Supervised Seeded Iterated Learning for Interactive Language Learning
Yuchen Lu, Soumye Singhal, Florian Strub, Olivier Pietquin, Aaron Courville
Dialog and Interactive Systems Short Paper
You can open the pre-recorded video in a separate window.
Abstract:
Language drift has been one of the major obstacles to train language models through interaction. When word-based conversational agents are trained towards completing a task, they tend to invent their language rather than leveraging natural language. In recent literature, two general methods partially counter this phenomenon: Supervised Selfplay (S2P) and Seeded Iterated Learning (SIL). While S2P jointly trains interactive and supervised losses to counter the drift, SIL changes the training dynamics to prevent language drift from occurring. In this paper, we first highlight their respective weaknesses, i.e., late-stage training collapses and higher negative likelihood when evaluated on human corpus. Given these observations, we introduce Supervised Seeded Iterated Learning (SSIL) to combine both methods to minimize their respective weaknesses. We then show the effectiveness of \algo in the language-drift translation game.
NOTE: Video may display a random order of authors.
Correct author list is at the top of this page.
Connected Papers in EMNLP2020
Similar Papers
Zero-Shot Cross-Lingual Transfer with Meta Learning
Farhad Nooralahzadeh, Giannis Bekoulis, Johannes Bjerva, Isabelle Augenstein,
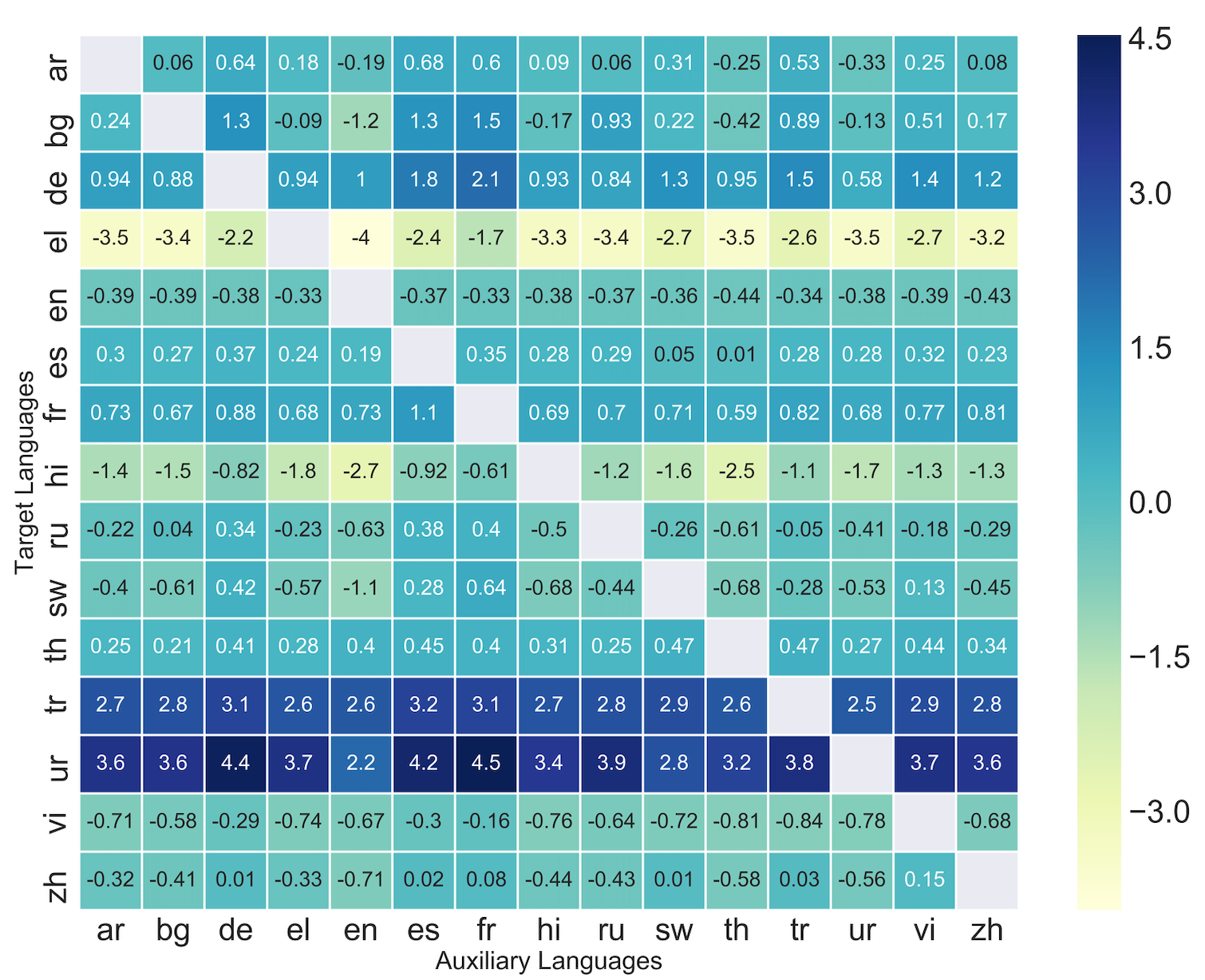
Structured Attention for Unsupervised Dialogue Structure Induction
Liang Qiu, Yizhou Zhao, Weiyan Shi, Yuan Liang, Feng Shi, Tao Yuan, Zhou Yu, Song-Chun Zhu,
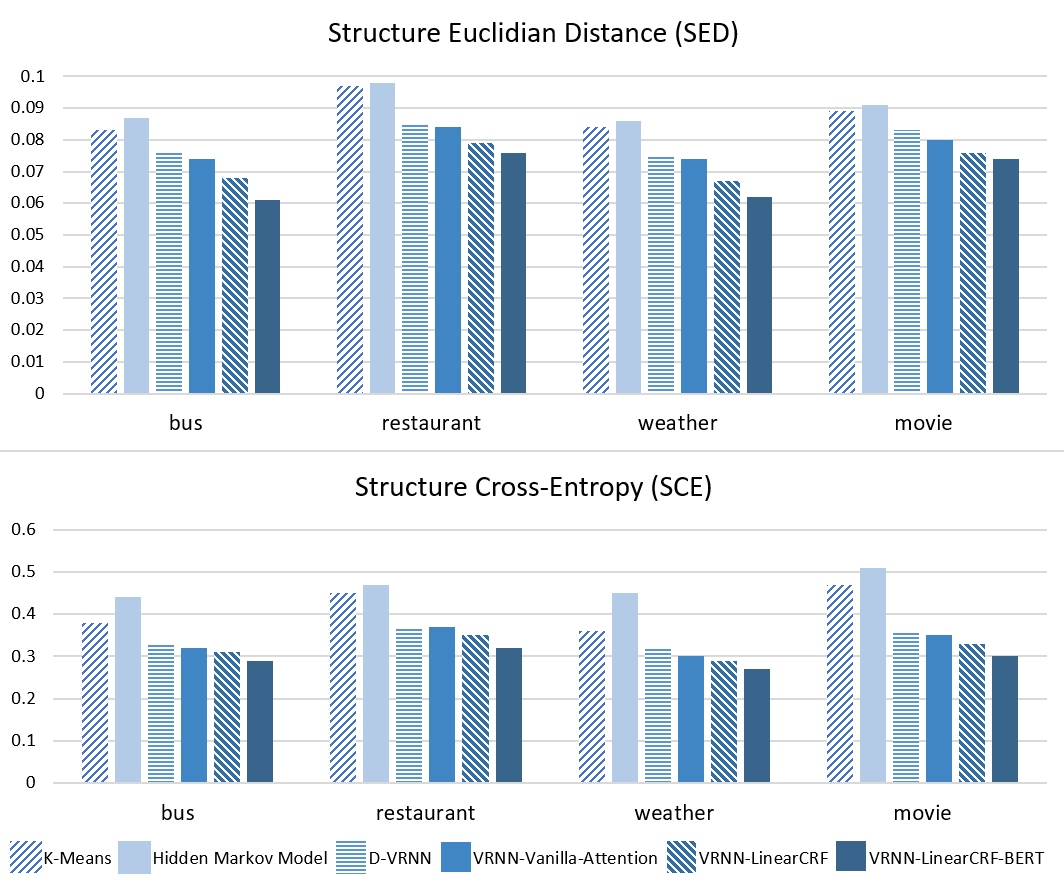
SLM: Learning a Discourse Language Representation with Sentence Unshuffling
Haejun Lee, Drew A. Hudson, Kangwook Lee, Christopher D. Manning,
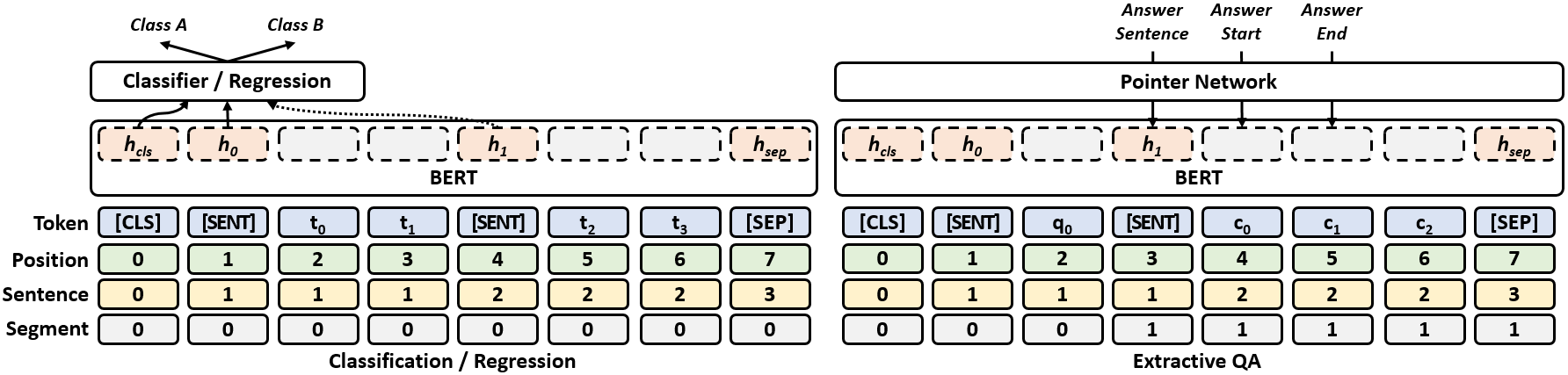
Structural Supervision Improves Few-Shot Learning and Syntactic Generalization in Neural Language Models
Ethan Wilcox, Peng Qian, Richard Futrell, Ryosuke Kohita, Roger Levy, Miguel Ballesteros,
