On the Ability and Limitations of Transformers to Recognize Formal Languages
Satwik Bhattamishra, Kabir Ahuja, Navin Goyal
Interpretability and Analysis of Models for NLP Long Paper
You can open the pre-recorded video in a separate window.
Abstract:
Transformers have supplanted recurrent models in a large number of NLP tasks. However, the differences in their abilities to model different syntactic properties remain largely unknown. Past works suggest that LSTMs generalize very well on regular languages and have close connections with counter languages. In this work, we systematically study the ability of Transformers to model such languages as well as the role of its individual components in doing so. We first provide a construction of Transformers for a subclass of counter languages, including well-studied languages such as n-ary Boolean Expressions, Dyck-1, and its generalizations. In experiments, we find that Transformers do well on this subclass, and their learned mechanism strongly correlates with our construction. Perhaps surprisingly, in contrast to LSTMs, Transformers do well only on a subset of regular languages with degrading performance as we make languages more complex according to a well-known measure of complexity. Our analysis also provides insights on the role of self-attention mechanism in modeling certain behaviors and the influence of positional encoding schemes on the learning and generalization abilities of the model.
NOTE: Video may display a random order of authors.
Correct author list is at the top of this page.
Connected Papers in EMNLP2020
Similar Papers
Analyzing Individual Neurons in Pre-trained Language Models
Nadir Durrani, Hassan Sajjad, Fahim Dalvi, Yonatan Belinkov,
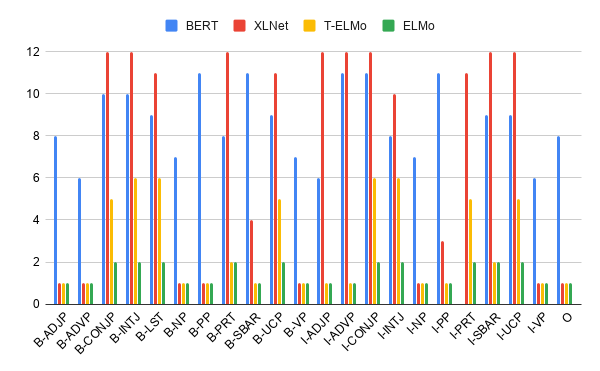
Grounded Compositional Outputs for Adaptive Language Modeling
Nikolaos Pappas, Phoebe Mulcaire, Noah A. Smith,
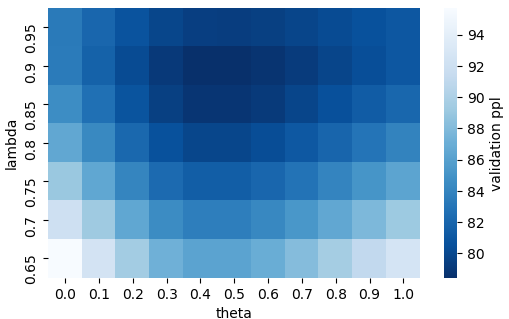
COGS: A Compositional Generalization Challenge Based on Semantic Interpretation
Najoung Kim, Tal Linzen,
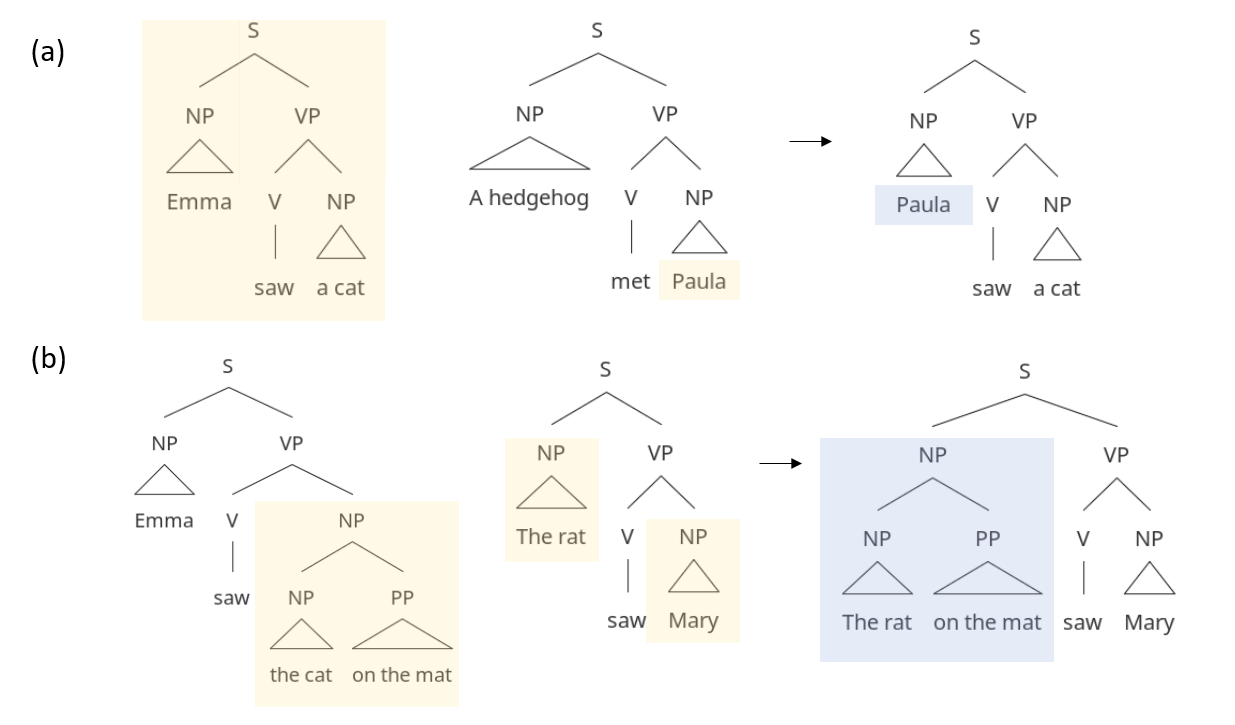
Syntactic Structure Distillation Pretraining for Bidirectional Encoders
Adhiguna Kuncoro, Lingpeng Kong, Daniel Fried, Dani Yogatama, Laura Rimell, Chris Dyer, Phil Blunsom,
