Detecting Attackable Sentences in Arguments
Yohan Jo, Seojin Bang, Emaad Manzoor, Eduard Hovy, Chris Reed
Sentiment Analysis, Stylistic Analysis, and Argument Mining Long Paper
You can open the pre-recorded video in a separate window.
Abstract:
Finding attackable sentences in an argument is the first step toward successful refutation in argumentation. We present a first large-scale analysis of sentence attackability in online arguments. We analyze driving reasons for attacks in argumentation and identify relevant characteristics of sentences. We demonstrate that a sentence's attackability is associated with many of these characteristics regarding the sentence's content, proposition types, and tone, and that an external knowledge source can provide useful information about attackability. Building on these findings, we demonstrate that machine learning models can automatically detect attackable sentences in arguments, significantly better than several baselines and comparably well to laypeople.
NOTE: Video may display a random order of authors.
Correct author list is at the top of this page.
Connected Papers in EMNLP2020
Similar Papers
An Analysis of Natural Language Inference Benchmarks through the Lens of Negation
Md Mosharaf Hossain, Venelin Kovatchev, Pranoy Dutta, Tiffany Kao, Elizabeth Wei, Eduardo Blanco,

Neural Conversational QA: Learning to Reason vs Exploiting Patterns
Nikhil Verma, Abhishek Sharma, Dhiraj Madan, Danish Contractor, Harshit Kumar, Sachindra Joshi,
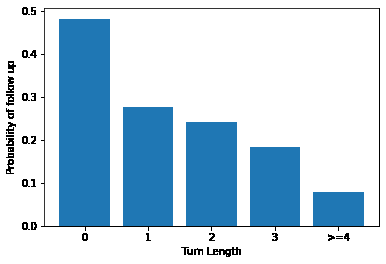
Adversarial Attack and Defense of Structured Prediction Models
Wenjuan Han, Liwen Zhang, Yong Jiang, Kewei Tu,
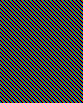