Detecting Independent Pronoun Bias with Partially-Synthetic Data Generation
Robert Munro, Alex (Carmen) Morrison
Interpretability and Analysis of Models for NLP Short Paper
You can open the pre-recorded video in a separate window.
Abstract:
We report that state-of-the-art parsers consistently failed to identify “hers” and “theirs” as pronouns but identified the masculine equivalent “his”. We find that the same biases exist in recent language models like BERT. While some of the bias comes from known sources, like training data with gender imbalances, we find that the bias is _amplified_ in the language models and that linguistic differences between English pronouns that are not inherently biased can become biases in some machine learning models. We introduce a new technique for measuring bias in models, using Bayesian approximations to generate partially-synthetic data from the model itself.
NOTE: Video may display a random order of authors.
Correct author list is at the top of this page.
Connected Papers in EMNLP2020
Similar Papers
Word Frequency Does Not Predict Grammatical Knowledge in Language Models
Charles Yu, Ryan Sie, Nicolas Tedeschi, Leon Bergen,
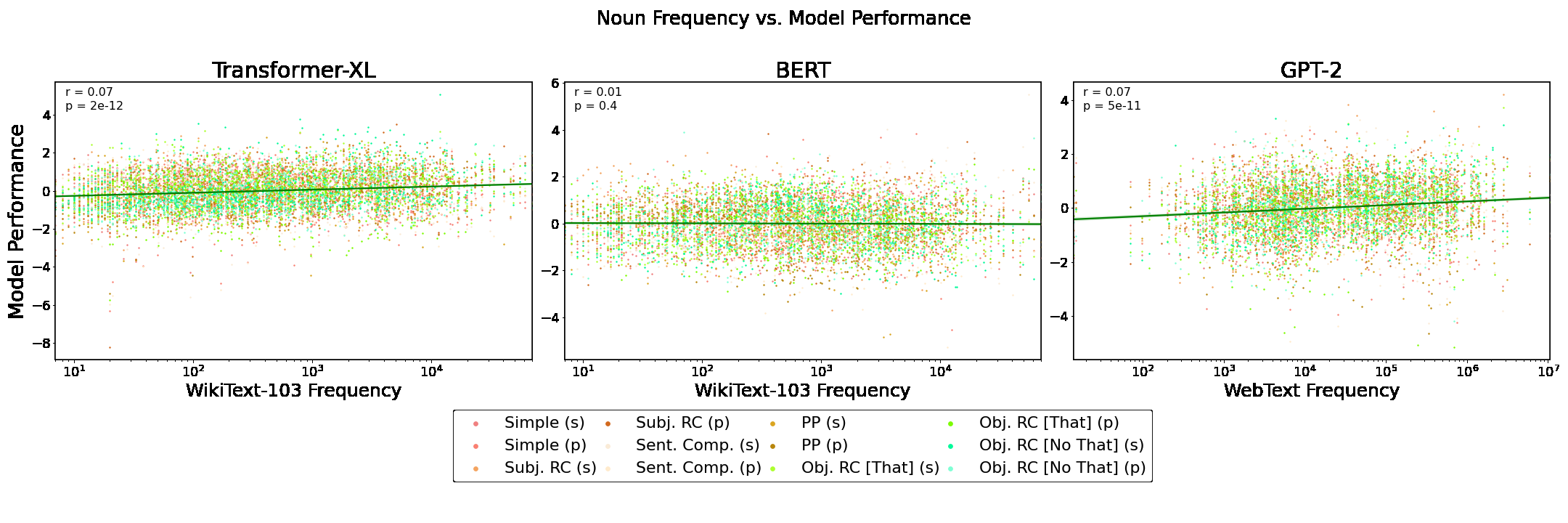
Detecting Word Sense Disambiguation Biases in Machine Translation for Model-Agnostic Adversarial Attacks
Denis Emelin, Ivan Titov, Rico Sennrich,
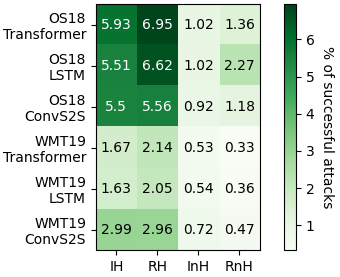
“You are grounded!”: Latent Name Artifacts in Pre-trained Language Models
Vered Shwartz, Rachel Rudinger, Oyvind Tafjord,

Investigating Cross-Linguistic Adjective Ordering Tendencies with a Latent-Variable Model
Jun Yen Leung, Guy Emerson, Ryan Cotterell,
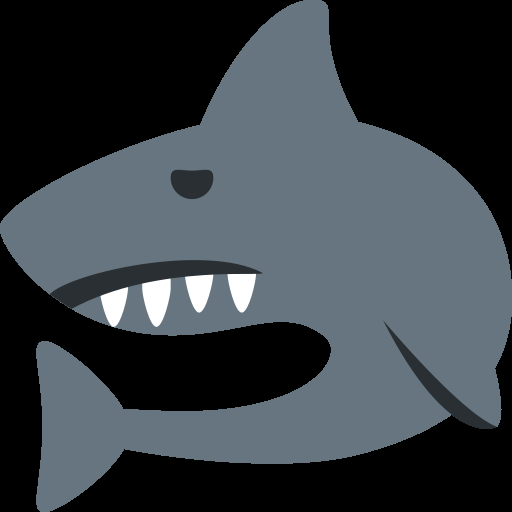