Zero-Shot Stance Detection: A Dataset and Model using Generalized Topic Representations
Emily Allaway, Kathleen McKeown
Sentiment Analysis, Stylistic Analysis, and Argument Mining Long Paper
You can open the pre-recorded video in a separate window.
Abstract:
Stance detection is an important component of understanding hidden influences in everyday life. Since there are thousands of potential topics to take a stance on, most with little to no training data, we focus on zero-shot stance detection: classifying stance from no training examples. In this paper, we present a new dataset for zero-shot stance detection that captures a wider range of topics and lexical variation than in previous datasets. Additionally, we propose a new model for stance detection that implicitly captures relationships between topics using generalized topic representations and show that this model improves performance on a number of challenging linguistic phenomena.
NOTE: Video may display a random order of authors.
Correct author list is at the top of this page.
Connected Papers in EMNLP2020
Similar Papers
Tired of Topic Models? Clusters of Pretrained Word Embeddings Make for Fast and Good Topics too!
Suzanna Sia, Ayush Dalmia, Sabrina J. Mielke,
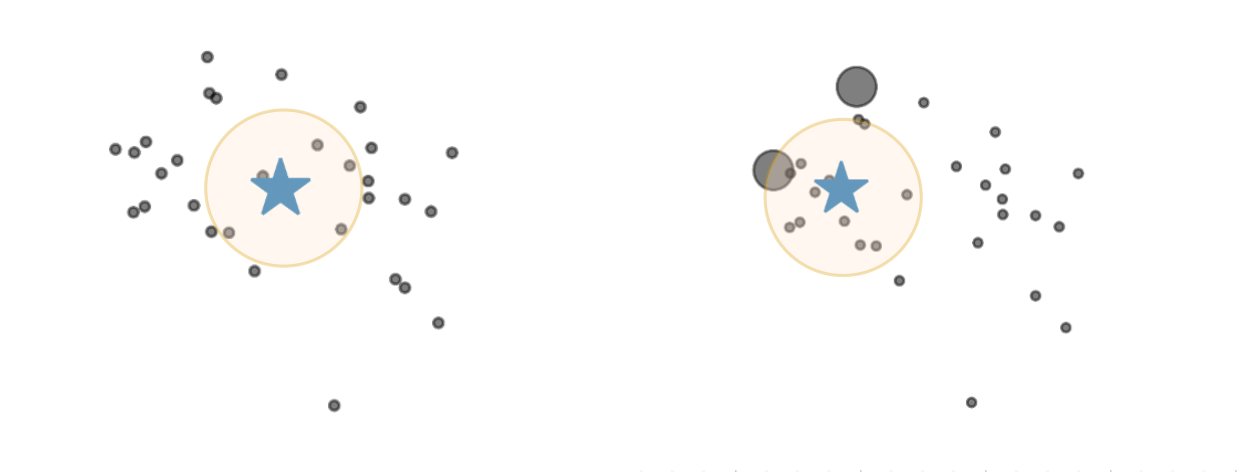
Unsupervised stance detection for arguments from consequences
Jonathan Kobbe, Ioana Hulpus, Heiner Stuckenschmidt,

Short Text Topic Modeling with Topic Distribution Quantization and Negative Sampling Decoder
Xiaobao Wu, Chunping Li, Yan Zhu, Yishu Miao,

Hashtags, Emotions, and Comments: A Large-Scale Dataset to Understand Fine-Grained Social Emotions to Online Topics
Keyang Ding, Jing Li, Yuji Zhang,
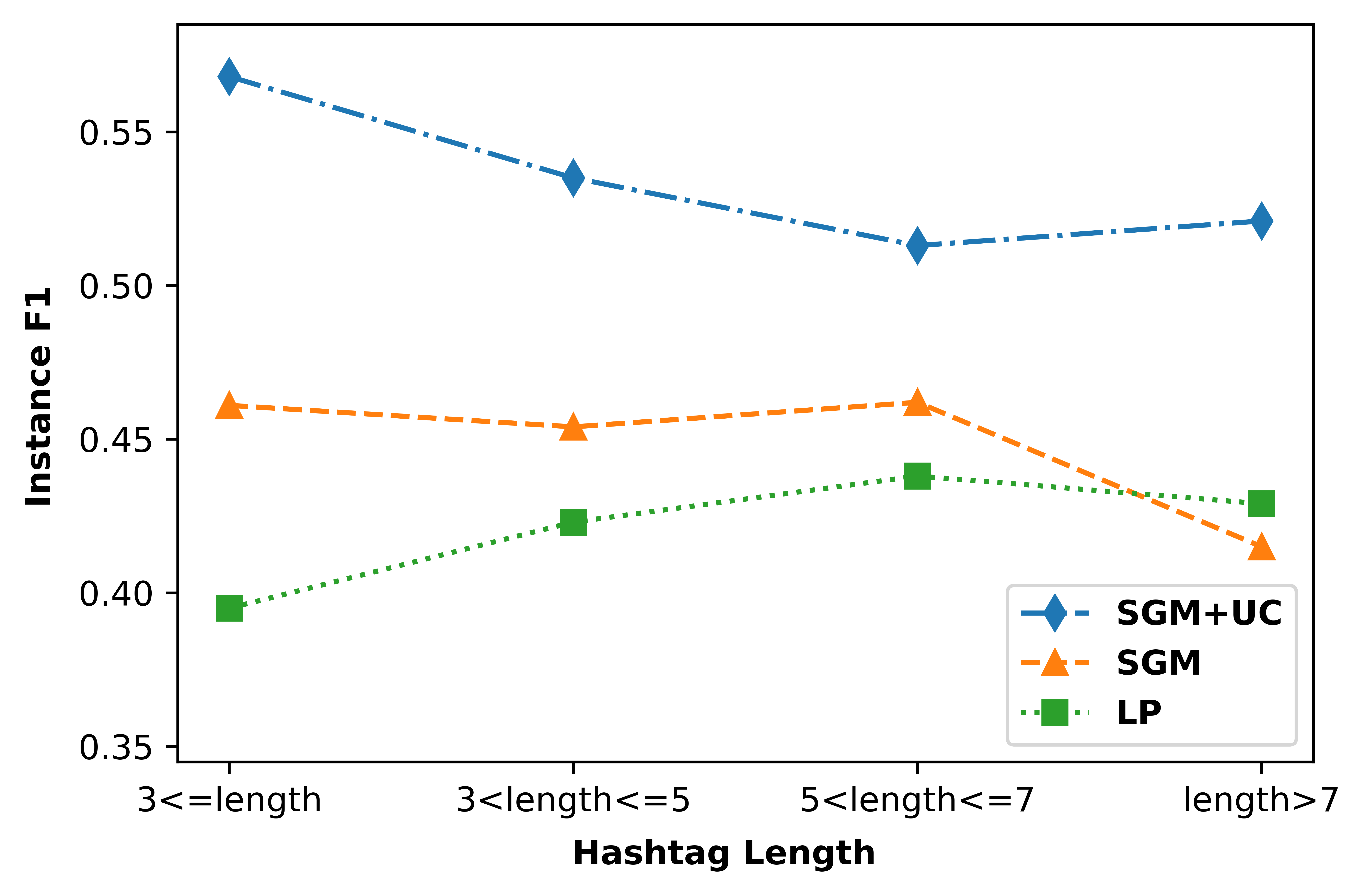