Birds have four legs?! NumerSense: Probing Numerical Commonsense Knowledge of Pre-Trained Language Models
Bill Yuchen Lin, Seyeon Lee, Rahul Khanna, Xiang Ren
Interpretability and Analysis of Models for NLP Short Paper
You can open the pre-recorded video in a separate window.
Abstract:
Recent works show that pre-trained language models (PTLMs), such as BERT, possess certain commonsense and factual knowledge. They suggest that it is promising to use PTLMs as ``neural knowledge bases'' via predicting masked words. Surprisingly, we find that this may not work for numerical commonsense knowledge (e.g., a bird usually has two legs). In this paper, we investigate whether and to what extent we can induce numerical commonsense knowledge from PTLMs as well as the robustness of this process. In this paper, we investigate whether and to what extent we can induce numerical commonsense knowledge from PTLMs as well as the robustness of this process. To study this, we introduce a novel probing task with a diagnostic dataset, NumerSense, containing 13.6k masked-word-prediction probes (10.5k for fine-tuning and 3.1k for testing). Our analysis reveals that: (1) BERT and its stronger variant RoBERTa perform poorly on the diagnostic dataset prior to any fine-tuning; (2) fine-tuning with distant supervision brings some improvement; (3) the best supervised model still performs poorly as compared to human performance (54.06% vs. 96.3% in accuracy).
NOTE: Video may display a random order of authors.
Correct author list is at the top of this page.
Connected Papers in EMNLP2020
Similar Papers
Learning Which Features Matter: RoBERTa Acquires a Preference for Linguistic Generalizations (Eventually)
Alex Warstadt, Yian Zhang, Xiaocheng Li, Haokun Liu, Samuel R. Bowman,
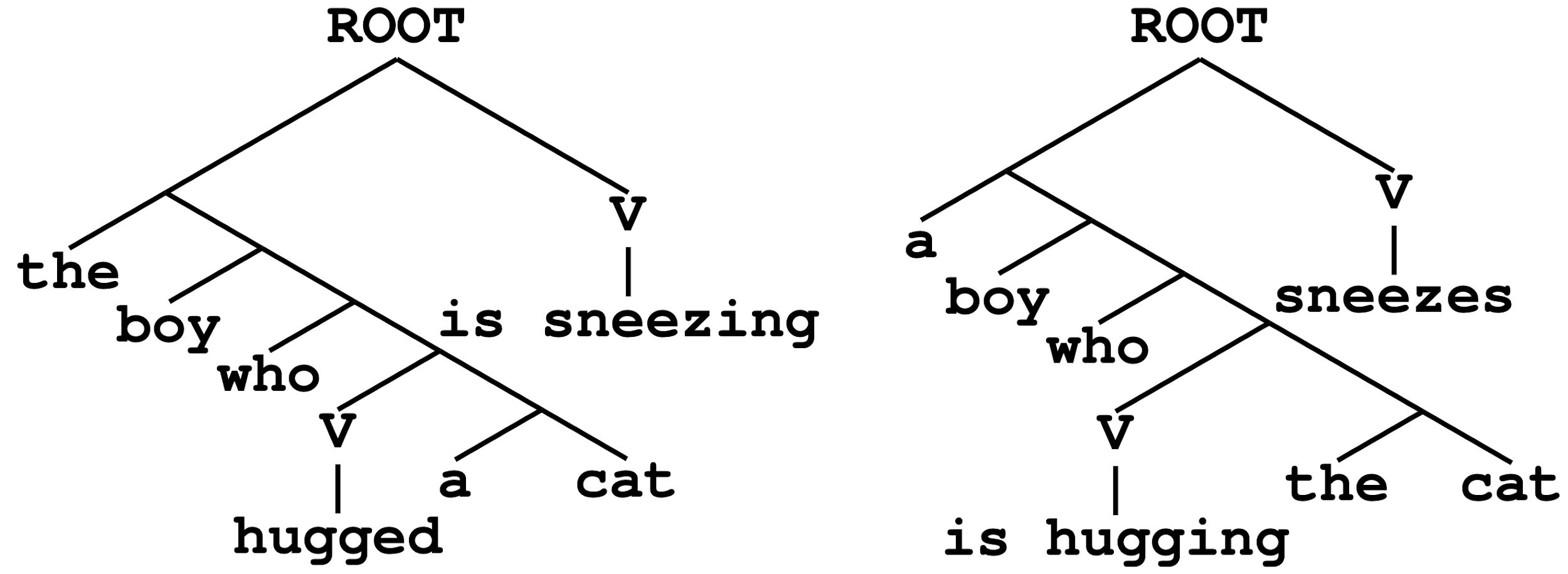
Generationary or “How We Went beyond Word Sense Inventories and Learned to Gloss”
Michele Bevilacqua, Marco Maru, Roberto Navigli,

MUTANT: A Training Paradigm for Out-of-Distribution Generalization in Visual Question Answering
Tejas Gokhale, Pratyay Banerjee, Chitta Baral, Yezhou Yang,
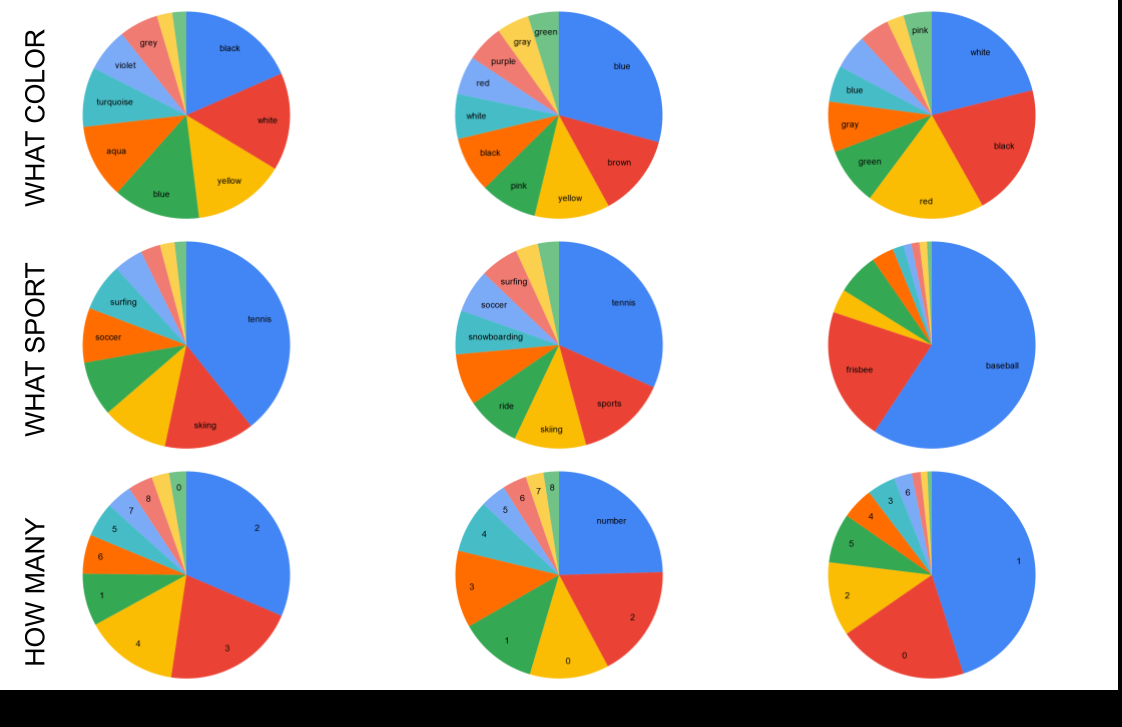
Intrinsic Probing through Dimension Selection
Lucas Torroba Hennigen, Adina Williams, Ryan Cotterell,
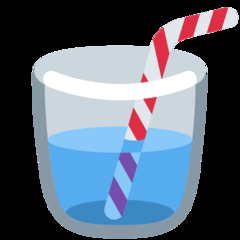