Attention is Not Only a Weight: Analyzing Transformers with Vector Norms
Goro Kobayashi, Tatsuki Kuribayashi, Sho Yokoi, Kentaro Inui
Interpretability and Analysis of Models for NLP Long Paper
You can open the pre-recorded video in a separate window.
Abstract:
Attention is a key component of Transformers, which have recently achieved considerable success in natural language processing. Hence, attention is being extensively studied to investigate various linguistic capabilities of Transformers, focusing on analyzing the parallels between attention weights and specific linguistic phenomena. This paper shows that attention weights alone are only one of the two factors that determine the output of attention and proposes a norm-based analysis that incorporates the second factor, the norm of the transformed input vectors. The findings of our norm-based analyses of BERT and a Transformer-based neural machine translation system include the following: (i) contrary to previous studies, BERT pays poor attention to special tokens, and (ii) reasonable word alignment can be extracted from attention mechanisms of Transformer. These findings provide insights into the inner workings of Transformers.
NOTE: Video may display a random order of authors.
Correct author list is at the top of this page.
Connected Papers in EMNLP2020
Similar Papers
On the Ability and Limitations of Transformers to Recognize Formal Languages
Satwik Bhattamishra, Kabir Ahuja, Navin Goyal,

Transformers: State-of-the-Art Natural Language Processing
Thomas Wolf, Julien Chaumond, Lysandre Debut, Victor Sanh, Clement Delangue, Anthony Moi, Pierric Cistac, Morgan Funtowicz, Joe Davison, Sam Shleifer, Remi Louf, Patrick von Platen, Tim Rault, Yacine Jernite, Teven Le Scao, Sylvain Gugger, Julien Plu, Clara Ma, Canwei Shen, Mariama Drame, Quentin Lhoest, Alexander Rush,
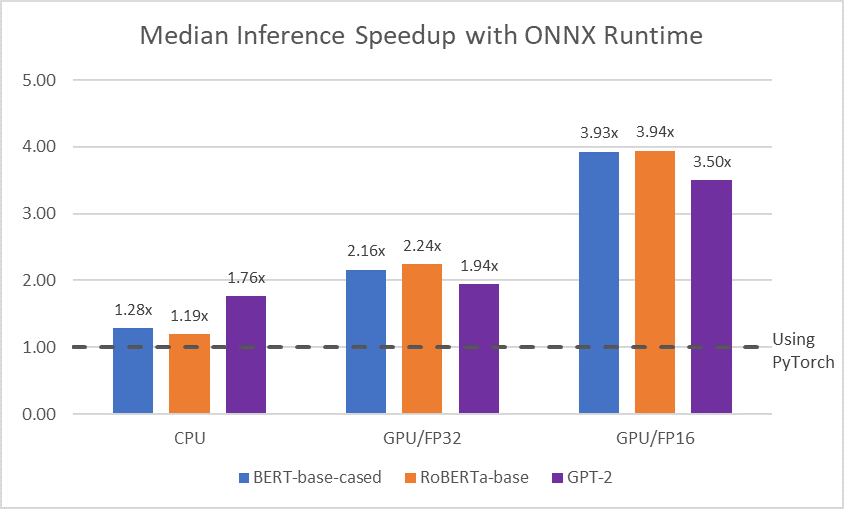
Losing Heads in the Lottery: Pruning Transformer Attention in Neural Machine Translation
Maximiliana Behnke, Kenneth Heafield,
