A Dataset for Tracking Entities in Open Domain Procedural Text
Niket Tandon, Keisuke Sakaguchi, Bhavana Dalvi, Dheeraj Rajagopal, Peter Clark, Michal Guerquin, Kyle Richardson, Eduard Hovy
Semantics: Sentence-level Semantics, Textual Inference and Other areas Long Paper
You can open the pre-recorded video in a separate window.
Abstract:
We present the first dataset for tracking state changes in procedural text from arbitrary domains by using an unrestricted (open) vocabulary. For example, in a text describing fog removal using potatoes, a car window may transition between being foggy, sticky, opaque, and clear. Previous formulations of this task provide the text and entities involved, and ask how those entities change for just a small, pre-defined set of attributes (e.g., location), limiting their fidelity. Our solution is a new task formulation where given just a procedural text as input, the task is to generate a set of state change tuples (entity, attribute, before-state, after-state) for each step, where the entity, attribute, and state values must be predicted from an open vocabulary. Using crowdsourcing, we create OPENPI, a high-quality (91.5% coverage as judged by humans and completely vetted), and large-scale dataset comprising 29,928 state changes over 4,050 sentences from 810 procedural real-world paragraphs from WikiHow.com. A current state-of-the-art generation model on this task achieves 16.1% F1 based on BLEU metric, leaving enough room for novel model architectures.
NOTE: Video may display a random order of authors.
Correct author list is at the top of this page.
Connected Papers in EMNLP2020
Similar Papers
TESA: A Task in Entity Semantic Aggregation for Abstractive Summarization
Clément Jumel, Annie Louis, Jackie Chi Kit Cheung,
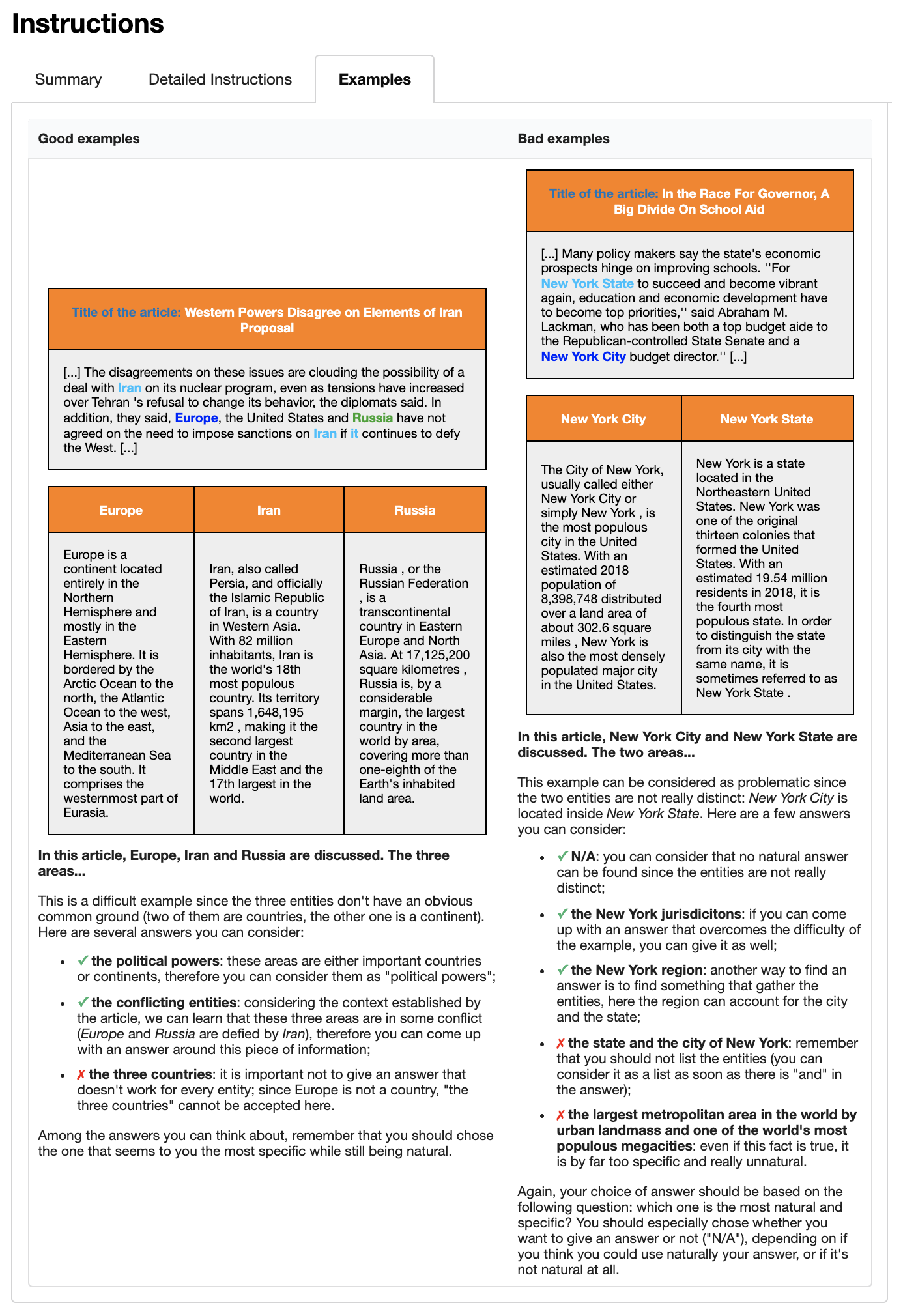
HUMAN: Hierarchical Universal Modular ANnotator
Moritz Wolf, Dana Ruiter, Ashwin Geet D'Sa, Liane Reiners, Jan Alexandersson, Dietrich Klakow,
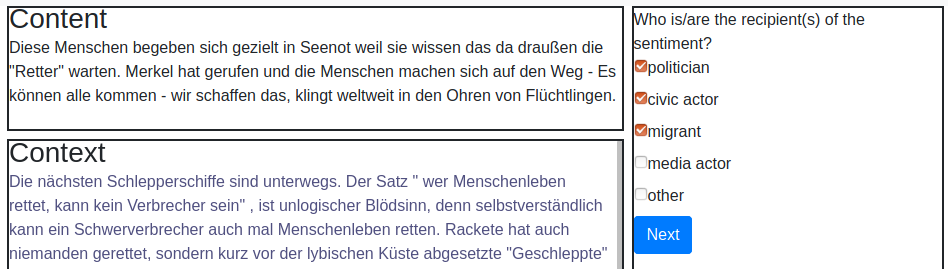
Scalable Zero-shot Entity Linking with Dense Entity Retrieval
Ledell Wu, Fabio Petroni, Martin Josifoski, Sebastian Riedel, Luke Zettlemoyer,

Incorporating Multimodal Information in Open-Domain Web Keyphrase Extraction
Yansen Wang, Zhen Fan, Carolyn Rose,
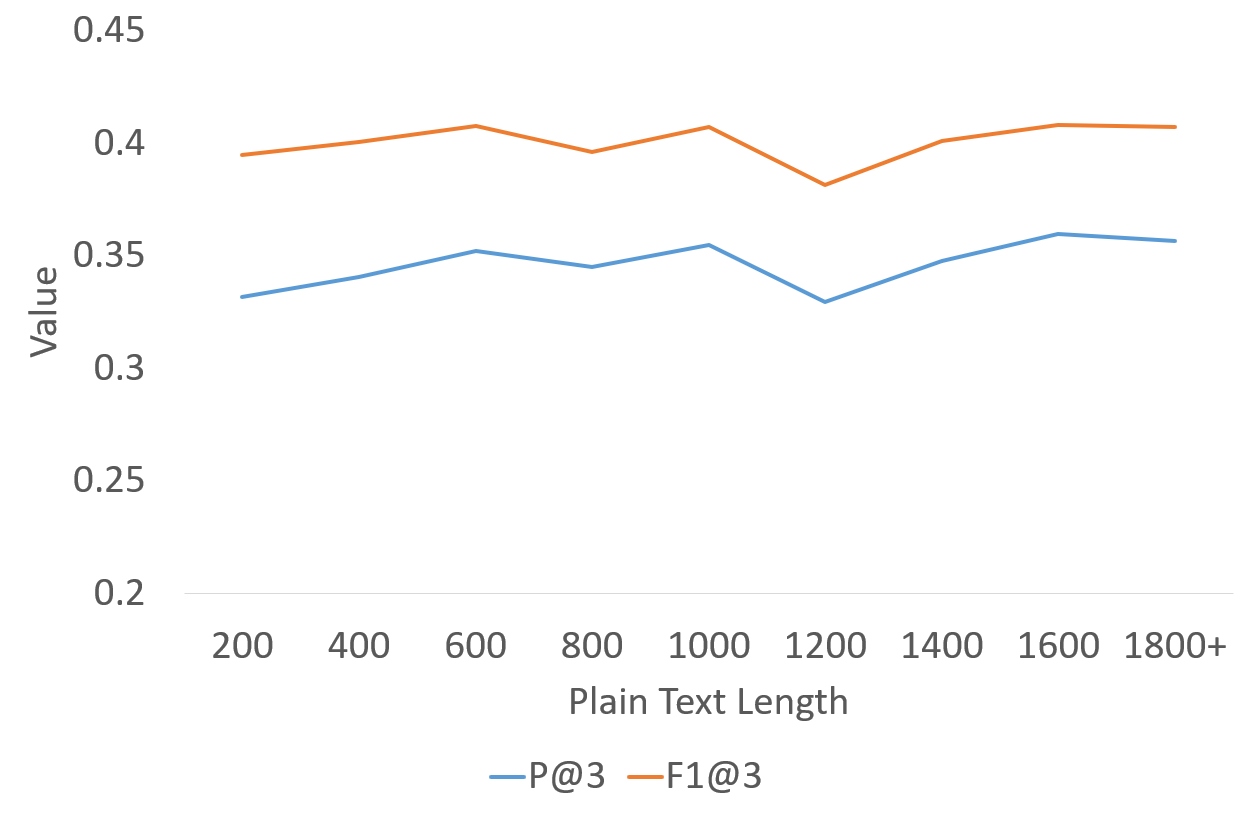