Coupled Hierarchical Transformer for Stance-Aware Rumor Verification in Social Media Conversations
Jianfei Yu, Jing Jiang, Ling Min Serena Khoo, Hai Leong Chieu, Rui Xia
Computational Social Science and Social Media Long Paper
You can open the pre-recorded video in a separate window.
Abstract:
The prevalent use of social media enables rapid spread of rumors on a massive scale, which leads to the emerging need of automatic rumor verification (RV). A number of previous studies focus on leveraging stance classification to enhance RV with multi-task learning (MTL) methods. However, most of these methods failed to employ pre-trained contextualized embeddings such as BERT, and did not exploit inter-task dependencies by using predicted stance labels to improve the RV task. Therefore, in this paper, to extend BERT to obtain thread representations, we first propose a Hierarchical Transformer, which divides each long thread into shorter subthreads, and employs BERT to separately represent each subthread, followed by a global Transformer layer to encode all the subthreads. We further propose a Coupled Transformer Module to capture the inter-task interactions and a Post-Level Attention layer to use the predicted stance labels for RV, respectively. Experiments on two benchmark datasets show the superiority of our Coupled Hierarchical Transformer model over existing MTL approaches.
NOTE: Video may display a random order of authors.
Correct author list is at the top of this page.
Connected Papers in EMNLP2020
Similar Papers
Syntactic Structure Distillation Pretraining for Bidirectional Encoders
Adhiguna Kuncoro, Lingpeng Kong, Daniel Fried, Dani Yogatama, Laura Rimell, Chris Dyer, Phil Blunsom,

Supertagging Combinatory Categorial Grammar with Attentive Graph Convolutional Networks
Yuanhe Tian, Yan Song, Fei Xia,
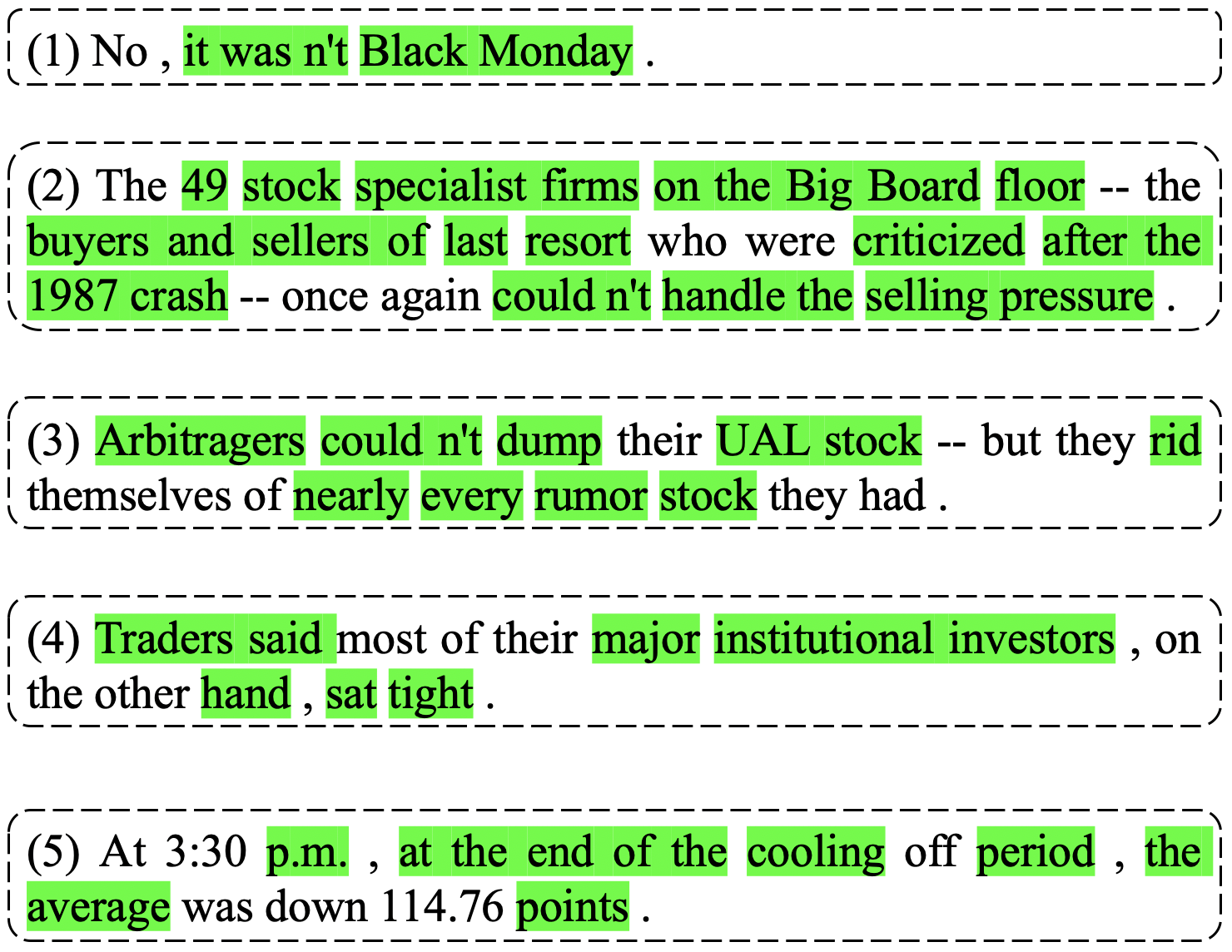
Recall and Learn: Fine-tuning Deep Pretrained Language Models with Less Forgetting
Sanyuan Chen, Yutai Hou, Yiming Cui, Wanxiang Che, Ting Liu, Xiangzhan Yu,
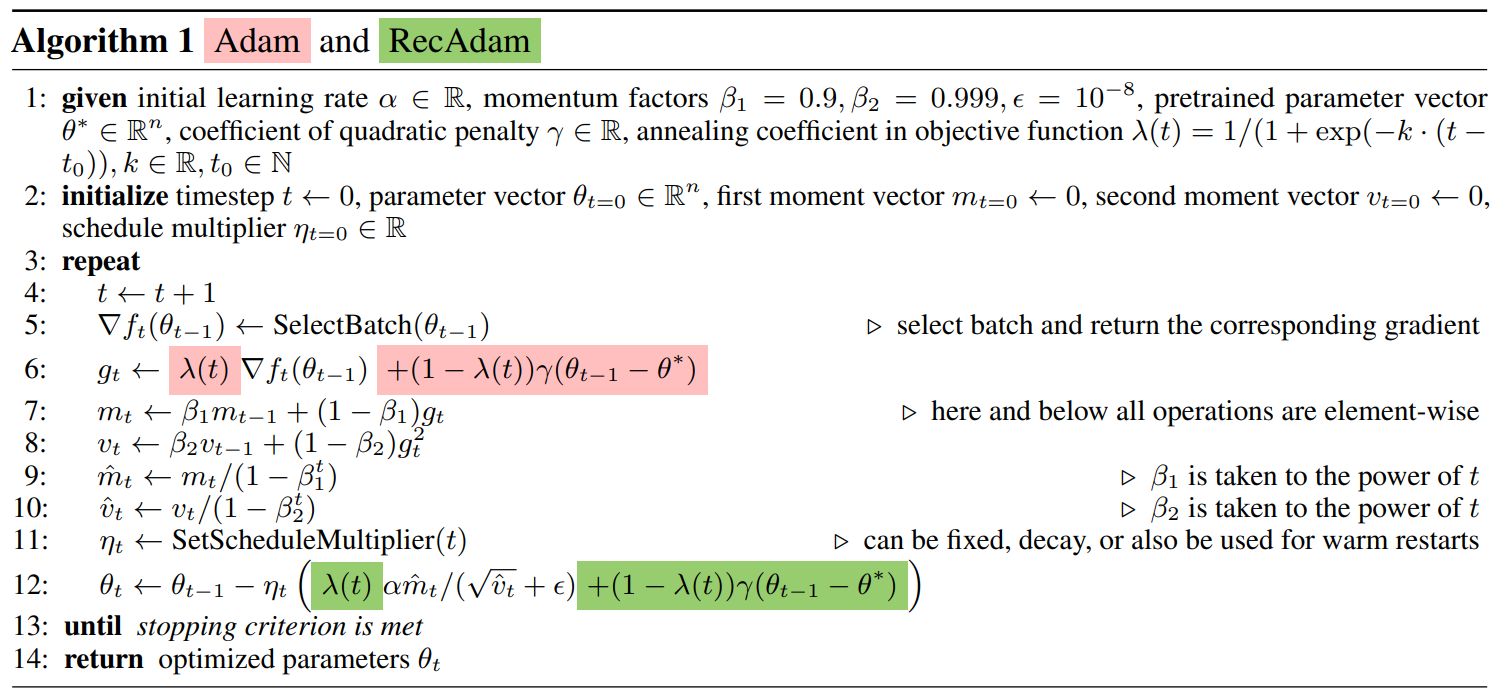