Scalable Zero-shot Entity Linking with Dense Entity Retrieval
Ledell Wu, Fabio Petroni, Martin Josifoski, Sebastian Riedel, Luke Zettlemoyer
Information Extraction Long Paper
You can open the pre-recorded video in a separate window.
Abstract:
This paper introduces a conceptually simple, scalable, and highly effective BERT-based entity linking model, along with an extensive evaluation of its accuracy-speed trade-off. We present a two-stage zero-shot linking algorithm, where each entity is defined only by a short textual description. The first stage does retrieval in a dense space defined by a bi-encoder that independently embeds the mention context and the entity descriptions. Each candidate is then re-ranked with a cross-encoder, that concatenates the mention and entity text. Experiments demonstrate that this approach is state of the art on recent zero-shot benchmarks (6 point absolute gains) and also on more established non-zero-shot evaluations (e.g. TACKBP-2010), despite its relative simplicity (e.g. no explicit entity embeddings or manually engineered mention tables). We also show that bi-encoder linking is very fast with nearest neighbor search (e.g. linking with 5.9 million candidates in 2 milliseconds), and that much of the accuracy gain from the more expensive cross-encoder can be transferred to the bi-encoder via knowledge distillation. Our code and models are available at https://github.com/facebookresearch/BLINK.
NOTE: Video may display a random order of authors.
Correct author list is at the top of this page.
Connected Papers in EMNLP2020
Similar Papers
Adaptive Attentional Network for Few-Shot Knowledge Graph Completion
Jiawei Sheng, Shu Guo, Zhenyu Chen, Juwei Yue, Lihong Wang, Tingwen Liu, Hongbo Xu,

Exploring and Evaluating Attributes, Values, and Structures for Entity Alignment
Zhiyuan Liu, Yixin Cao, Liangming Pan, Juanzi Li, Zhiyuan Liu, Tat-Seng Chua,

HIT: Nested Named Entity Recognition via Head-Tail Pair and Token Interaction
Yu Wang, Yun Li, Hanghang Tong, Ziye Zhu,
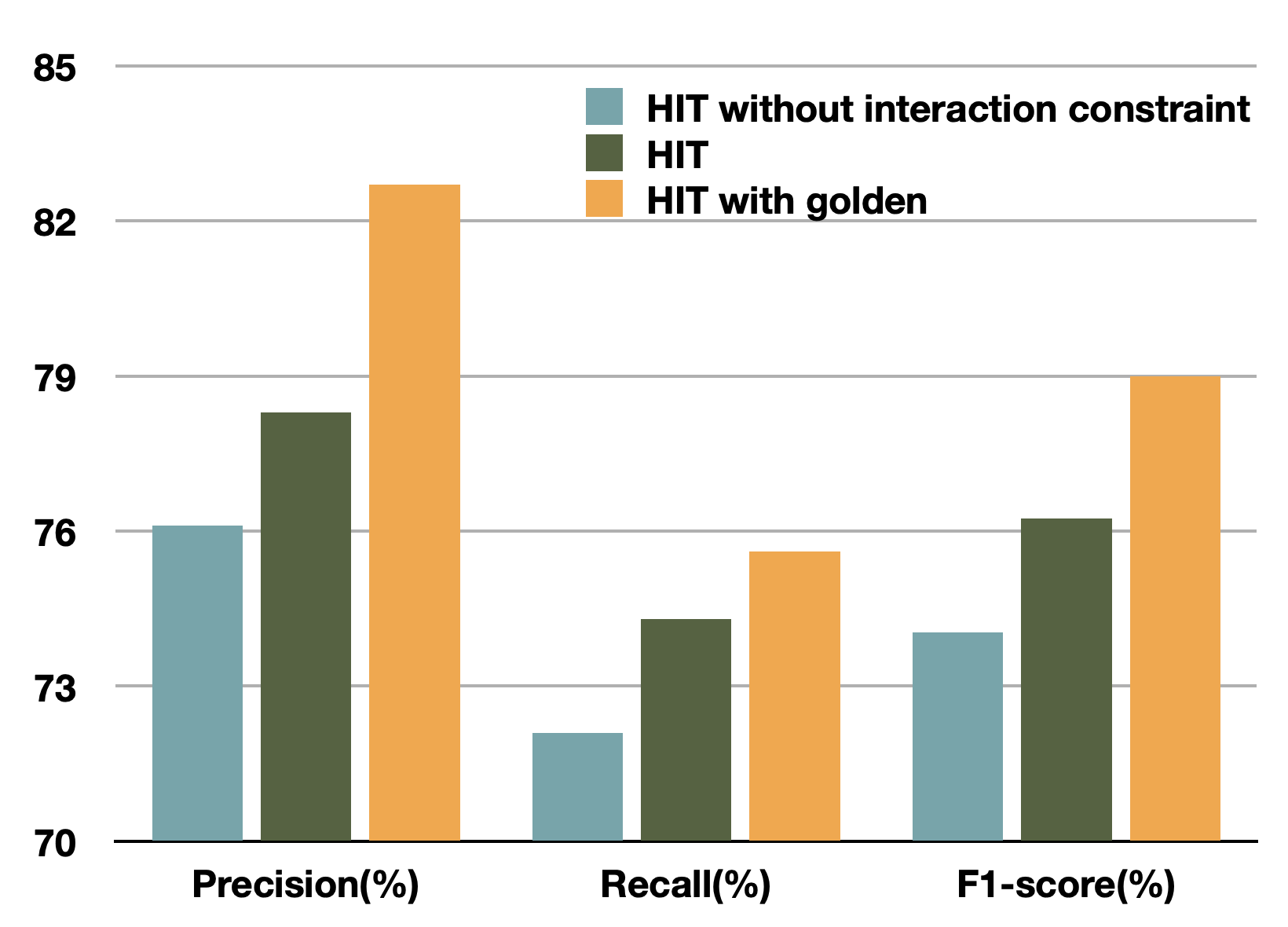