Incorporating Multimodal Information in Open-Domain Web Keyphrase Extraction
Yansen Wang, Zhen Fan, Carolyn Rose
Information Retrieval and Text Mining Long Paper
You can open the pre-recorded video in a separate window.
Abstract:
Open-domain Keyphrase extraction (KPE) on the Web is a fundamental yet complex NLP task with a wide range of practical applications within the field of Information Retrieval. In contrast to other document types, web page designs are intended for easy navigation and information finding. Effective designs encode within the layout and formatting signals that point to where the important information can be found. In this work, we propose a modeling approach that leverages these multi-modal signals to aid in the KPE task. In particular, we leverage both lexical and visual features (e.g., size, font, position) at the micro-level to enable effective strategy induction and meta-level features that describe pages at a macro-level to aid in strategy selection. Our evaluation demonstrates that a combination of effective strategy induction and strategy selection within this approach for the KPE task outperforms state-of-the-art models. A qualitative post-hoc analysis illustrates how these features function within the model.
NOTE: Video may display a random order of authors.
Correct author list is at the top of this page.
Connected Papers in EMNLP2020
Similar Papers
OpenUE: An Open Toolkit of Universal Extraction from Text
Ningyu Zhang, Shumin Deng, Zhen Bi, Haiyang Yu, Jiacheng Yang, Mosha Chen, Fei Huang, Wei Zhang, Huajun Chen,
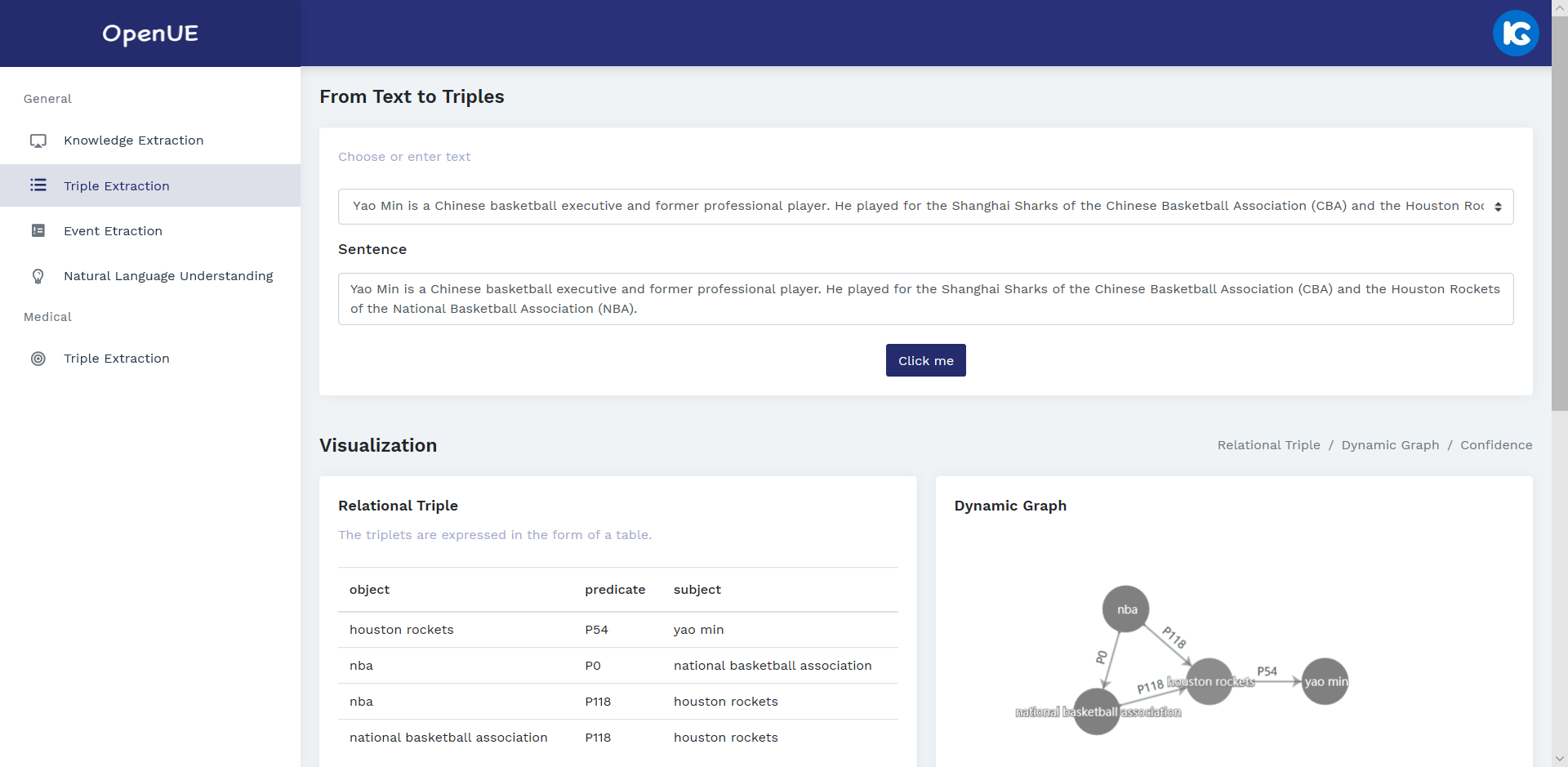
Form2Seq : A Framework for Higher-Order Form Structure Extraction
Milan Aggarwal, Hiresh Gupta, Mausoom Sarkar, Balaji Krishnamurthy,
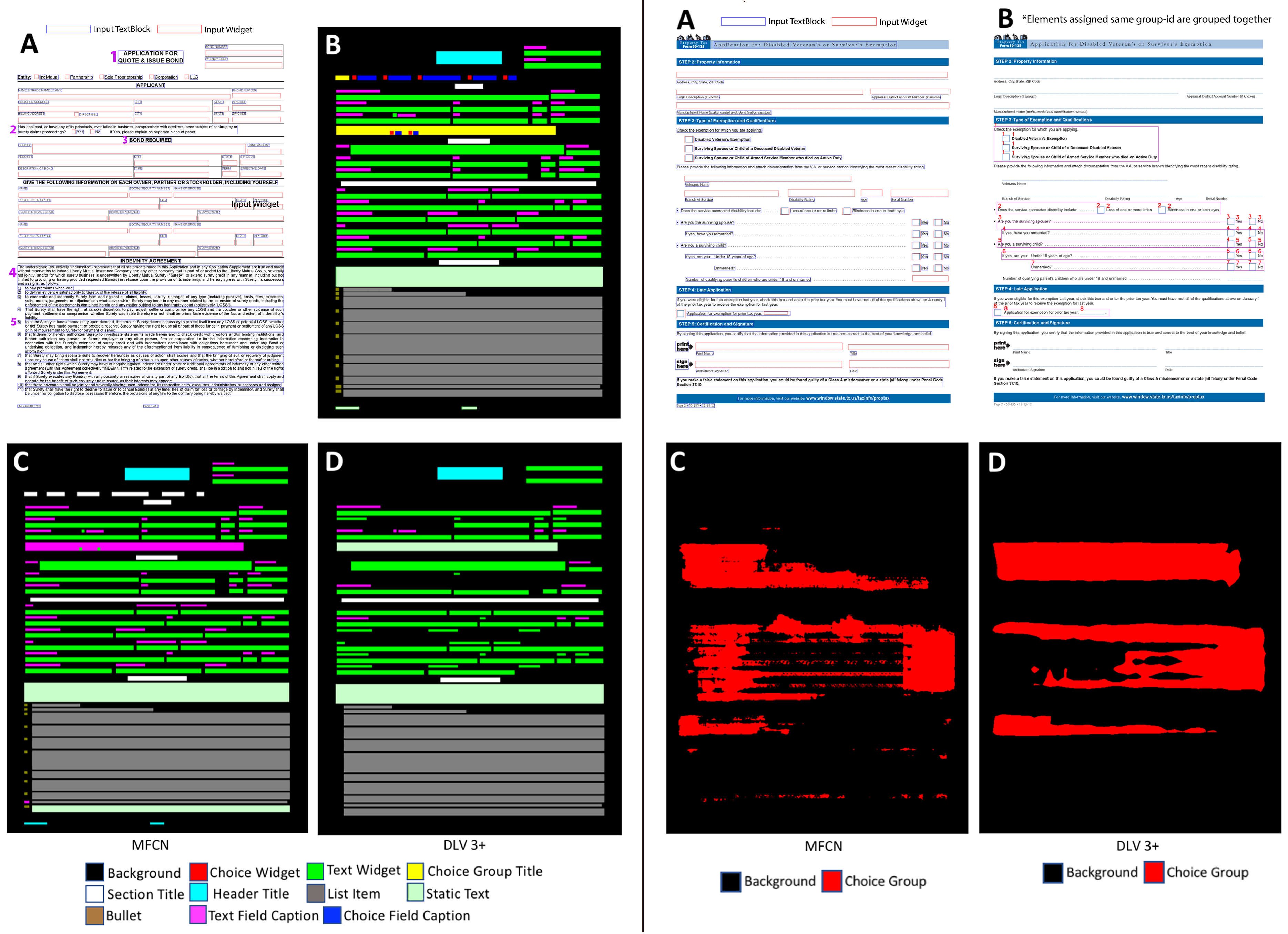
Design Challenges in Low-resource Cross-lingual Entity Linking
Xingyu Fu, Weijia Shi, Xiaodong Yu, Zian Zhao, Dan Roth,
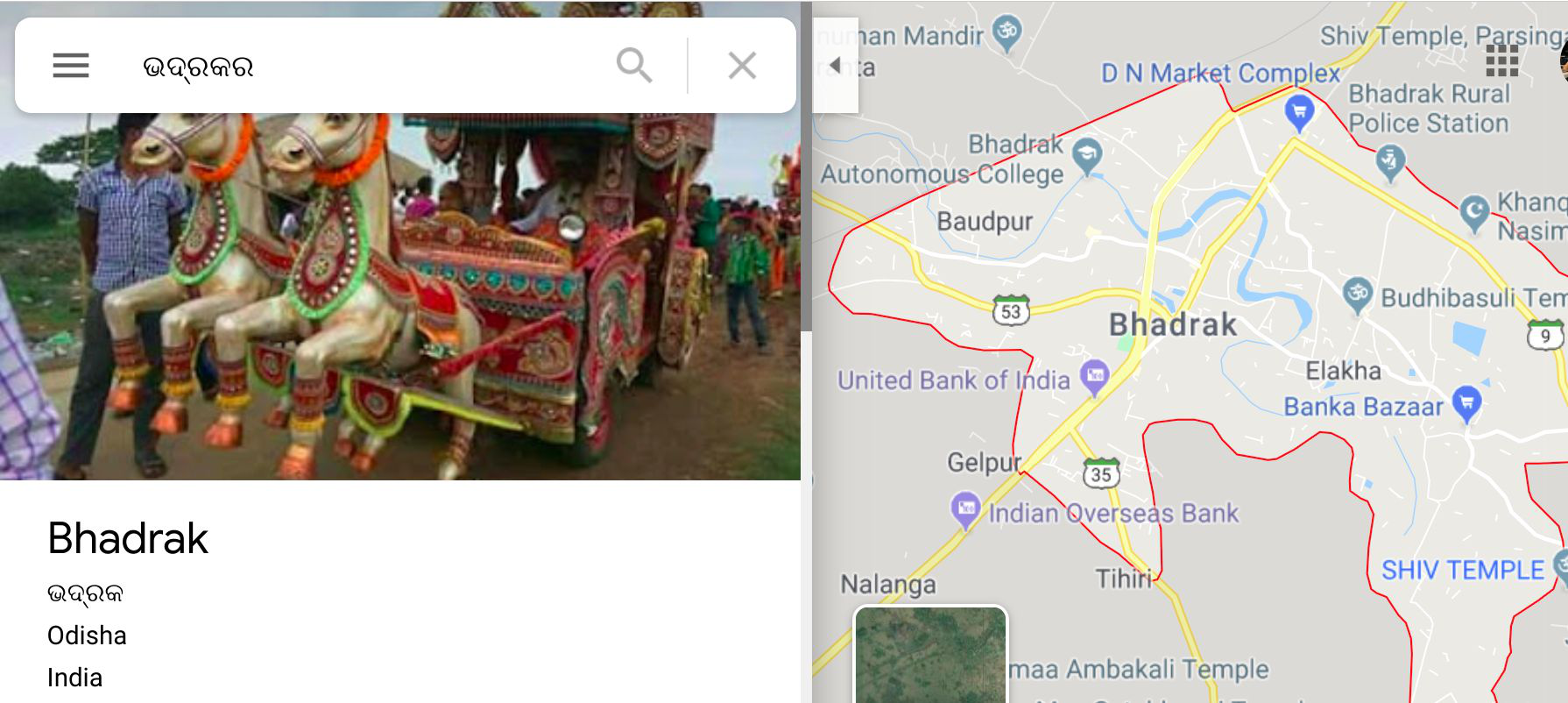
Quantitative argument summarization and beyond: Cross-domain key point analysis
Roy Bar-Haim, Yoav Kantor, Lilach Eden, Roni Friedman, Dan Lahav, Noam Slonim,
