Wasserstein Distance Regularized Sequence Representation for Text Matching in Asymmetrical Domains
Weijie Yu, Chen Xu, Jun Xu, Liang Pang, Xiaopeng Gao, Xiaozhao Wang, Ji-Rong Wen
NLP Applications Long Paper
You can open the pre-recorded video in a separate window.
Abstract:
One approach to matching texts from asymmetrical domains is projecting the input sequences into a common semantic space as feature vectors upon which the matching function can be readily defined and learned. In real-world matching practices, it is often observed that with the training goes on, the feature vectors projected from different domains tend to be indistinguishable. The phenomenon, however, is often overlooked in existing matching models. As a result, the feature vectors are constructed without any regularization, which inevitably increases the difficulty of learning the downstream matching functions. In this paper, we propose a novel match method tailored for text matching in asymmetrical domains, called WD-Match. In WD-Match, a Wasserstein distance-based regularizer is defined to regularize the features vectors projected from different domains. As a result, the method enforces the feature projection function to generate vectors such that those correspond to different domains cannot be easily discriminated. The training process of WD-Match amounts to a game that minimizes the matching loss regularized by the Wasserstein distance. WD-Match can be used to improve different text matching methods, by using the method as its underlying matching model. Four popular text matching methods have been exploited in the paper. Experimental results based on four publicly available benchmarks showed that WD-Match consistently outperformed the underlying methods and the baselines.
NOTE: Video may display a random order of authors.
Correct author list is at the top of this page.
Connected Papers in EMNLP2020
Similar Papers
DeezyMatch: A Flexible Deep Learning Approach to Fuzzy String Matching
Kasra Hosseini, Federico Nanni, Mariona Coll Ardanuy,
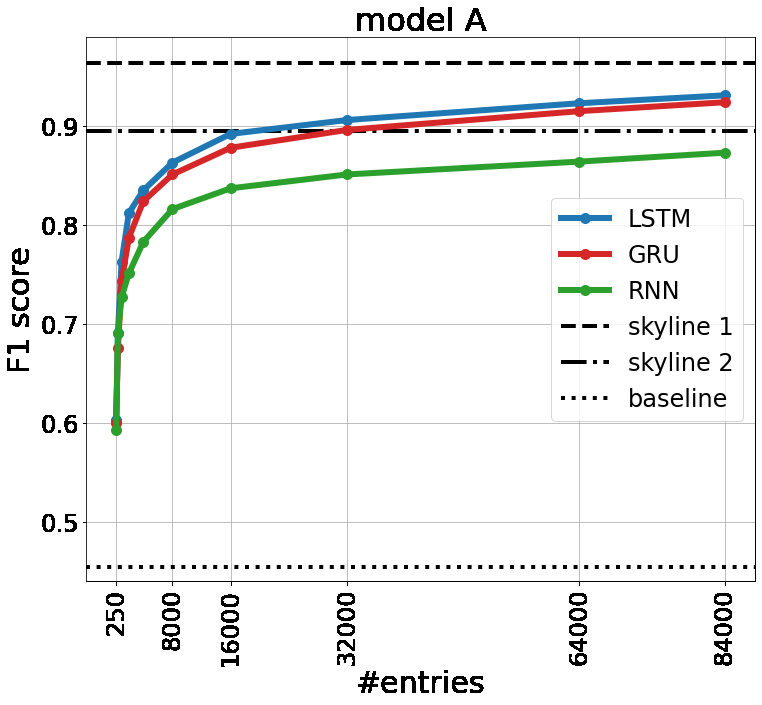
Semantic Label Smoothing for Sequence to Sequence Problems
Michal Lukasik, Himanshu Jain, Aditya Menon, Seungyeon Kim, Srinadh Bhojanapalli, Felix Yu, Sanjiv Kumar,

When Hearst Is not Enough: Improving Hypernymy Detection from Corpus with Distributional Models
Changlong Yu, Jialong Han, Peifeng Wang, Yangqiu Song, Hongming Zhang, Wilfred Ng, Shuming Shi,
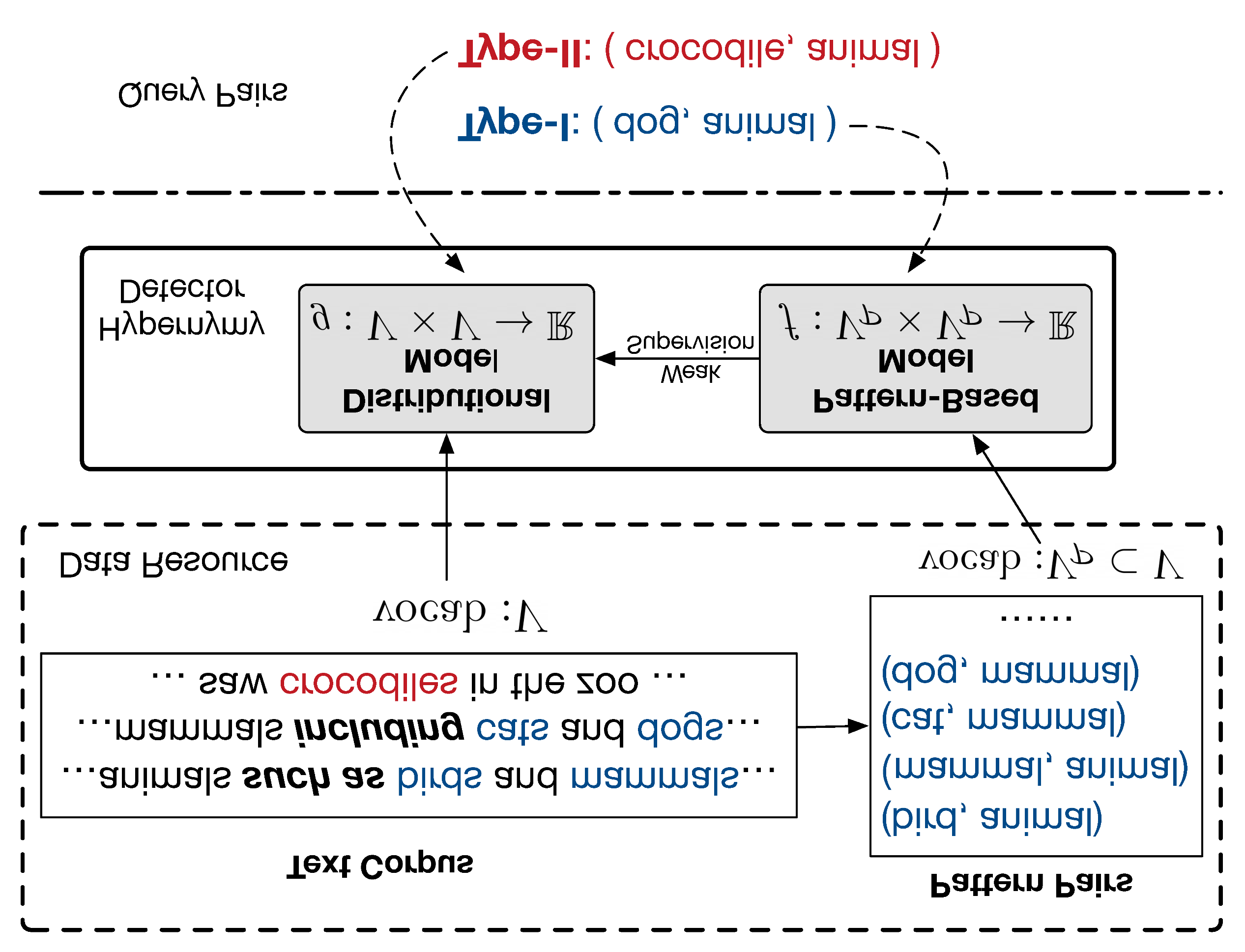
Embedding Words in Non-Vector Space with Unsupervised Graph Learning
Max Ryabinin, Sergei Popov, Liudmila Prokhorenkova, Elena Voita,
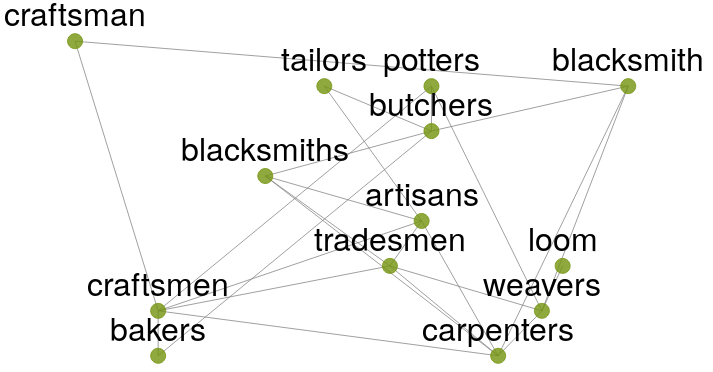