Fortifying Toxic Speech Detectors Against Veiled Toxicity
Xiaochuang Han, Yulia Tsvetkov
Computational Social Science and Social Media Short Paper
You can open the pre-recorded video in a separate window.
Abstract:
Modern toxic speech detectors are incompetent in recognizing disguised offensive language, such as adversarial attacks that deliberately avoid known toxic lexicons, or manifestations of implicit bias. Building a large annotated dataset for such veiled toxicity can be very expensive. In this work, we propose a framework aimed at fortifying existing toxic speech detectors without a large labeled corpus of veiled toxicity. Just a handful of probing examples are used to surface orders of magnitude more disguised offenses. We augment the toxic speech detector's training data with these discovered offensive examples, thereby making it more robust to veiled toxicity while preserving its utility in detecting overt toxicity.
NOTE: Video may display a random order of authors.
Correct author list is at the top of this page.
Connected Papers in EMNLP2020
Similar Papers
Detecting Word Sense Disambiguation Biases in Machine Translation for Model-Agnostic Adversarial Attacks
Denis Emelin, Ivan Titov, Rico Sennrich,
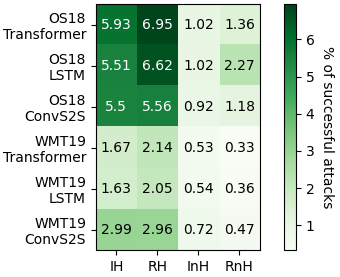
Improving Grammatical Error Correction Models with Purpose-Built Adversarial Examples
Lihao Wang, Xiaoqing Zheng,
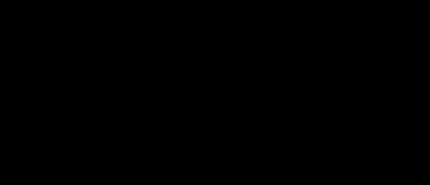
T3: Tree-Autoencoder Constrained Adversarial Text Generation for Targeted Attack
Boxin Wang, Hengzhi Pei, Boyuan Pan, Qian Chen, Shuohang Wang, Bo Li,

Detecting Cross-Modal Inconsistency to Defend Against Neural Fake News
Reuben Tan, Bryan Plummer, Kate Saenko,
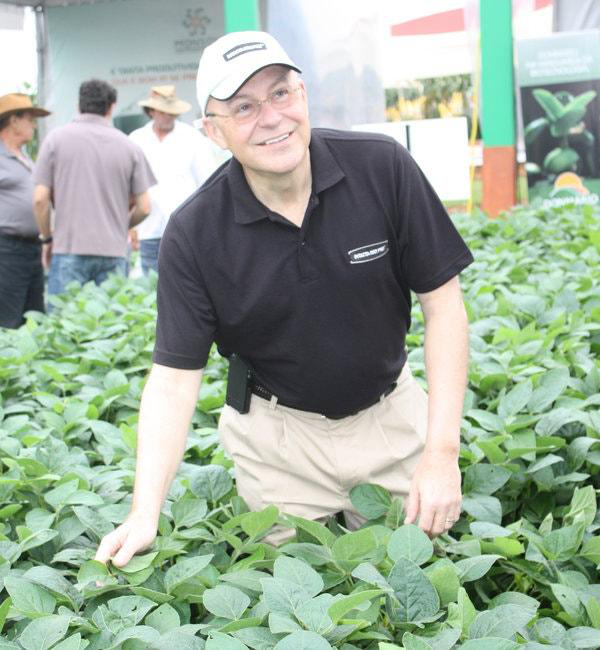