Is the Best Better? Bayesian Statistical Model Comparison for Natural Language Processing
Piotr Szymański, Kyle Gorman
Machine Learning for NLP Short Paper
You can open the pre-recorded video in a separate window.
Abstract:
Recent work raises concerns about the use of standard splits to compare natural language processing models. We propose a Bayesian statistical model comparison technique which uses k-fold cross-validation across multiple data sets to estimate the likelihood that one model will outperform the other, or that the two will produce practically equivalent results. We use this technique to rank six English part-of-speech taggers across two data sets and three evaluation metrics.
NOTE: Video may display a random order of authors.
Correct author list is at the top of this page.
Connected Papers in EMNLP2020
Similar Papers
Predicting Reference: What do Language Models Learn about Discourse Models?
Shiva Upadhye, Leon Bergen, Andrew Kehler,

Detecting Independent Pronoun Bias with Partially-Synthetic Data Generation
Robert Munro, Alex (Carmen) Morrison,
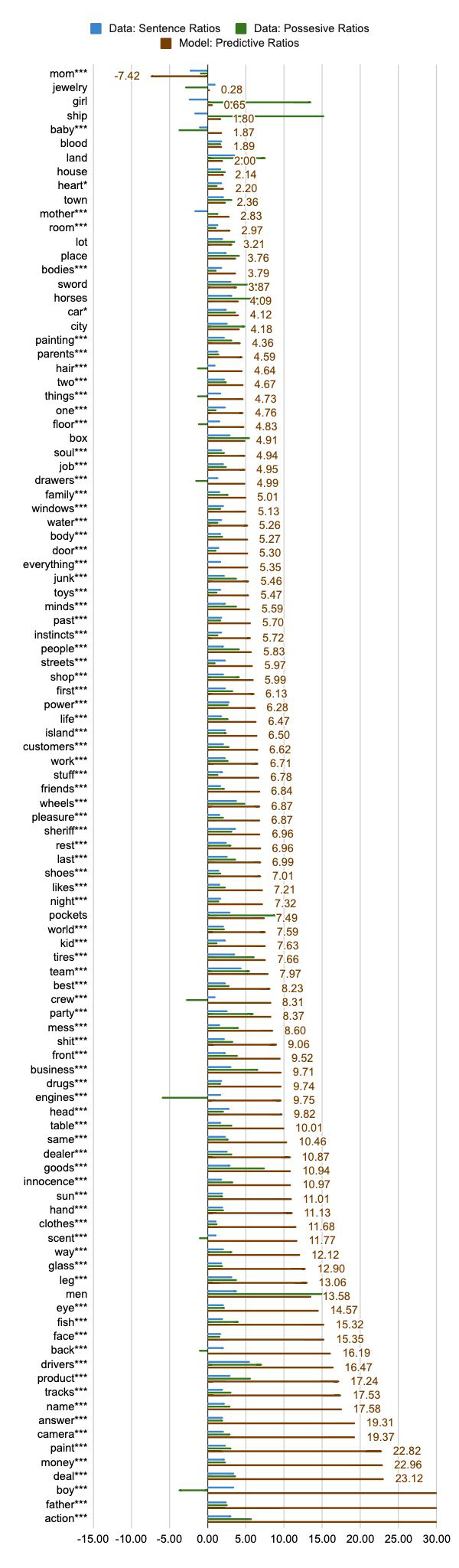
Experience Grounds Language
Yonatan Bisk, Ari Holtzman, Jesse Thomason, Jacob Andreas, Yoshua Bengio, Joyce Chai, Mirella Lapata, Angeliki Lazaridou, Jonathan May, Aleksandr Nisnevich, Nicolas Pinto, Joseph Turian,
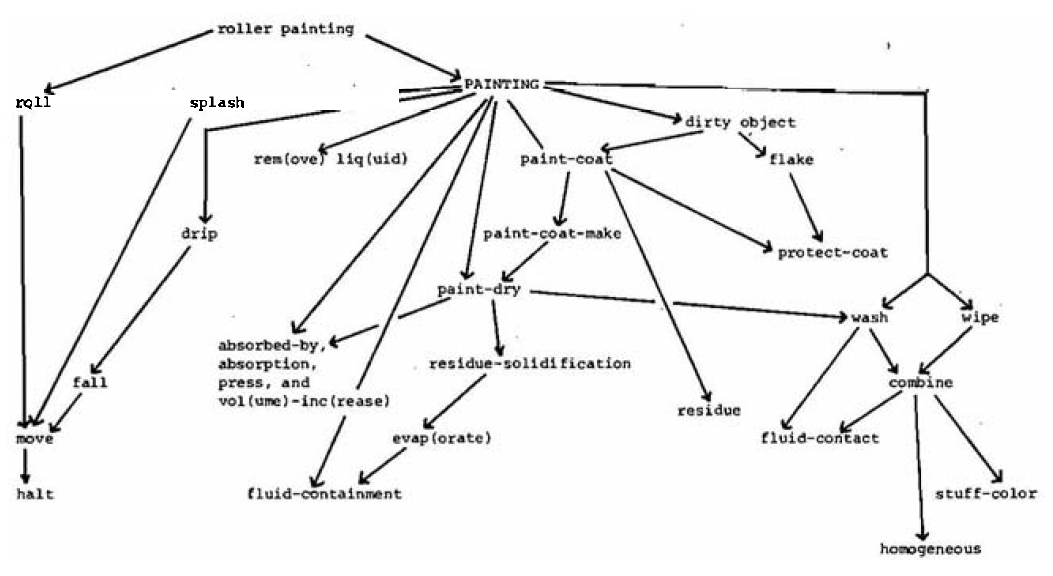
Investigating representations of verb bias in neural language models
Robert Hawkins, Takateru Yamakoshi, Thomas Griffiths, Adele Goldberg,
