Multi-resolution Annotations for Emoji Prediction
Weicheng Ma, Ruibo Liu, Lili Wang, Soroush Vosoughi
NLP Applications Long Paper
You can open the pre-recorded video in a separate window.
Abstract:
Emojis are able to express various linguistic components, including emotions, sentiments, events, etc. Predicting the proper emojis associated with text provides a way to summarize the text accurately, and it has been proven to be a good auxiliary task to many Natural Language Understanding (NLU) tasks. Labels in existing emoji prediction datasets are all passage-based and are usually under the multi-class classification setting. However, in many cases, one single emoji cannot fully cover the theme of a piece of text. It is thus useful to infer the part of text related to each emoji. The lack of multi-label and aspect-level emoji prediction datasets is one of the bottlenecks for this task. This paper annotates an emoji prediction dataset with passage-level multi-class/multi-label, and aspect-level multi-class annotations. We also present a novel annotation method with which we generate the aspect-level annotations. The annotations are generated heuristically, taking advantage of the self-attention mechanism in Transformer networks. We validate the annotations both automatically and manually to ensure their quality. We also benchmark the dataset with a pre-trained BERT model.
NOTE: Video may display a random order of authors.
Correct author list is at the top of this page.
Connected Papers in EMNLP2020
Similar Papers
Neural Extractive Summarization with Hierarchical Attentive Heterogeneous Graph Network
Ruipeng Jia, Yanan Cao, Hengzhu Tang, Fang Fang, Cong Cao, Shi Wang,
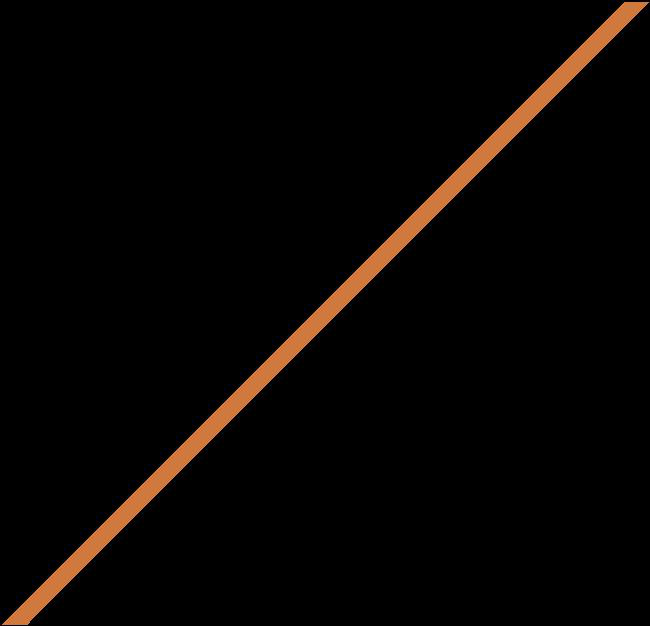
Weakly-Supervised Aspect-Based Sentiment Analysis via Joint Aspect-Sentiment Topic Embedding
Jiaxin Huang, Yu Meng, Fang Guo, Heng Ji, Jiawei Han,

MODE-LSTM: A Parameter-efficient Recurrent Network with Multi-Scale for Sentence Classification
Qianli Ma, Zhenxi Lin, Jiangyue Yan, Zipeng Chen, Liuhong Yu,
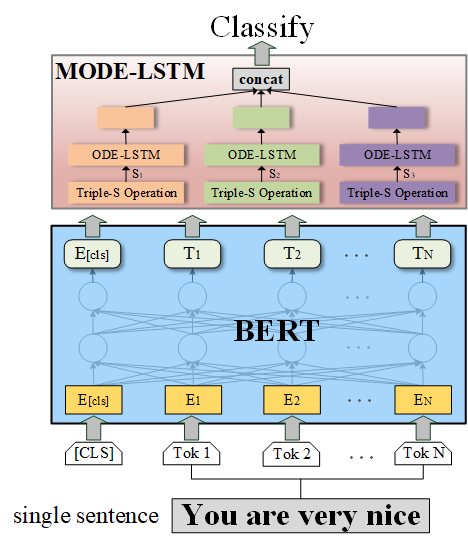