Causal Inference of Script Knowledge
Noah Weber, Rachel Rudinger, Benjamin Van Durme
Semantics: Sentence-level Semantics, Textual Inference and Other areas Long Paper
You can open the pre-recorded video in a separate window.
Abstract:
When does a sequence of events define an everyday scenario and how can this knowledge be induced from text? Prior works in inducing such scripts have relied on, in one form or another, measures of correlation between instances of events in a corpus. We argue from both a conceptual and practical sense that a purely correlation-based approach is insufficient, and instead propose an approach to script induction based on the causal effect between events, formally defined via interventions. Through both human and automatic evaluations, we show that the output of our method based on causal effects better matches the intuition of what a script represents.
NOTE: Video may display a random order of authors.
Correct author list is at the top of this page.
Connected Papers in EMNLP2020
Similar Papers
Reasoning about Goals, Steps, and Temporal Ordering with WikiHow
Li Zhang, Qing Lyu, Chris Callison-Burch,
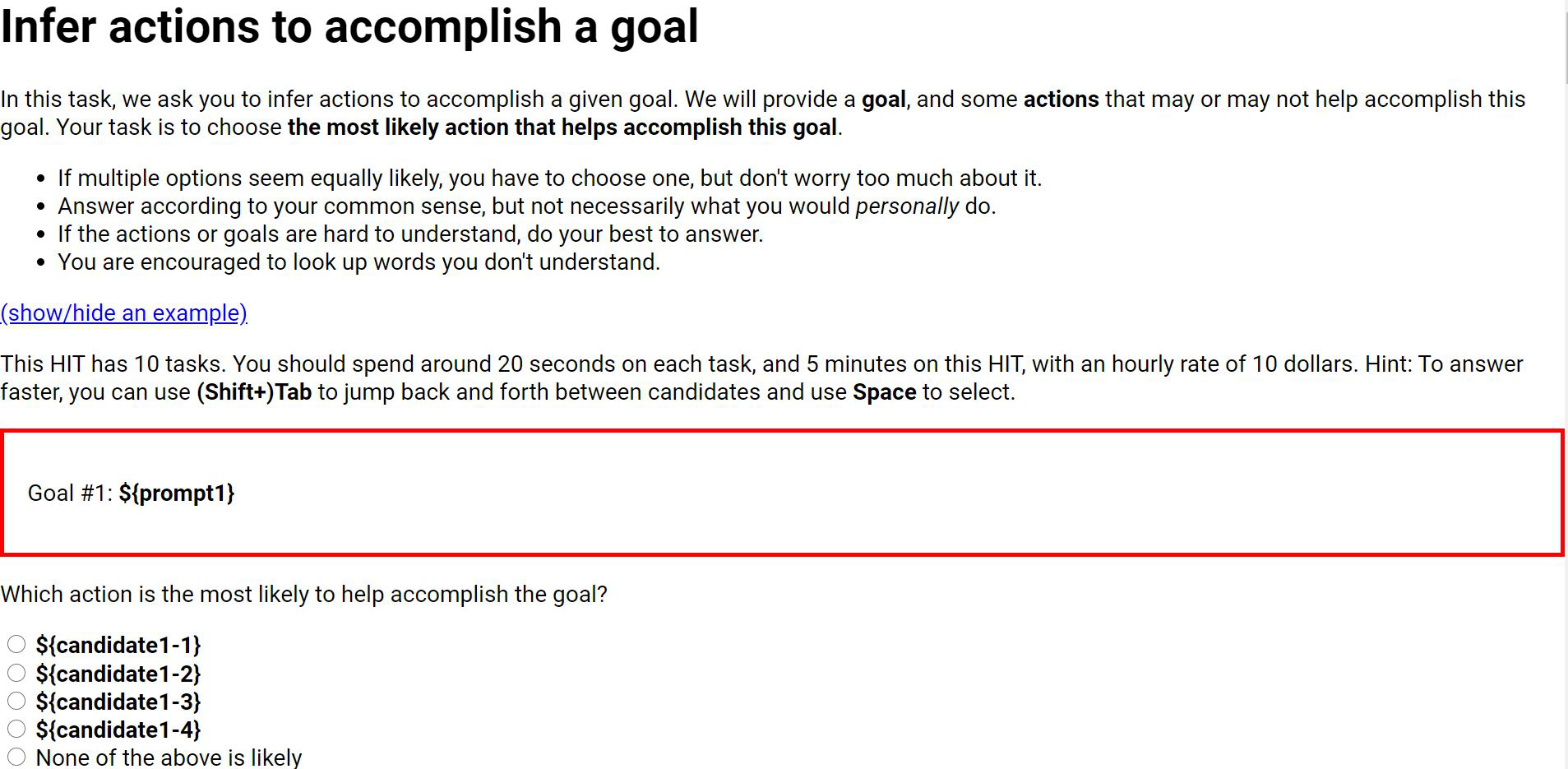
TORQUE: A Reading Comprehension Dataset of Temporal Ordering Questions
Qiang Ning, Hao Wu, Rujun Han, Nanyun Peng, Matt Gardner, Dan Roth,
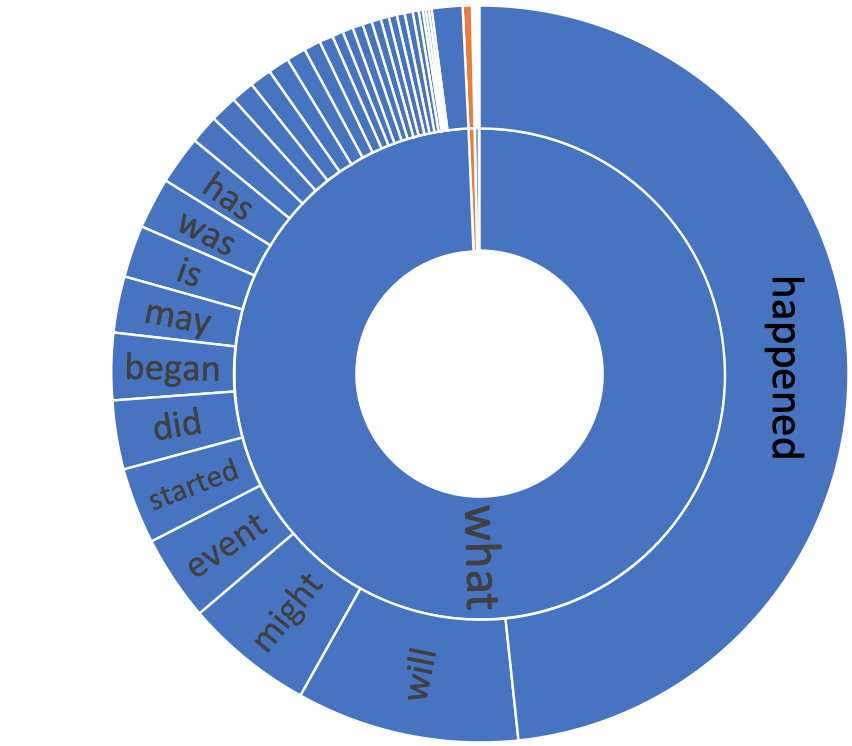
Towards Interpretable Reasoning over Paragraph Effects in Situation
Mucheng Ren, Xiubo Geng, Tao Qin, Heyan Huang, Daxin Jiang,
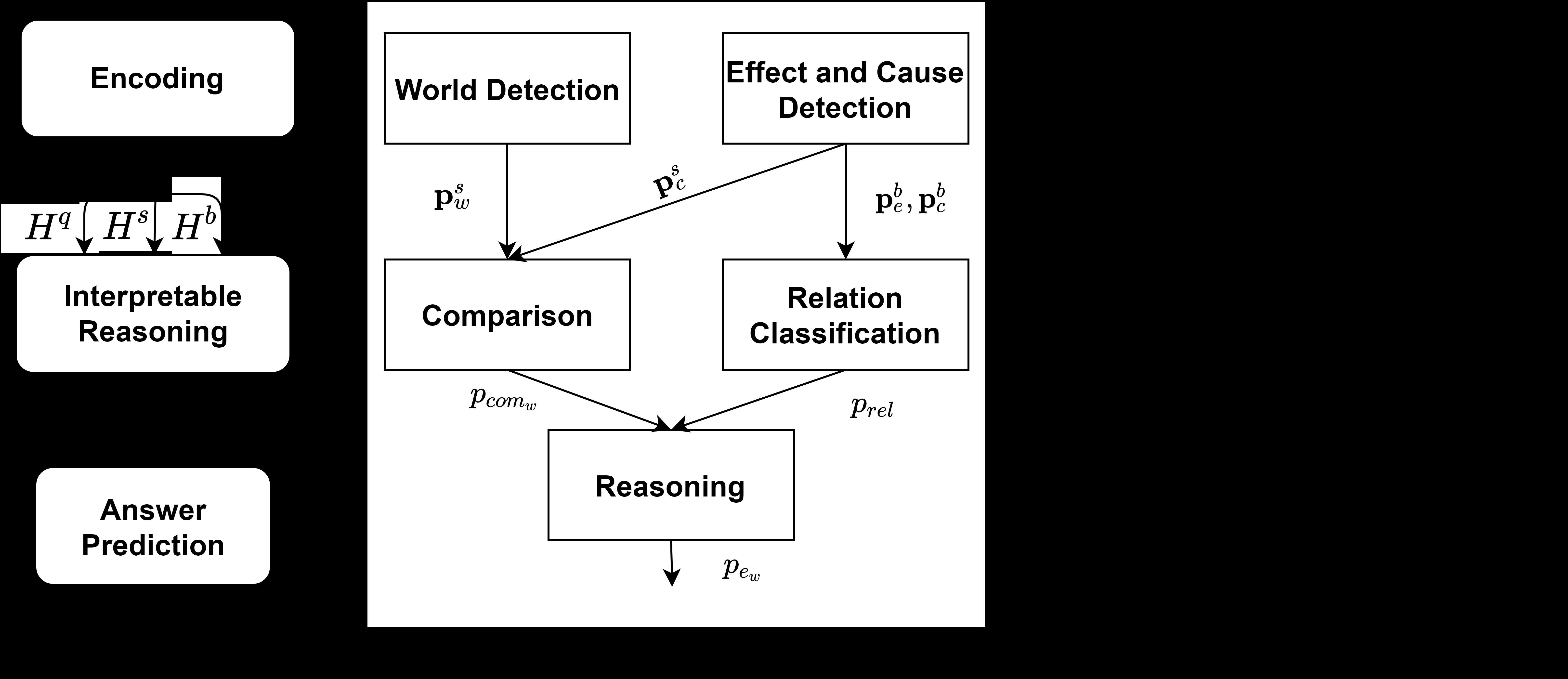
Experience Grounds Language
Yonatan Bisk, Ari Holtzman, Jesse Thomason, Jacob Andreas, Yoshua Bengio, Joyce Chai, Mirella Lapata, Angeliki Lazaridou, Jonathan May, Aleksandr Nisnevich, Nicolas Pinto, Joseph Turian,
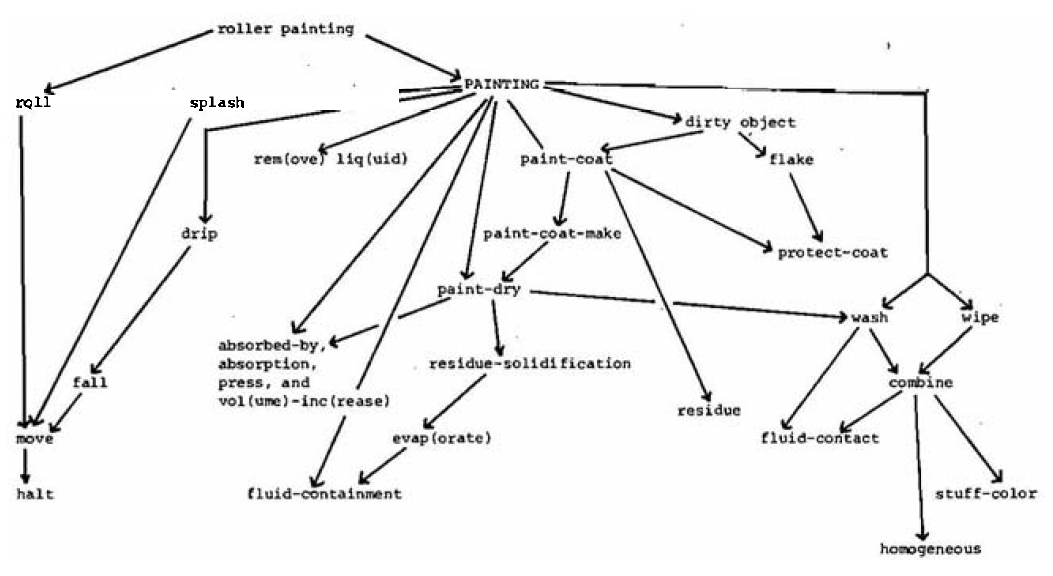