Multi-Unit Transformers for Neural Machine Translation
Jianhao Yan, Fandong Meng, Jie Zhou
Machine Translation and Multilinguality Long Paper
You can open the pre-recorded video in a separate window.
Abstract:
Transformer models achieve remarkable success in Neural Machine Translation. Many efforts have been devoted to deepening the Transformer by stacking several units (i.e., a combination of Multihead Attentions and FFN) in a cascade, while the investigation over multiple parallel units draws little attention. In this paper, we propose the Multi-Unit Transformer (MUTE) , which aim to promote the expressiveness of the Transformer by introducing diverse and complementary units. Specifically, we use several parallel units and show that modeling with multiple units improves model performance and introduces diversity. Further, to better leverage the advantage of the multi-unit setting, we design biased module and sequential dependency that guide and encourage complementariness among different units. Experimental results on three machine translation tasks, the NIST Chinese-to-English, WMT'14 English-to-German and WMT'18 Chinese-to-English, show that the MUTE models significantly outperform the Transformer-Base, by up to +1.52, +1.90 and +1.10 BLEU points, with only a mild drop in inference speed (about 3.1\%). In addition, our methods also surpass the Transformer-Big model, with only 54\% of its parameters. These results demonstrate the effectiveness of the MUTE, as well as its efficiency in both the inference process and parameter usage.
NOTE: Video may display a random order of authors.
Correct author list is at the top of this page.
Connected Papers in EMNLP2020
Similar Papers
Shallow-to-Deep Training for Neural Machine Translation
Bei Li, Ziyang Wang, Hui Liu, Yufan Jiang, Quan Du, Tong Xiao, Huizhen Wang, Jingbo Zhu,

Long-Short Term Masking Transformer: A Simple but Effective Baseline for Document-level Neural Machine Translation
Pei Zhang, Boxing Chen, Niyu Ge, Kai Fan,

Towards Enhancing Faithfulness for Neural Machine Translation
Rongxiang Weng, Heng Yu, Xiangpeng Wei, Weihua Luo,
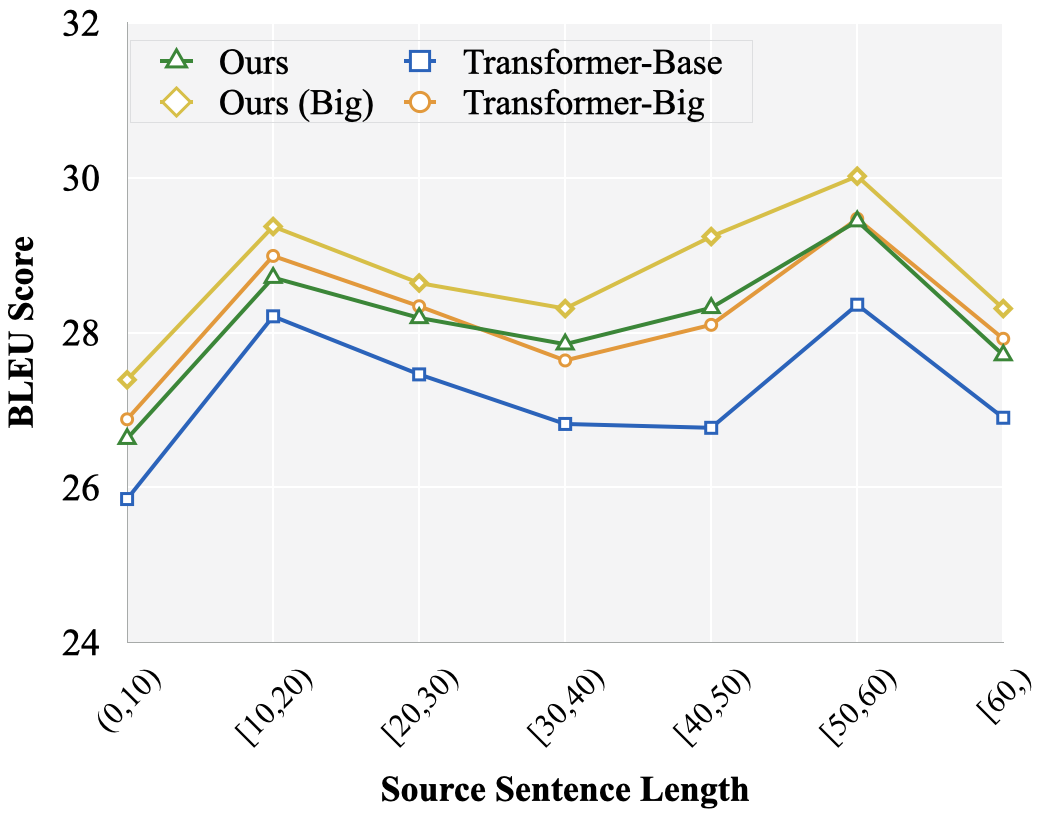
ETC: Encoding Long and Structured Inputs in Transformers
Joshua Ainslie, Santiago Ontanon, Chris Alberti, Vaclav Cvicek, Zachary Fisher, Philip Pham, Anirudh Ravula, Sumit Sanghai, Qifan Wang, Li Yang,
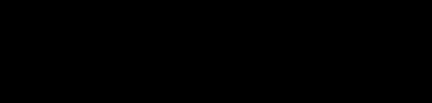