When Hearst Is not Enough: Improving Hypernymy Detection from Corpus with Distributional Models
Changlong Yu, Jialong Han, Peifeng Wang, Yangqiu Song, Hongming Zhang, Wilfred Ng, Shuming Shi
Semantics: Lexical Semantics Long Paper
You can open the pre-recorded video in a separate window.
Abstract:
We address hypernymy detection, i.e., whether an is-a relationship exists between words (x ,y), with the help of large textual corpora. Most conventional approaches to this task have been categorized to be either pattern-based or distributional. Recent studies suggest that pattern-based ones are superior, if large-scale Hearst pairs are extracted and fed, with the sparsity of unseen (x ,y) pairs relieved. However, they become invalid in some specific sparsity cases, where x or y is not involved in any pattern. For the first time, this paper quantifies the non-negligible existence of those specific cases. We also demonstrate that distributional methods are ideal to make up for pattern-based ones in such cases. We devise a complementary framework, under which a pattern-based and a distributional model collaborate seamlessly in cases which they each prefer. On several benchmark datasets, our framework demonstrates improvements that are both competitive and explainable.
NOTE: Video may display a random order of authors.
Correct author list is at the top of this page.
Connected Papers in EMNLP2020
Similar Papers
Small but Mighty: New Benchmarks for Split and Rephrase
Li Zhang, Huaiyu Zhu, Siddhartha Brahma, Yunyao Li,
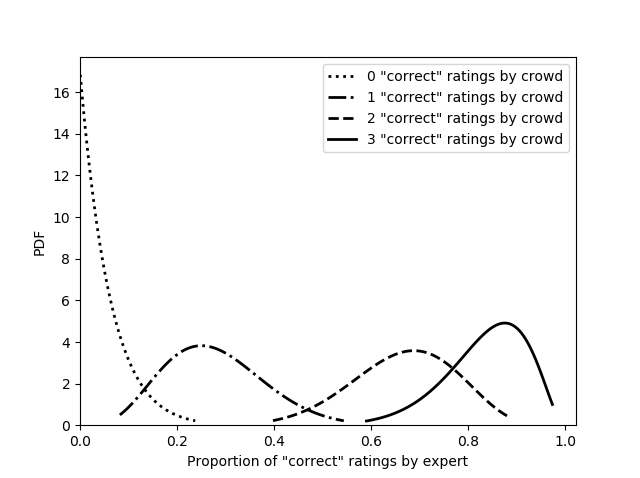
XL-WiC: A Multilingual Benchmark for Evaluating Semantic Contextualization
Alessandro Raganato, Tommaso Pasini, Jose Camacho-Collados, Mohammad Taher Pilehvar,
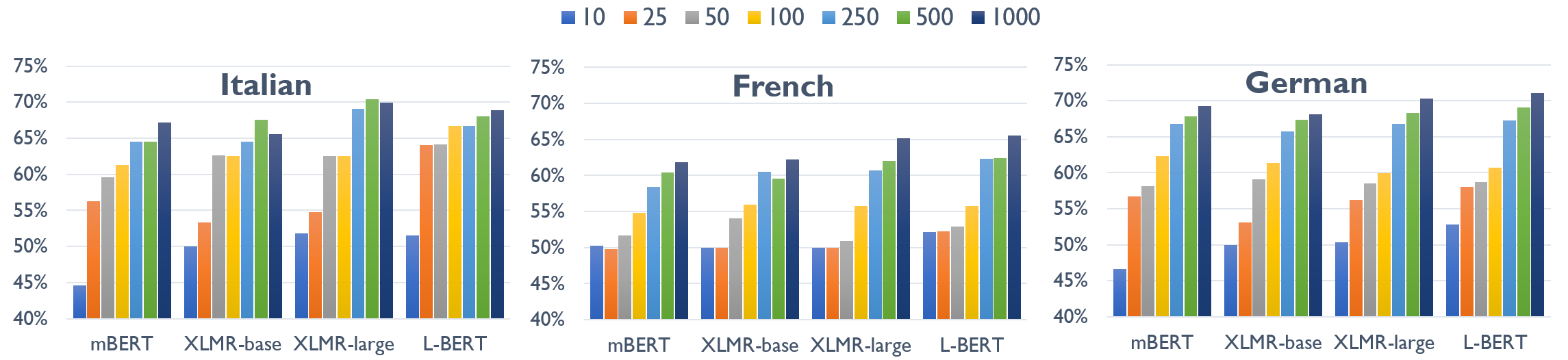
Multilingual Offensive Language Identification with Cross-lingual Embeddings
Tharindu Ranasinghe, Marcos Zampieri,
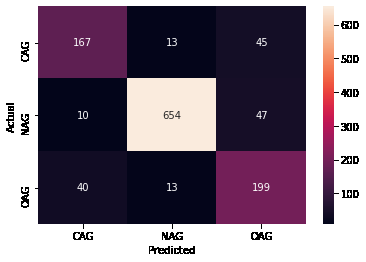