Compressive Summarization with Plausibility and Salience Modeling
Shrey Desai, Jiacheng Xu, Greg Durrett
Summarization Long Paper
You can open the pre-recorded video in a separate window.
Abstract:
Compressive summarization systems typically rely on a seed set of syntactic rules to determine under what circumstances deleting a span is permissible, then learn which compressions to actually apply by optimizing for ROUGE. In this work, we propose to relax these explicit syntactic constraints on candidate spans, and instead leave the decision about what to delete to two data-driven criteria: plausibility and salience. Deleting a span is plausible if removing it maintains the grammaticality and factuality of a sentence, and it is salient if it removes important information from the summary. Each of these is judged by a pre-trained Transformer model, and only deletions that are both plausible and not salient can be applied. When integrated into a simple extraction-compression pipeline, our method achieves strong in-domain results on benchmark datasets, and human evaluation shows that the plausibility model generally selects for grammatical and factual deletions. Furthermore, the flexibility of our approach allows it to generalize cross-domain, and we show that our system fine-tuned on only 500 samples from a new domain can match or exceed a strong in-domain extractive model.
NOTE: Video may display a random order of authors.
Correct author list is at the top of this page.
Connected Papers in EMNLP2020
Similar Papers
Syntactic Structure Distillation Pretraining for Bidirectional Encoders
Adhiguna Kuncoro, Lingpeng Kong, Daniel Fried, Dani Yogatama, Laura Rimell, Chris Dyer, Phil Blunsom,

An Information Bottleneck Approach for Controlling Conciseness in Rationale Extraction
Bhargavi Paranjape, Mandar Joshi, John Thickstun, Hannaneh Hajishirzi, Luke Zettlemoyer,
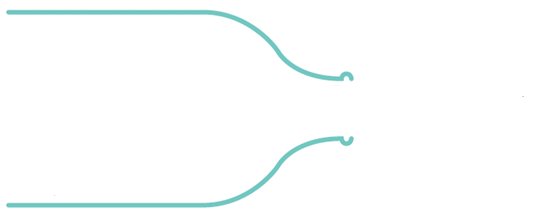
Evaluating the Factual Consistency of Abstractive Text Summarization
Wojciech Kryscinski, Bryan McCann, Caiming Xiong, Richard Socher,

Q-learning with Language Model for Edit-based Unsupervised Summarization
Ryosuke Kohita, Akifumi Wachi, Yang Zhao, Ryuki Tachibana,
