An Embedding Model for Estimating Legislative Preferences from the Frequency and Sentiment of Tweets
Gregory Spell, Brian Guay, Sunshine Hillygus, Lawrence Carin
Computational Social Science and Social Media Long Paper
You can open the pre-recorded video in a separate window.
Abstract:
Legislator preferences are typically represented as measures of general ideology estimated from roll call votes on legislation, potentially masking important nuances in legislators’ political attitudes. In this paper we introduce a method of measuring more specific legislator attitudes using an alternative expression of preferences: tweeting. Specifically, we present an embedding-based model for predicting the frequency and sentiment of legislator tweets. To illustrate our method, we model legislators’ attitudes towards President Donald Trump as vector embeddings that interact with embeddings for Trump himself constructed using a neural network from the text of his daily tweets. We demonstrate the predictive performance of our model on tweets authored by members of the U.S. House and Senate related to the president from November 2016 to February 2018. We further assess the quality of our learned representations for legislators by comparing to traditional measures of legislator preferences.
NOTE: Video may display a random order of authors.
Correct author list is at the top of this page.
Connected Papers in EMNLP2020
Similar Papers
We Can Detect Your Bias: Predicting the Political Ideology of News Articles
Ramy Baly, Giovanni Da San Martino, James Glass, Preslav Nakov,
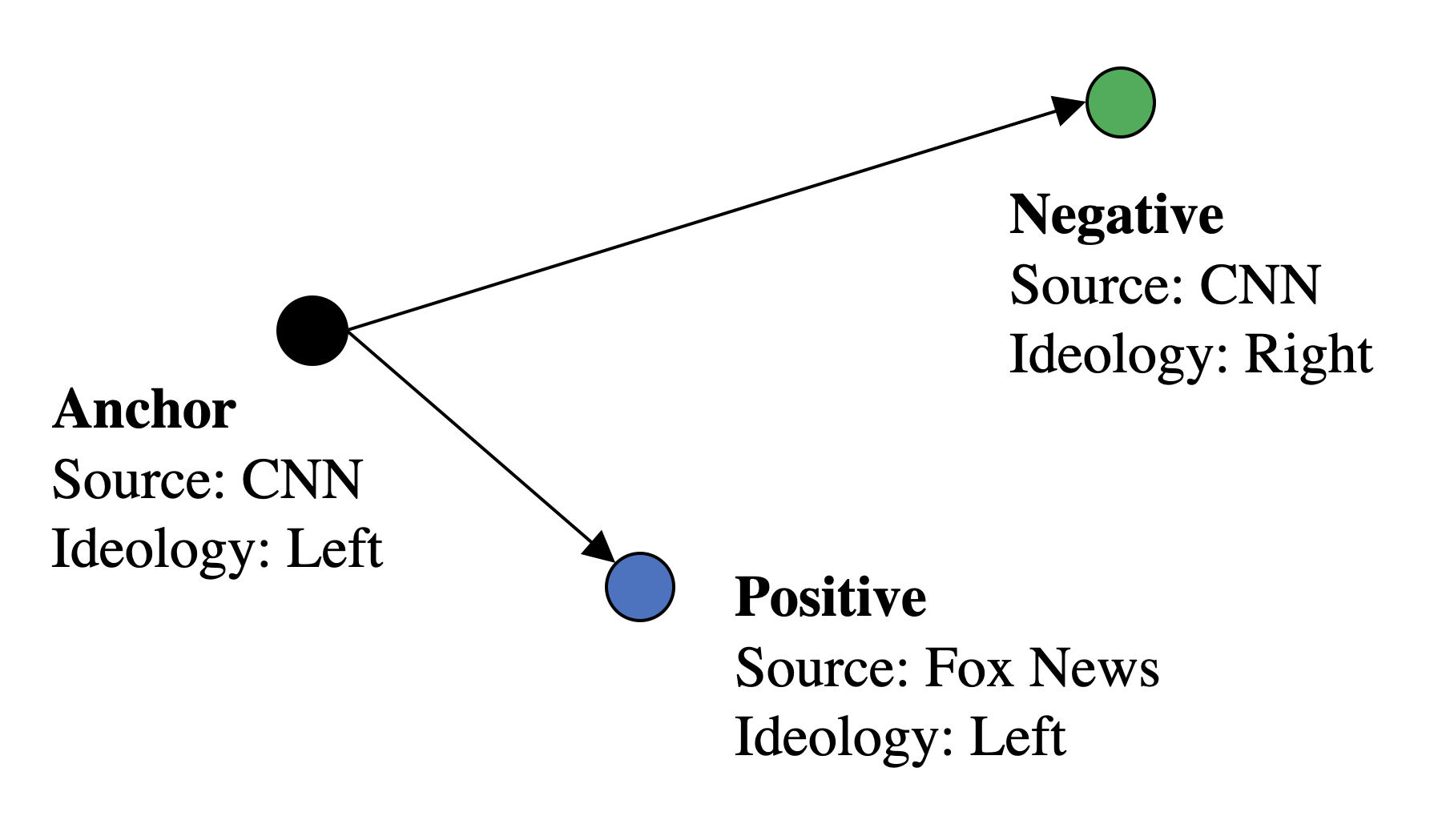
Unsupervised stance detection for arguments from consequences
Jonathan Kobbe, Ioana Hulpus, Heiner Stuckenschmidt,

Compositional Demographic Word Embeddings
Charles Welch, Jonathan K. Kummerfeld, Verónica Pérez-Rosas, Rada Mihalcea,

Weakly-Supervised Aspect-Based Sentiment Analysis via Joint Aspect-Sentiment Topic Embedding
Jiaxin Huang, Yu Meng, Fang Guo, Heng Ji, Jiawei Han,
