Position-Aware Tagging for Aspect Sentiment Triplet Extraction
Lu Xu, Hao Li, Wei Lu, Lidong Bing
Syntax: Tagging, Chunking, and Parsing Long Paper
You can open the pre-recorded video in a separate window.
Abstract:
Aspect Sentiment Triplet Extraction (ASTE) is the task of extracting the triplets of target entities, their associated sentiment, and opinion spans explaining the reason for the sentiment. Existing research efforts mostly solve this problem using pipeline approaches, which break the triplet extraction process into several stages. Our observation is that the three elements within a triplet are highly related to each other, and this motivates us to build a joint model to extract such triplets using a sequence tagging approach. However, how to effectively design a tagging approach to extract the triplets that can capture the rich interactions among the elements is a challenging research question. In this work, we propose the first end-to-end model with a novel position-aware tagging scheme that is capable of jointly extracting the triplets. Our experimental results on several existing datasets show that jointly capturing elements in the triplet using our approach leads to improved performance over the existing approaches. We also conducted extensive experiments to investigate the model effectiveness and robustness.
NOTE: Video may display a random order of authors.
Correct author list is at the top of this page.
Connected Papers in EMNLP2020
Similar Papers
Aspect Sentiment Classification with Aspect-Specific Opinion Spans
Lu Xu, Lidong Bing, Wei Lu, Fei Huang,
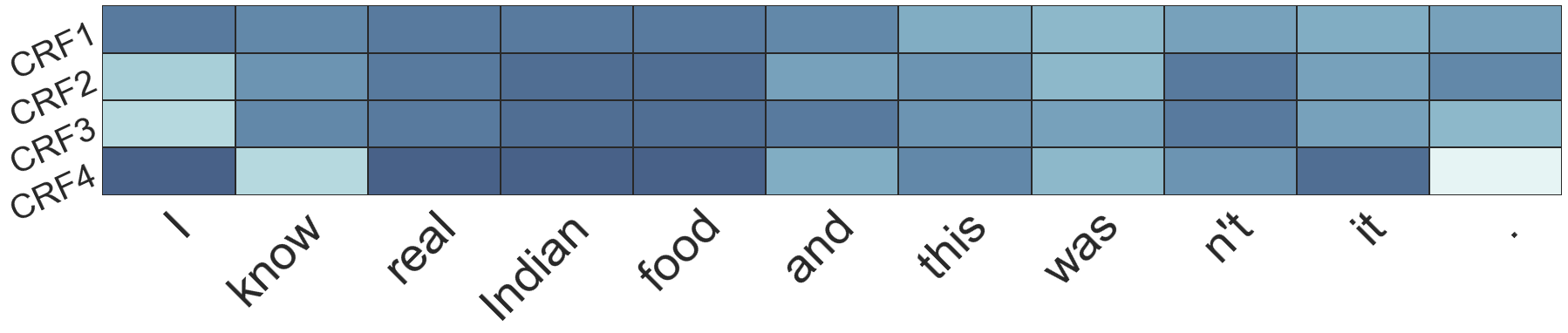
Introducing Syntactic Structures into Target Opinion Word Extraction with Deep Learning
Amir Pouran Ben Veyseh, Nasim Nouri, Franck Dernoncourt, Dejing Dou, Thien Huu Nguyen,

Multi-resolution Annotations for Emoji Prediction
Weicheng Ma, Ruibo Liu, Lili Wang, Soroush Vosoughi,
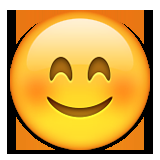