Competence-Level Prediction and Resume & Job Description Matching Using Context-Aware Transformer Models
Changmao Li, Elaine Fisher, Rebecca Thomas, Steve Pittard, Vicki Hertzberg, Jinho D. Choi
NLP Applications Long Paper
You can open the pre-recorded video in a separate window.
Abstract:
This paper presents a comprehensive study on resume classification to reduce the time and labor needed to screen an overwhelming number of applications significantly, while improving the selection of suitable candidates. A total of 6,492 resumes are extracted from 24,933 job applications for 252 positions designated into four levels of experience for Clinical Research Coordinators (CRC). Each resume is manually annotated to its most appropriate CRC position by experts through several rounds of triple annotation to establish guidelines. As a result, a high Kappa score of 61% is achieved for inter-annotator agreement. Given this dataset, novel transformer-based classification models are developed for two tasks: the first task takes a resume and classifies it to a CRC level (T1), and the second task takes both a resume and a job description to apply and predicts if the application is suited to the job (T2). Our best models using section encoding and a multi-head attention decoding give results of 73.3% to T1 and 79.2% to T2. Our analysis shows that the prediction errors are mostly made among adjacent CRC levels, which are hard for even experts to distinguish, implying the practical value of our models in real HR platforms.
NOTE: Video may display a random order of authors.
Correct author list is at the top of this page.
Connected Papers in EMNLP2020
Similar Papers
Program Enhanced Fact Verification with Verbalization and Graph Attention Network
Xiaoyu Yang, Feng Nie, Yufei Feng, Quan Liu, Zhigang Chen, Xiaodan Zhu,

Slot Attention with Value Normalization for Multi-Domain Dialogue State Tracking
Yexiang Wang, Yi Guo, Siqi Zhu,

Temporal Knowledge Base Completion: New Algorithms and Evaluation Protocols
Prachi Jain, Sushant Rathi, Mausam, Soumen Chakrabarti,
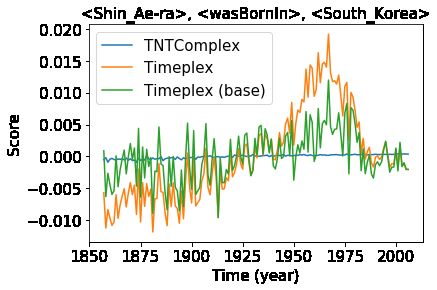
XL-WiC: A Multilingual Benchmark for Evaluating Semantic Contextualization
Alessandro Raganato, Tommaso Pasini, Jose Camacho-Collados, Mohammad Taher Pilehvar,
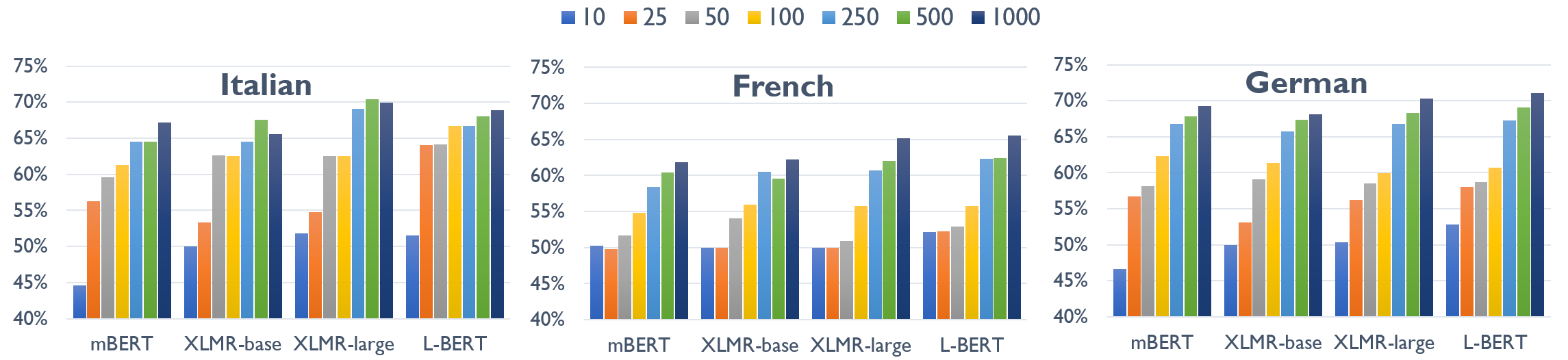