Task-Completion Dialogue Policy Learning via Monte Carlo Tree Search with Dueling Network
Sihan Wang, Kaijie Zhou, Kunfeng Lai, Jianping Shen
Dialog and Interactive Systems Long Paper
You can open the pre-recorded video in a separate window.
Abstract:
We introduce a framework of Monte Carlo Tree Search with Double-q Dueling network (MCTS-DDU) for task-completion dialogue policy learning. Different from the previous deep model-based reinforcement learning methods, which uses background planning and may suffer from low-quality simulated experiences, MCTS-DDU performs decision-time planning based on dialogue state search trees built by Monte Carlo simulations and is robust to the simulation errors. Such idea arises naturally in human behaviors, e.g. predicting others' responses and then deciding our own actions. In the simulated movie-ticket booking task, our method outperforms the background planning approaches significantly. We demonstrate the effectiveness of MCTS and the dueling network in detailed ablation studies, and also compare the performance upper bounds of these two planning methods.
NOTE: Video may display a random order of authors.
Correct author list is at the top of this page.
Connected Papers in EMNLP2020
Similar Papers
MinTL: Minimalist Transfer Learning for Task-Oriented Dialogue Systems
Zhaojiang Lin, Andrea Madotto, Genta Indra Winata, Pascale Fung,
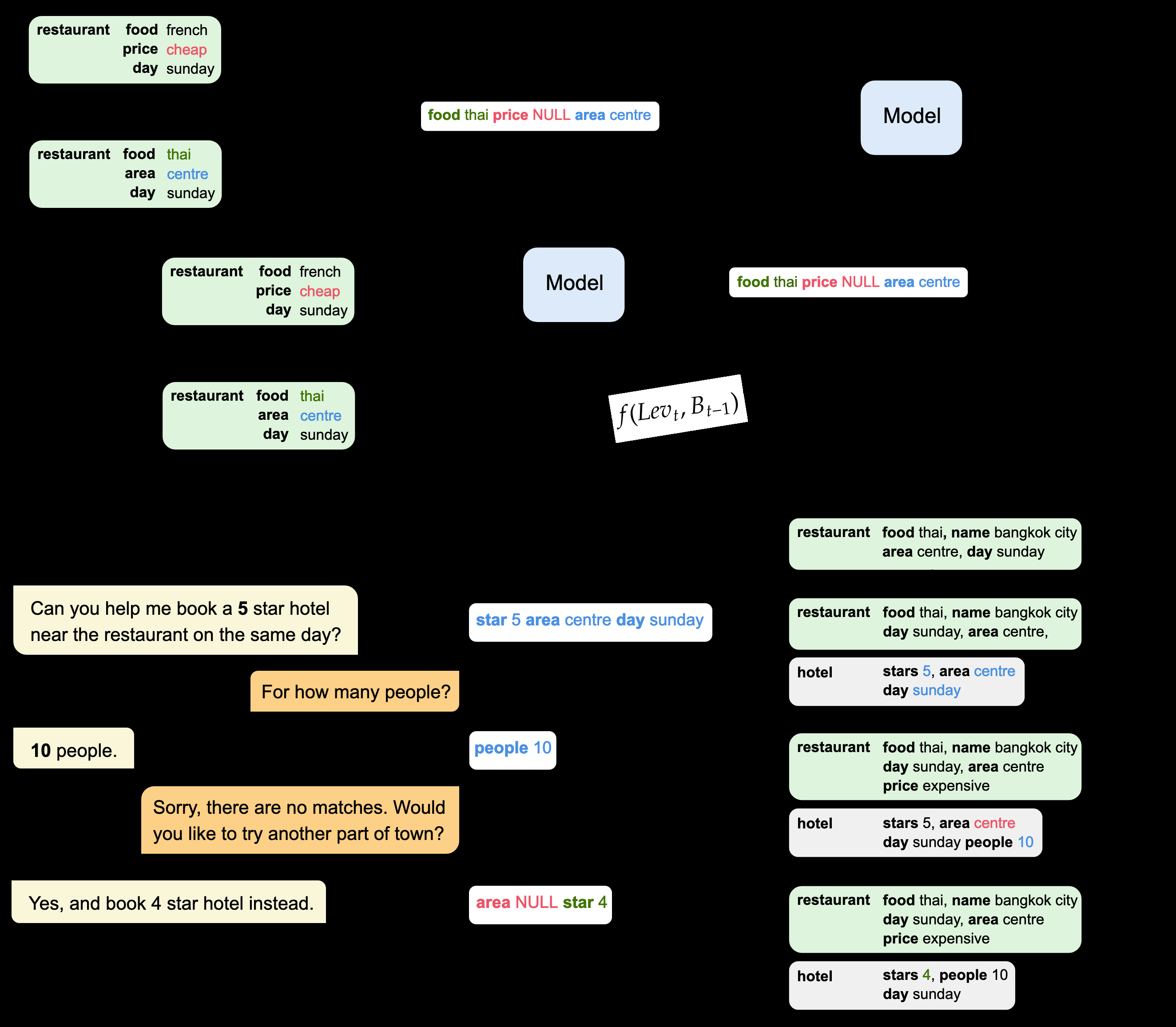
Task-Oriented Dialogue as Dataflow Synthesis
Jacob Andreas, John Bufe, David Burkett, Charles Chen, Josh Clausman, Jean Crawford, Kate Crim, Jordan DeLoach, Leah Dorner, Jason Eisner, Hao Fang, Alan Guo, David Hall, Kristin Hayes, Kellie Hill, Diana Ho, Wendy Iwaszuk, Smriti Jha, Dan Klein, Jayant Krishnamurthy, Theo Lanman, Percy Liang, Christopher Lin, Ilya Lintsbakh, Andy McGovern, Alexander Nisnevich, Adam Pauls, Brent Read, Dan Roth, Subhro Roy, Beth Short, Div Slomin, Ben Snyder, Stephon Striplin, Yu Su, Zachary Tellman, Sam Thomson, Andrei Vorobev, Izabela Witoszko, Jason Wolfe, Abby Wray, Yuchen Zhang, Alexander Zotov, Jesse Rusak, Dmitrij Petters,

Multi-turn Response Selection using Dialogue Dependency Relations
Qi Jia, Yizhu Liu, Siyu Ren, Kenny Zhu, Haifeng Tang,

Learning a Simple and Effective Model for Multi-turn Response Generation with Auxiliary Tasks
Yufan Zhao, Can Xu, Wei Wu,
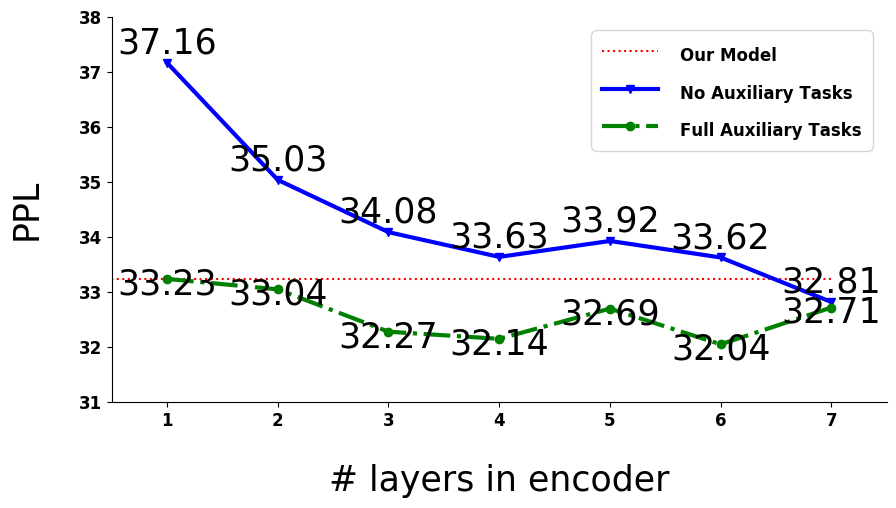