Unsupervised Adaptation of Question Answering Systems via Generative Self-training
Steven Rennie, Etienne Marcheret, Neil Mallinar, David Nahamoo, Vaibhava Goel
Question Answering Long Paper
You can open the pre-recorded video in a separate window.
Abstract:
BERT-era question answering systems have recently achieved impressive performance on several question-answering (QA) tasks. These systems are based on representations that have been pre-trained on self-supervised tasks such as word masking and sentence entailment, using massive amounts of data. Nevertheless, additional pre-training closer to the end-task, such as training on synthetic QA pairs, has been shown to improve performance. While recent work has considered augmenting labelled data and leveraging large unlabelled datasets to generate synthetic QA data, directly adapting to target data has received little attention. In this paper we investigate the iterative generation of synthetic QA pairs as a way to realize unsupervised self adaptation. Motivated by the success of the roundtrip consistency method for filtering generated QA pairs, we present iterative generalizations of the approach, which maximize an approximation of a lower bound on the probability of the adaptation data. By adapting on synthetic QA pairs generated on the target data, our method is able to improve QA systems significantly, using an order of magnitude less synthetic data and training computation than existing augmentation approaches.
NOTE: Video may display a random order of authors.
Correct author list is at the top of this page.
Connected Papers in EMNLP2020
Similar Papers
Unsupervised Question Decomposition for Question Answering
Ethan Perez, Patrick Lewis, Wen-tau Yih, Kyunghyun Cho, Douwe Kiela,

MUTANT: A Training Paradigm for Out-of-Distribution Generalization in Visual Question Answering
Tejas Gokhale, Pratyay Banerjee, Chitta Baral, Yezhou Yang,
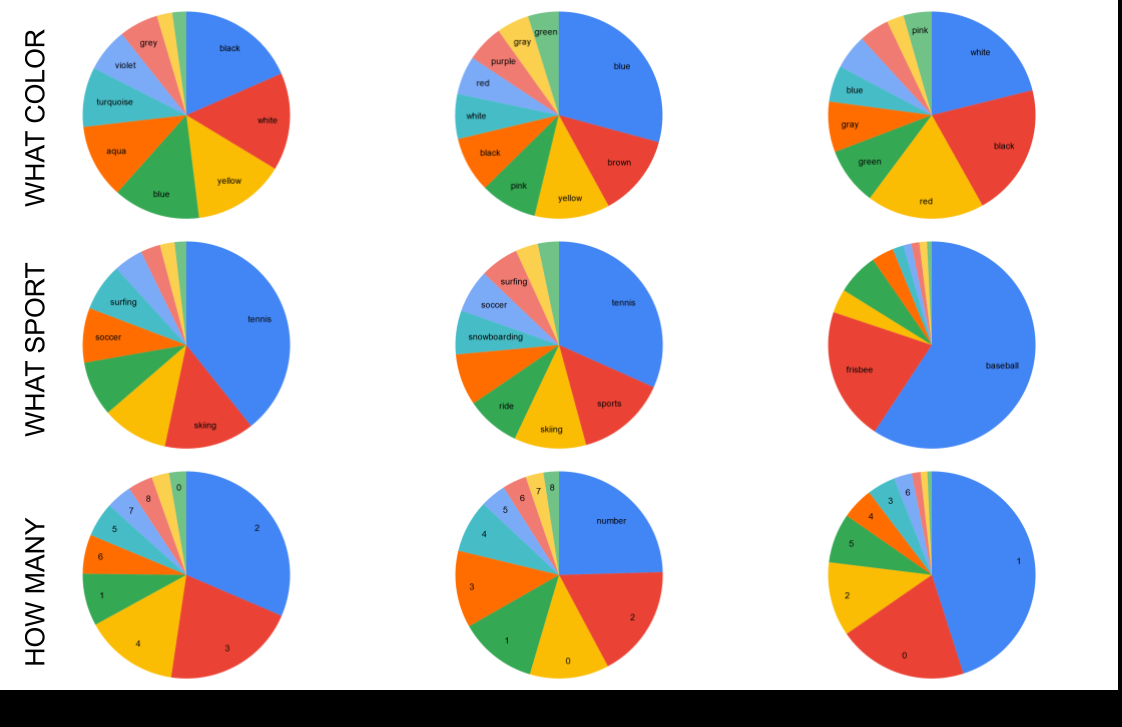
Cross-Thought for Sentence Encoder Pre-training
Shuohang Wang, Yuwei Fang, Siqi Sun, Zhe Gan, Yu Cheng, Jingjing Liu, Jing Jiang,
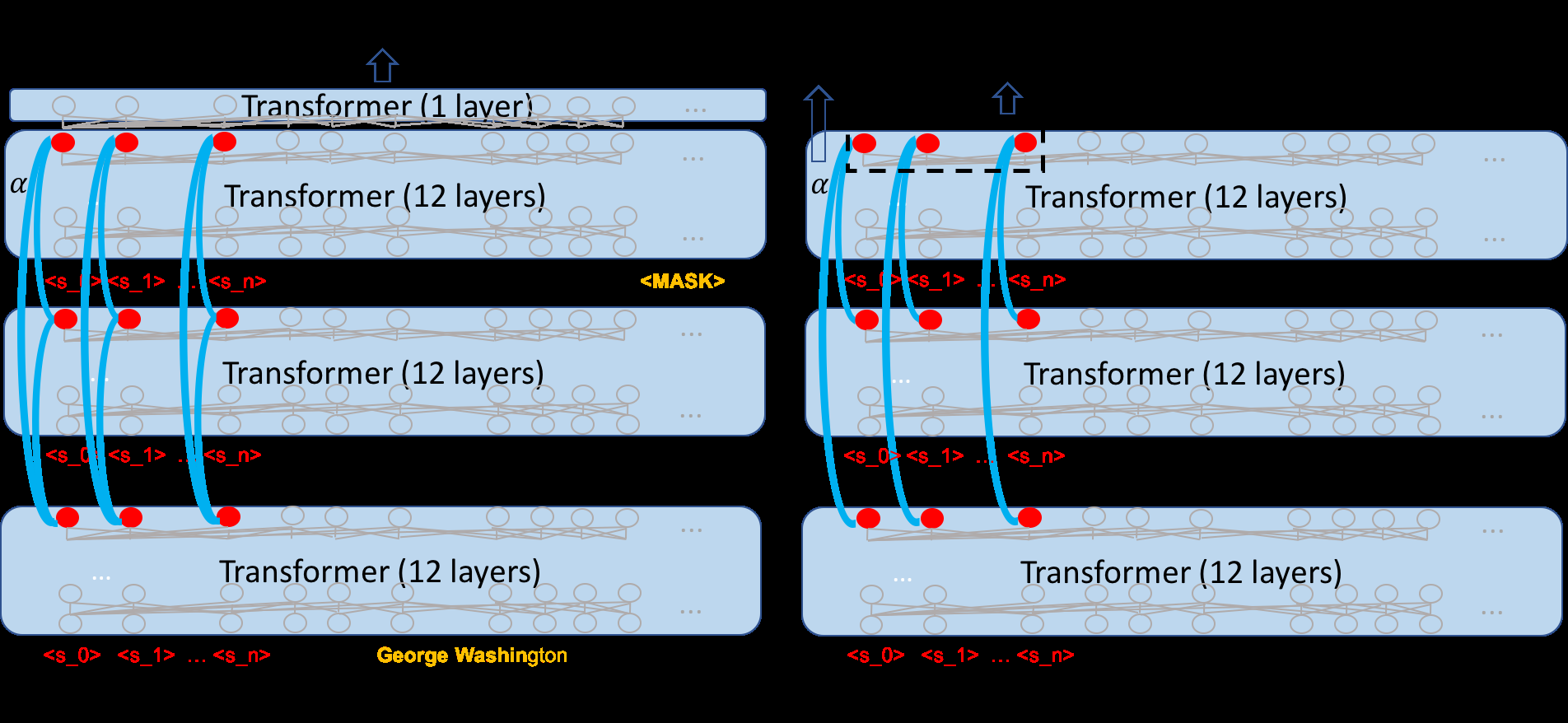
Don't Read Too Much Into It: Adaptive Computation for Open-Domain Question Answering
Yuxiang Wu, Sebastian Riedel, Pasquale Minervini, Pontus Stenetorp,
