A Method for Building a Commonsense Inference Dataset based on Basic Events
Kazumasa Omura, Daisuke Kawahara, Sadao Kurohashi
Semantics: Sentence-level Semantics, Textual Inference and Other areas Long Paper
You can open the pre-recorded video in a separate window.
Abstract:
We present a scalable, low-bias, and low-cost method for building a commonsense inference dataset that combines automatic extraction from a corpus and crowdsourcing. Each problem is a multiple-choice question that asks contingency between basic events. We applied the proposed method to a Japanese corpus and acquired 104k problems. While humans can solve the resulting problems with high accuracy (88.9%), the accuracy of a high-performance transfer learning model is reasonably low (76.0%). We also confirmed through dataset analysis that the resulting dataset contains low bias. We released the datatset to facilitate language understanding research.
NOTE: Video may display a random order of authors.
Correct author list is at the top of this page.
Connected Papers in EMNLP2020
Similar Papers
Annotating Temporal Dependency Graphs via Crowdsourcing
Jiarui Yao, Haoling Qiu, Bonan Min, Nianwen Xue,
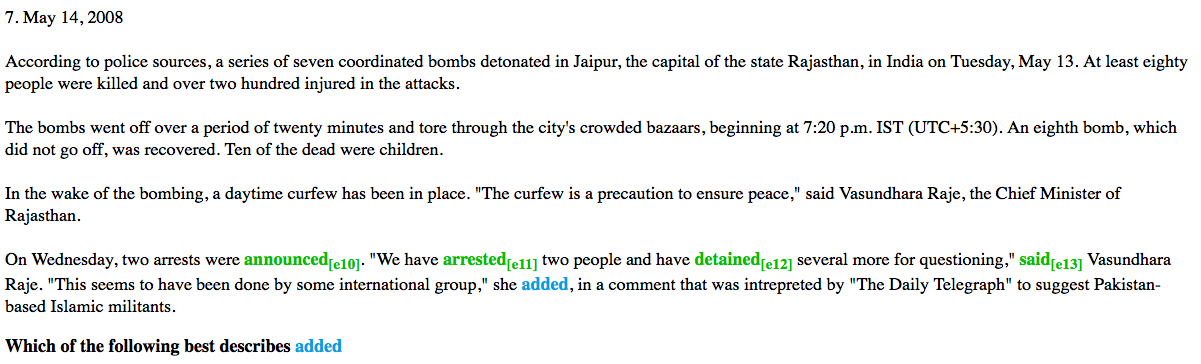
Small but Mighty: New Benchmarks for Split and Rephrase
Li Zhang, Huaiyu Zhu, Siddhartha Brahma, Yunyao Li,
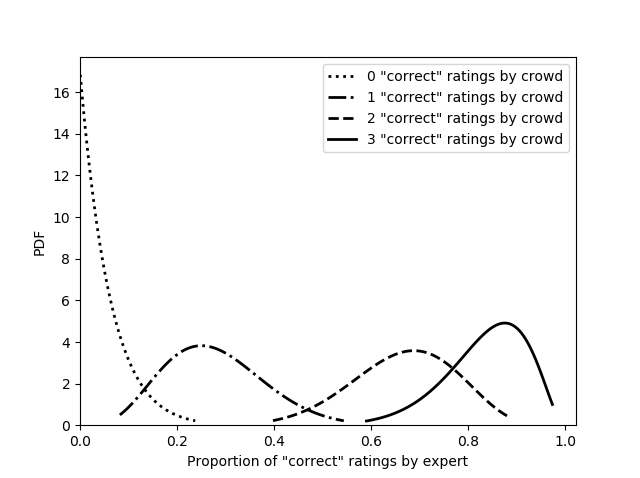
ALICE: Active Learning with Contrastive Natural Language Explanations
Weixin Liang, James Zou, Zhou Yu,
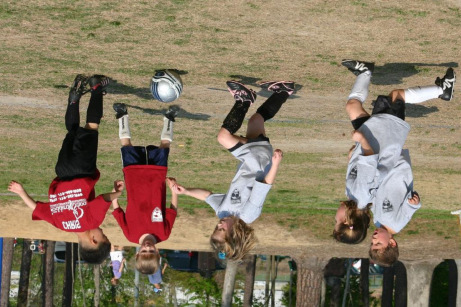