Assessing Phrasal Representation and Composition in Transformers
Lang Yu, Allyson Ettinger
Interpretability and Analysis of Models for NLP Long Paper
You can open the pre-recorded video in a separate window.
Abstract:
Deep transformer models have pushed performance on NLP tasks to new limits, suggesting sophisticated treatment of complex linguistic inputs, such as phrases. However, we have limited understanding of how these models handle representation of phrases, and whether this reflects sophisticated composition of phrase meaning like that done by humans. In this paper, we present systematic analysis of phrasal representations in state-of-the-art pre-trained transformers. We use tests leveraging human judgments of phrase similarity and meaning shift, and compare results before and after control of word overlap, to tease apart lexical effects versus composition effects. We find that phrase representation in these models relies heavily on word content, with little evidence of nuanced composition. We also identify variations in phrase representation quality across models, layers, and representation types, and make corresponding recommendations for usage of representations from these models.
NOTE: Video may display a random order of authors.
Correct author list is at the top of this page.
Connected Papers in EMNLP2020
Similar Papers
Syntactic Structure Distillation Pretraining for Bidirectional Encoders
Adhiguna Kuncoro, Lingpeng Kong, Daniel Fried, Dani Yogatama, Laura Rimell, Chris Dyer, Phil Blunsom,

ETC: Encoding Long and Structured Inputs in Transformers
Joshua Ainslie, Santiago Ontanon, Chris Alberti, Vaclav Cvicek, Zachary Fisher, Philip Pham, Anirudh Ravula, Sumit Sanghai, Qifan Wang, Li Yang,
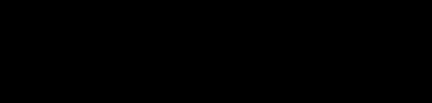
Compositional and Lexical Semantics in RoBERTa, BERT and DistilBERT: A Case Study on CoQA
Ieva Staliūnaitė, Ignacio Iacobacci,

Grounded Compositional Outputs for Adaptive Language Modeling
Nikolaos Pappas, Phoebe Mulcaire, Noah A. Smith,
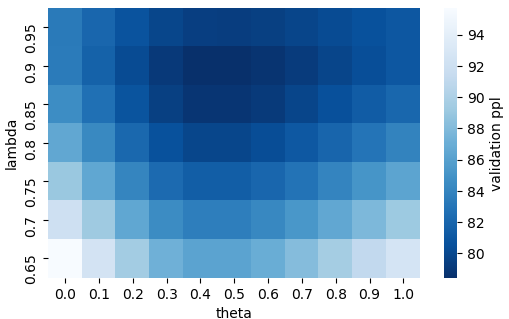