Re-evaluating Evaluation in Text Summarization
Manik Bhandari, Pranav Narayan Gour, Atabak Ashfaq, Pengfei Liu, Graham Neubig
Summarization Long Paper
You can open the pre-recorded video in a separate window.
Abstract:
Automated evaluation metrics as a stand-in for manual evaluation are an essential part of the development of text-generation tasks such as text summarization. However, while the field has progressed, our standard metrics have not -- for nearly 20 years ROUGE has been the standard evaluation in most summarization papers. In this paper, we make an attempt to re-evaluate the evaluation method for text summarization: assessing the reliability of automatic metrics using top-scoring system outputs, both abstractive and extractive, on recently popular datasets for both system-level and summary-level evaluation settings. We find that conclusions about evaluation metrics on older datasets do not necessarily hold on modern datasets and systems. We release a dataset of human judgments that are collected from 25 top-scoring neural summarization systems (14 abstractive and 11 extractive).
NOTE: Video may display a random order of authors.
Correct author list is at the top of this page.
Connected Papers in EMNLP2020
Similar Papers
What Have We Achieved on Text Summarization?
Dandan Huang, Leyang Cui, Sen Yang, Guangsheng Bao, Kun Wang, Jun Xie, Yue Zhang,

Multi-Fact Correction in Abstractive Text Summarization
Yue Dong, Shuohang Wang, Zhe Gan, Yu Cheng, Jackie Chi Kit Cheung, Jingjing Liu,
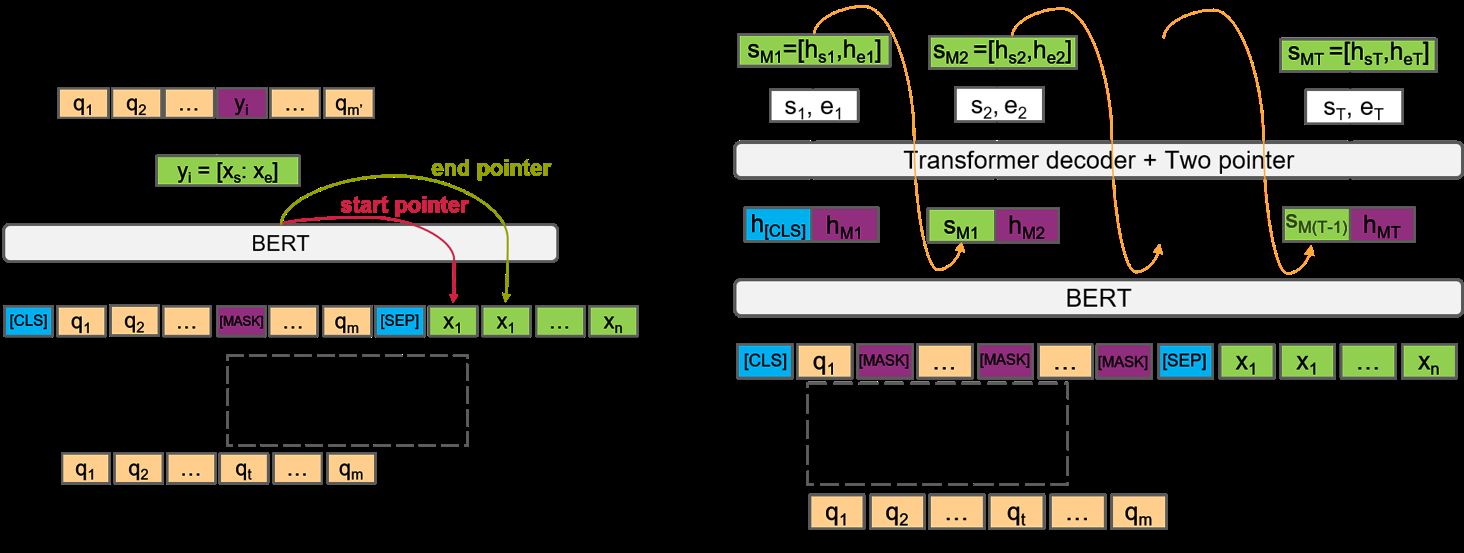
Pre-training for Abstractive Document Summarization by Reinstating Source Text
Yanyan Zou, Xingxing Zhang, Wei Lu, Furu Wei, Ming Zhou,
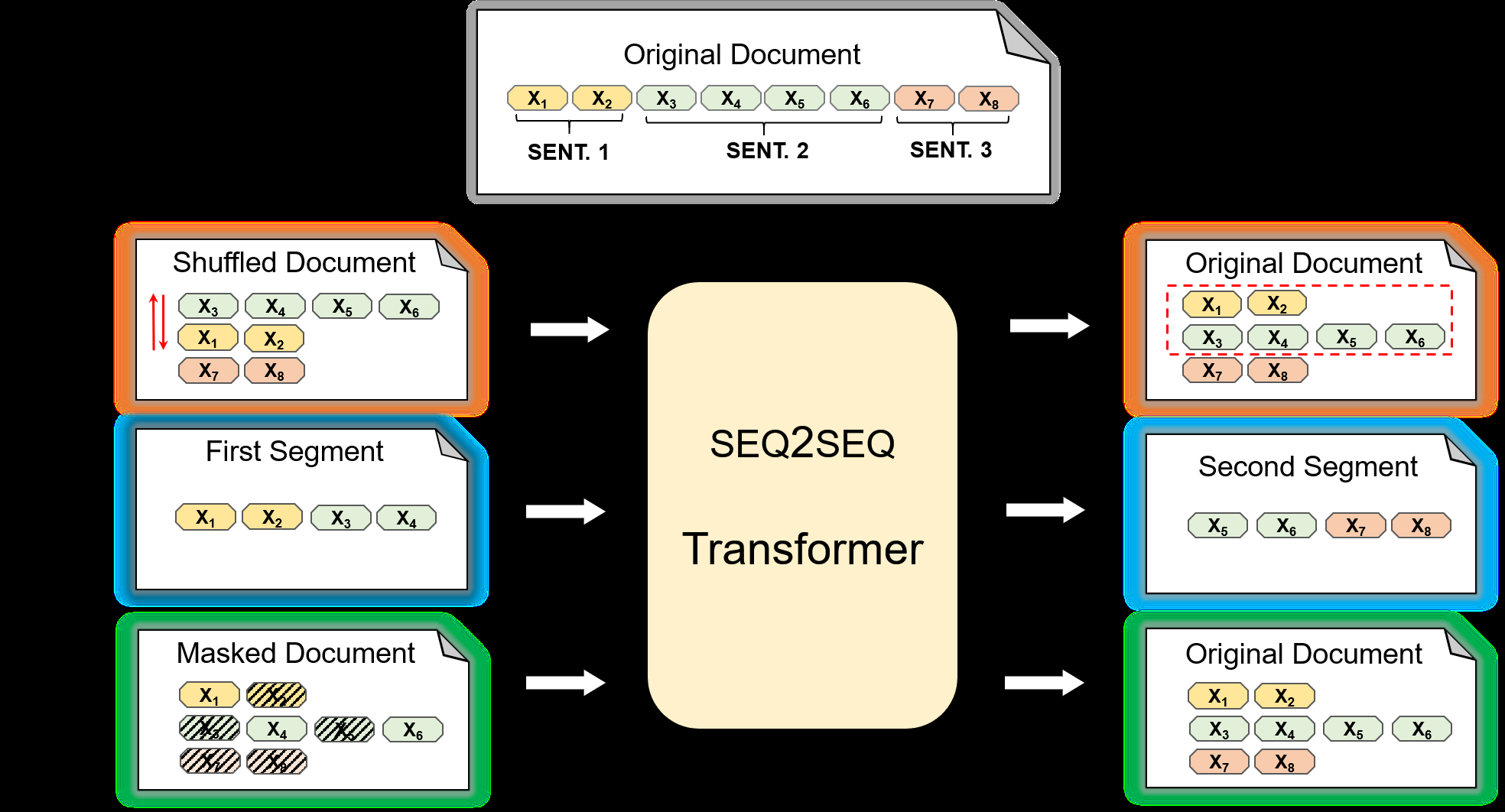