Sparsity Makes Sense: Word Sense Disambiguation Using Sparse Contextualized Word Representations
Gábor Berend
Semantics: Lexical Semantics Long Paper
You can open the pre-recorded video in a separate window.
Abstract:
In this paper, we demonstrate that by utilizing sparse word representations, it becomes possible to surpass the results of more complex task-specific models on the task of fine-grained all-words word sense disambiguation. Our proposed algorithm relies on an overcomplete set of semantic basis vectors that allows us to obtain sparse contextualized word representations. We introduce such an information theory-inspired synset representation based on the co-occurrence of word senses and non-zero coordinates for word forms which allows us to achieve an aggregated F-score of 78.8 over a combination of five standard word sense disambiguating benchmark datasets. We also demonstrate the general applicability of our proposed framework by evaluating it towards part-of-speech tagging on four different treebanks. Our results indicate a significant improvement over the application of the dense word representations.
NOTE: Video may display a random order of authors.
Correct author list is at the top of this page.
Connected Papers in EMNLP2020
Similar Papers
With More Contexts Comes Better Performance: Contextualized Sense Embeddings for All-Round Word Sense Disambiguation
Bianca Scarlini, Tommaso Pasini, Roberto Navigli,
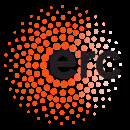
A Synset Relation-enhanced Framework with a Try-again Mechanism for Word Sense Disambiguation
Ming Wang, Yinglin Wang,

Improving Word Sense Disambiguation with Translations
Yixing Luan, Bradley Hauer, Lili Mou, Grzegorz Kondrak,
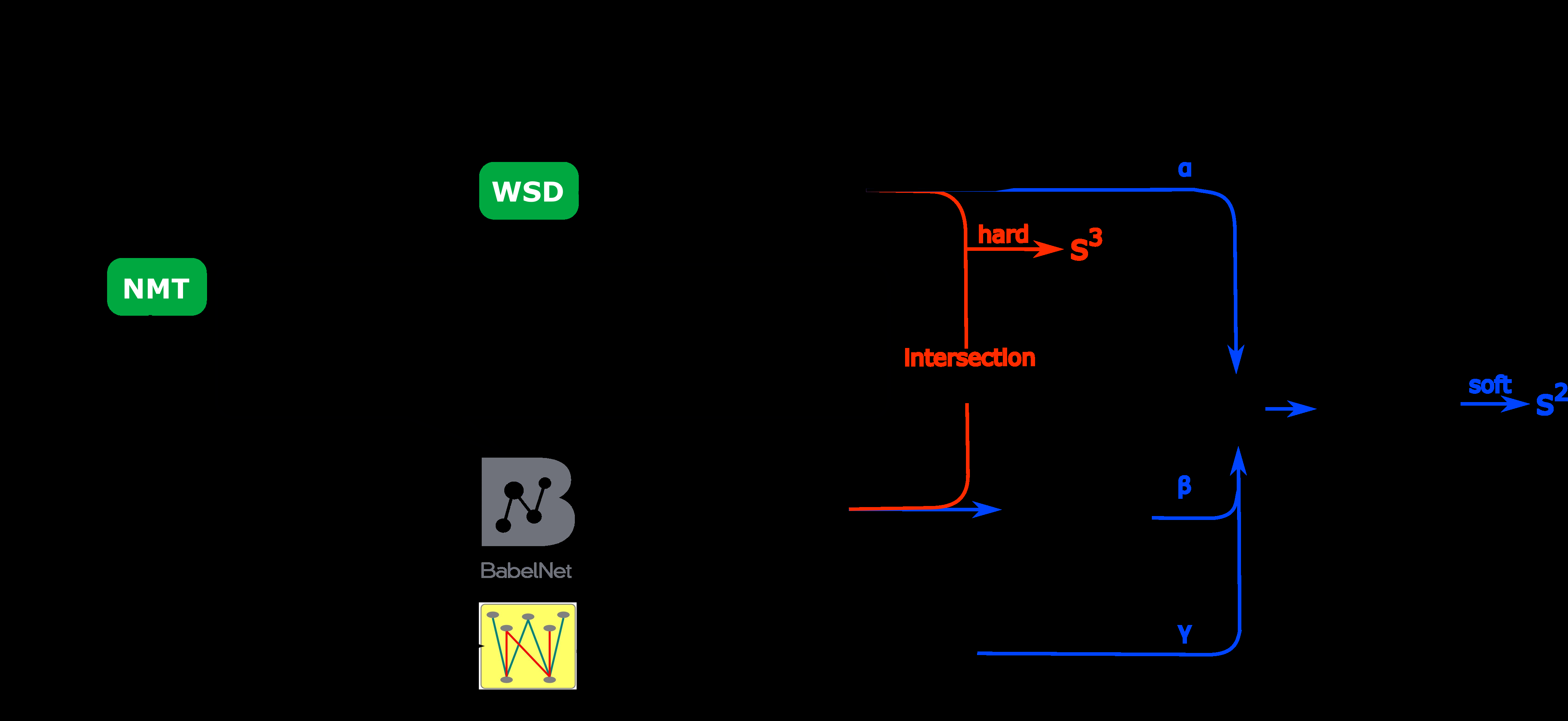
Speakers Fill Lexical Semantic Gaps with Context
Tiago Pimentel, Rowan Hall Maudslay, Damian Blasi, Ryan Cotterell,
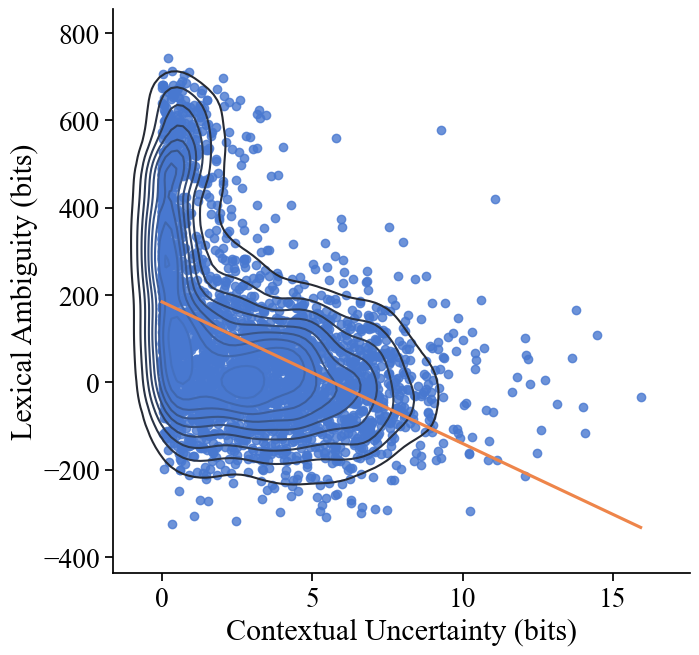