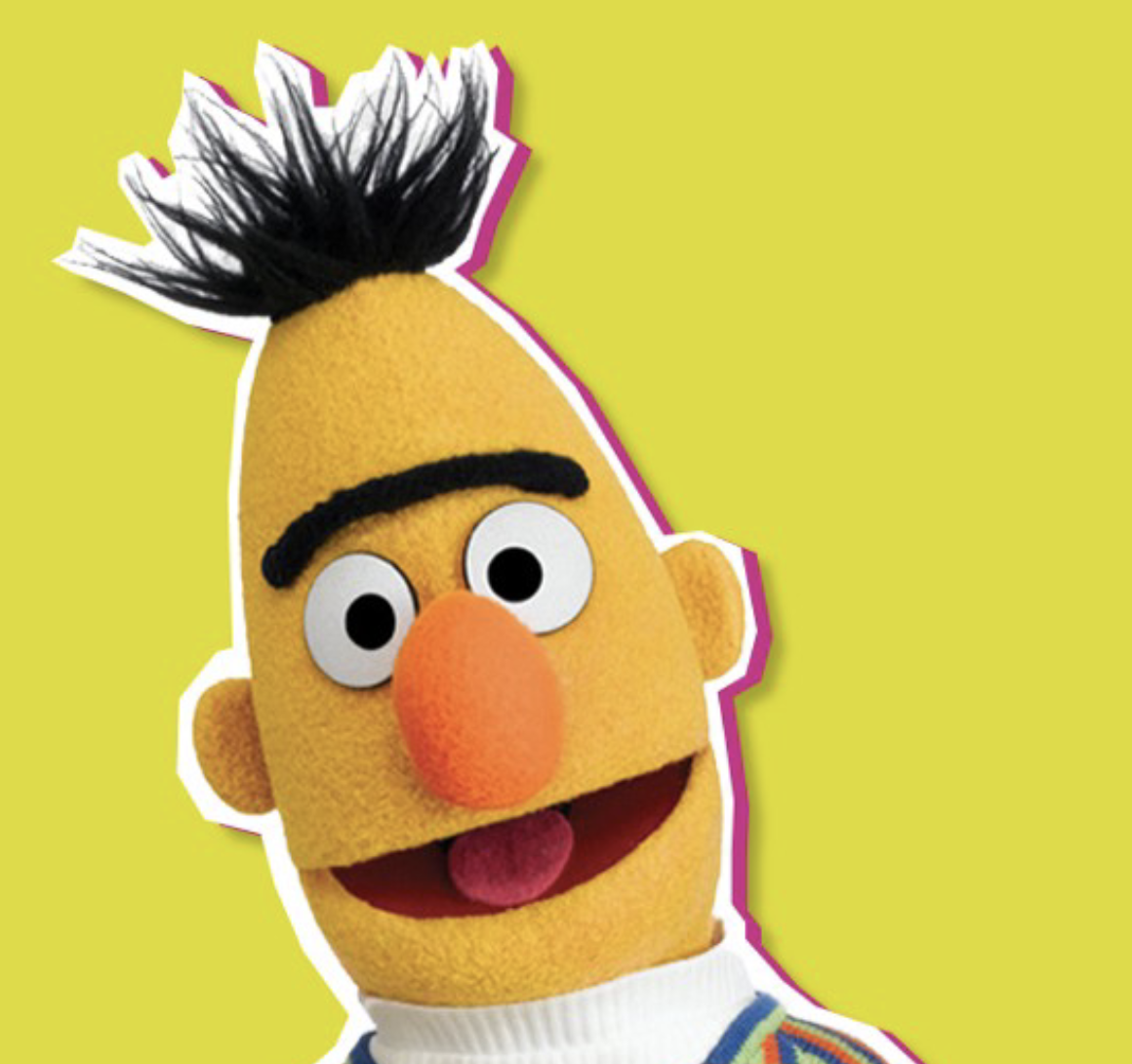