HSCNN: A Hybrid-Siamese Convolutional Neural Network for Extremely Imbalanced Multi-label Text Classification
Wenshuo Yang, Jiyi Li, Fumiyo Fukumoto, Yanming Ye
NLP Applications Short Paper
Abstract:
The data imbalance problem is a crucial issue for the multi-label text classification. Some existing works tackle it by proposing imbalanced loss objectives instead of the vanilla cross-entropy loss, but their performances remain limited in the cases of extremely imbalanced data. We propose a hybrid solution which adapts general networks for the head categories, and few-shot techniques for the tail categories. We propose a Hybrid-Siamese Convolutional Neural Network (HSCNN) with additional technical attributes, i.e., a multi-task architecture based on Single and Siamese networks; a category-specific similarity in the Siamese structure; a specific sampling method for training HSCNN. The results using two benchmark datasets and three loss objectives show that our method can improve the performance of Single networks with diverse loss objectives on the tail or entire categories.
Connected Papers in EMNLP2020
Similar Papers
SetConv: A New Approach for Learning from Imbalanced Data
Yang Gao, Yi-Fan Li, Yu Lin, Charu Aggarwal, Latifur Khan,
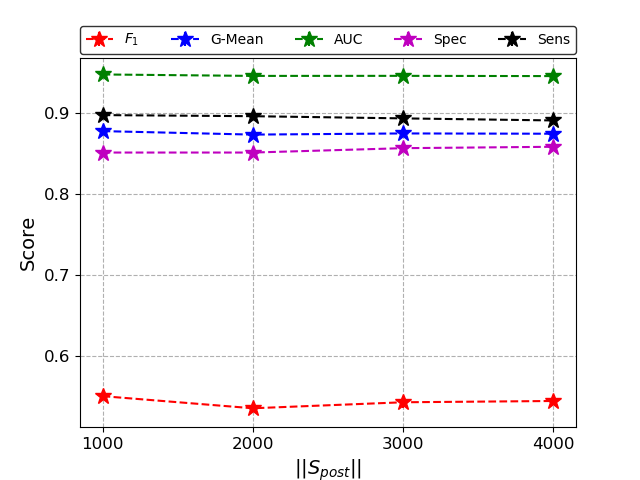
Active Learning for BERT: An Empirical Study
Liat Ein-Dor, Alon Halfon, Ariel Gera, Eyal Shnarch, Lena Dankin, Leshem Choshen, Marina Danilevsky, Ranit Aharonov, Yoav Katz, Noam Slonim,
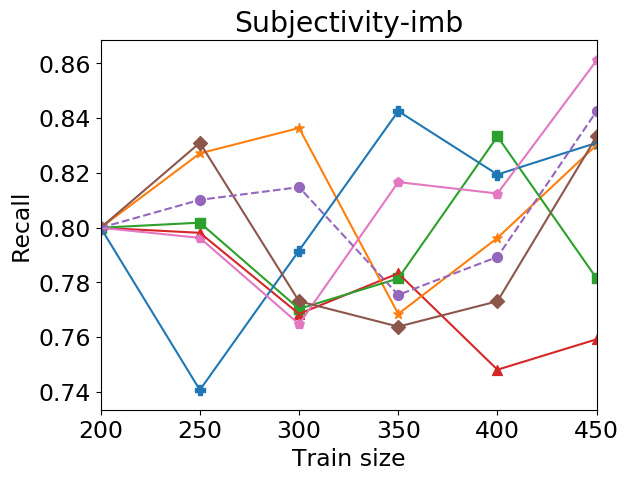
Adversarial Self-Supervised Data-Free Distillation for Text Classification
Xinyin Ma, Yongliang Shen, Gongfan Fang, Chen Chen, Chenghao Jia, Weiming Lu,

A Multi-Task Incremental Learning Framework with Category Name Embedding for Aspect-Category Sentiment Analysis
Zehui Dai, Cheng Peng, Huajie Chen, Yadong Ding,
