Hierarchical Evidence Set Modeling for Automated Fact Extraction and Verification
Shyam Subramanian, Kyumin Lee
Semantics: Sentence-level Semantics, Textual Inference and Other areas Long Paper
You can open the pre-recorded video in a separate window.
Abstract:
Automated fact extraction and verification is a challenging task that involves finding relevant evidence sentences from a reliable corpus to verify the truthfulness of a claim. Existing models either (i) concatenate all the evidence sentences, leading to the inclusion of redundant and noisy information; or (ii) process each claim-evidence sentence pair separately and aggregate all of them later, missing the early combination of related sentences for more accurate claim verification. Unlike the prior works, in this paper, we propose Hierarchical Evidence Set Modeling (HESM), a framework to extract evidence sets (each of which may contain multiple evidence sentences), and verify a claim to be supported, refuted or not enough info, by encoding and attending the claim and evidence sets at different levels of hierarchy. Our experimental results show that HESM outperforms 7 state-of-the-art methods for fact extraction and claim verification. Our source code is available at https://github.com/ShyamSubramanian/HESM.
NOTE: Video may display a random order of authors.
Correct author list is at the top of this page.
Connected Papers in EMNLP2020
Similar Papers
Fact or Fiction: Verifying Scientific Claims
David Wadden, Shanchuan Lin, Kyle Lo, Lucy Lu Wang, Madeleine van Zuylen, Arman Cohan, Hannaneh Hajishirzi,
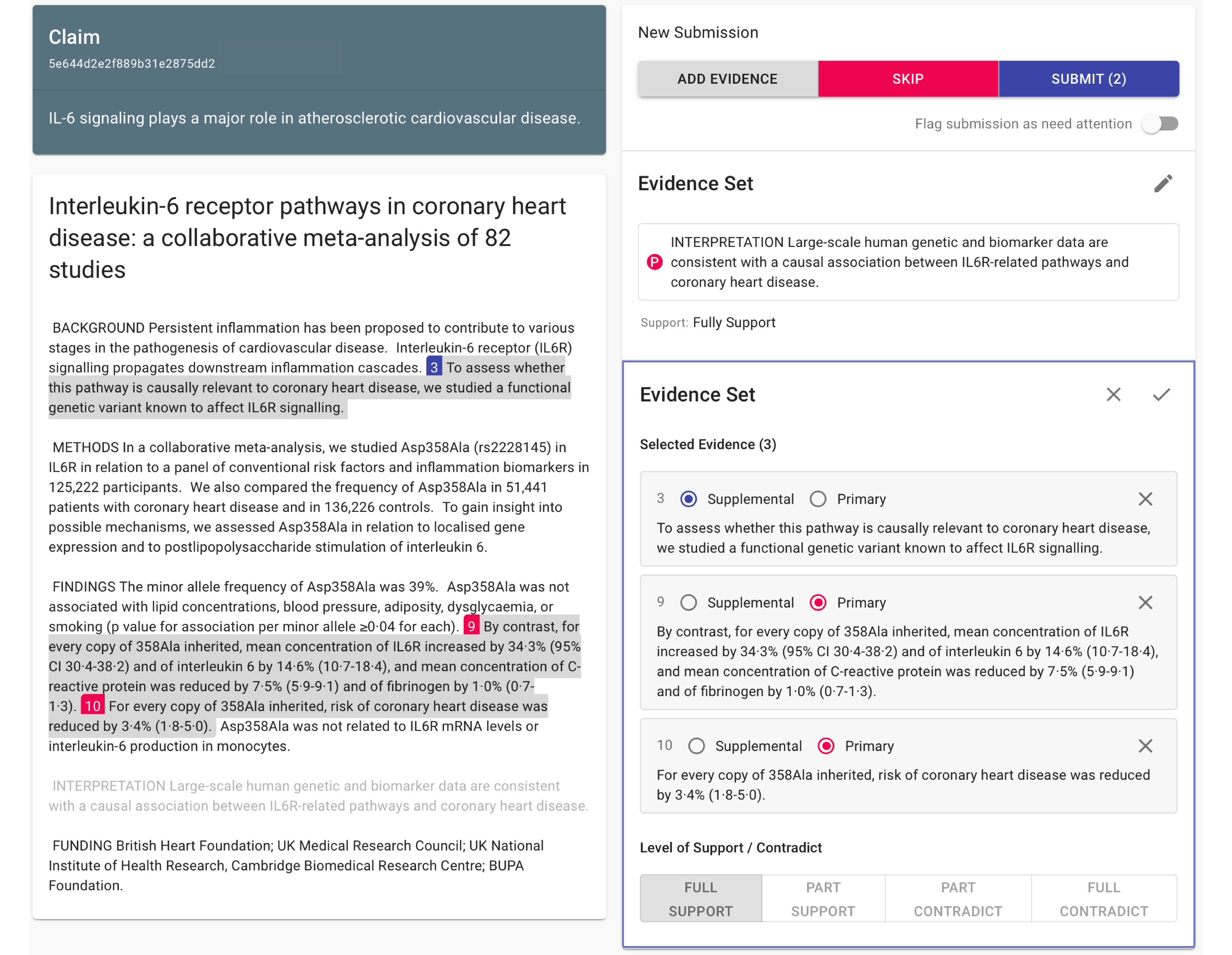
Generating Fact Checking Briefs
Angela Fan, Aleksandra Piktus, Fabio Petroni, Guillaume Wenzek, Marzieh Saeidi, Andreas Vlachos, Antoine Bordes, Sebastian Riedel,
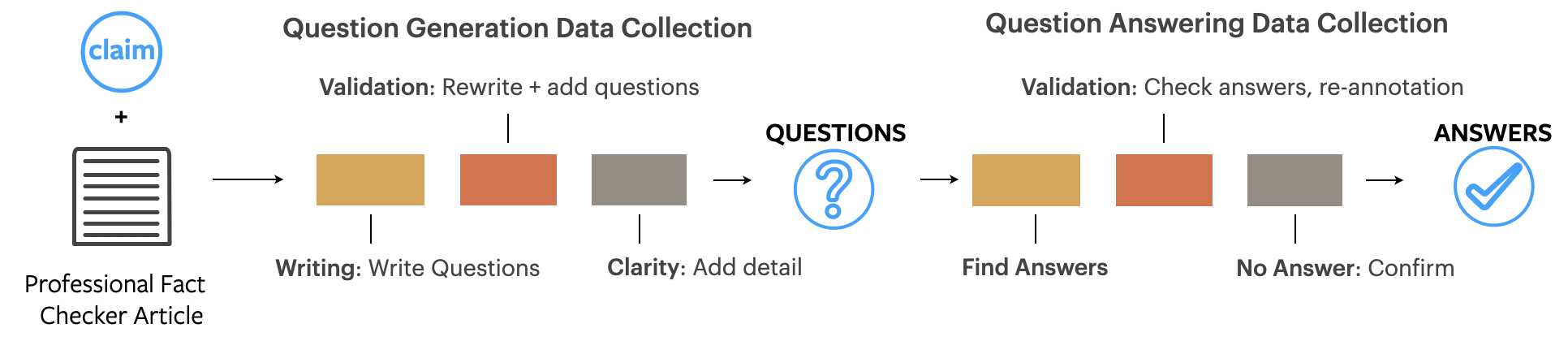
Learning from Context or Names? An Empirical Study on Neural Relation Extraction
Hao Peng, Tianyu Gao, Xu Han, Yankai Lin, Peng Li, Zhiyuan Liu, Maosong Sun, Jie Zhou,
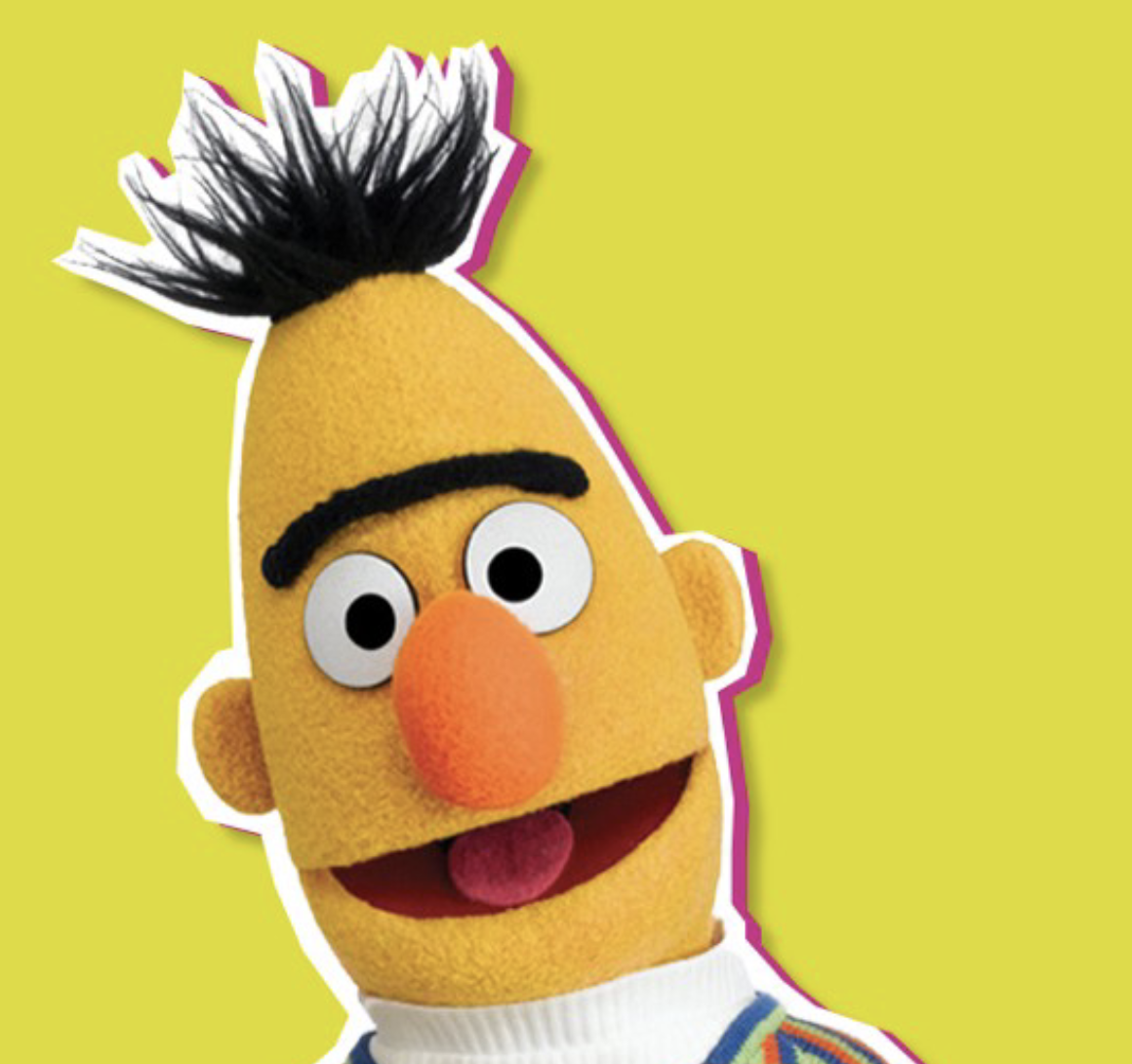