When BERT Plays the Lottery, All Tickets Are Winning
Sai Prasanna, Anna Rogers, Anna Rumshisky
Interpretability and Analysis of Models for NLP Long Paper
You can open the pre-recorded video in a separate window.
Abstract:
{L}arge {T}ransformer-based models were shown to be reducible to a smaller number of self-attention heads and layers. We consider this phenomenon from the perspective of the lottery ticket hypothesis, using both structured and magnitude pruning. For fine-tuned {BERT}, we show that (a) it is possible to find subnetworks achieving performance that is comparable with that of the full model, and (b) similarly-sized subnetworks sampled from the rest of the model perform worse. Strikingly, with structured pruning even the worst possible subnetworks remain highly trainable, indicating that most pre-trained {BERT} weights are potentially useful. We also study the ``good" subnetworks to see if their success can be attributed to superior linguistic knowledge, but find them unstable, and not explained by meaningful self-attention patterns.
NOTE: Video may display a random order of authors.
Correct author list is at the top of this page.
Connected Papers in EMNLP2020
Similar Papers
Precise Task Formalization Matters in Winograd Schema Evaluations
Haokun Liu, William Huang, Dhara Mungra, Samuel R. Bowman,

Losing Heads in the Lottery: Pruning Transformer Attention in Neural Machine Translation
Maximiliana Behnke, Kenneth Heafield,

oLMpics - On what Language Model Pre-training Captures
Alon Talmor, Yanai Elazar, Yoav Goldberg, Jonathan Berant,

The World is Not Binary: Learning to Rank with Grayscale Data for Dialogue Response Selection
Zibo Lin, Deng Cai, Yan Wang, Xiaojiang Liu, Haitao Zheng, Shuming Shi,
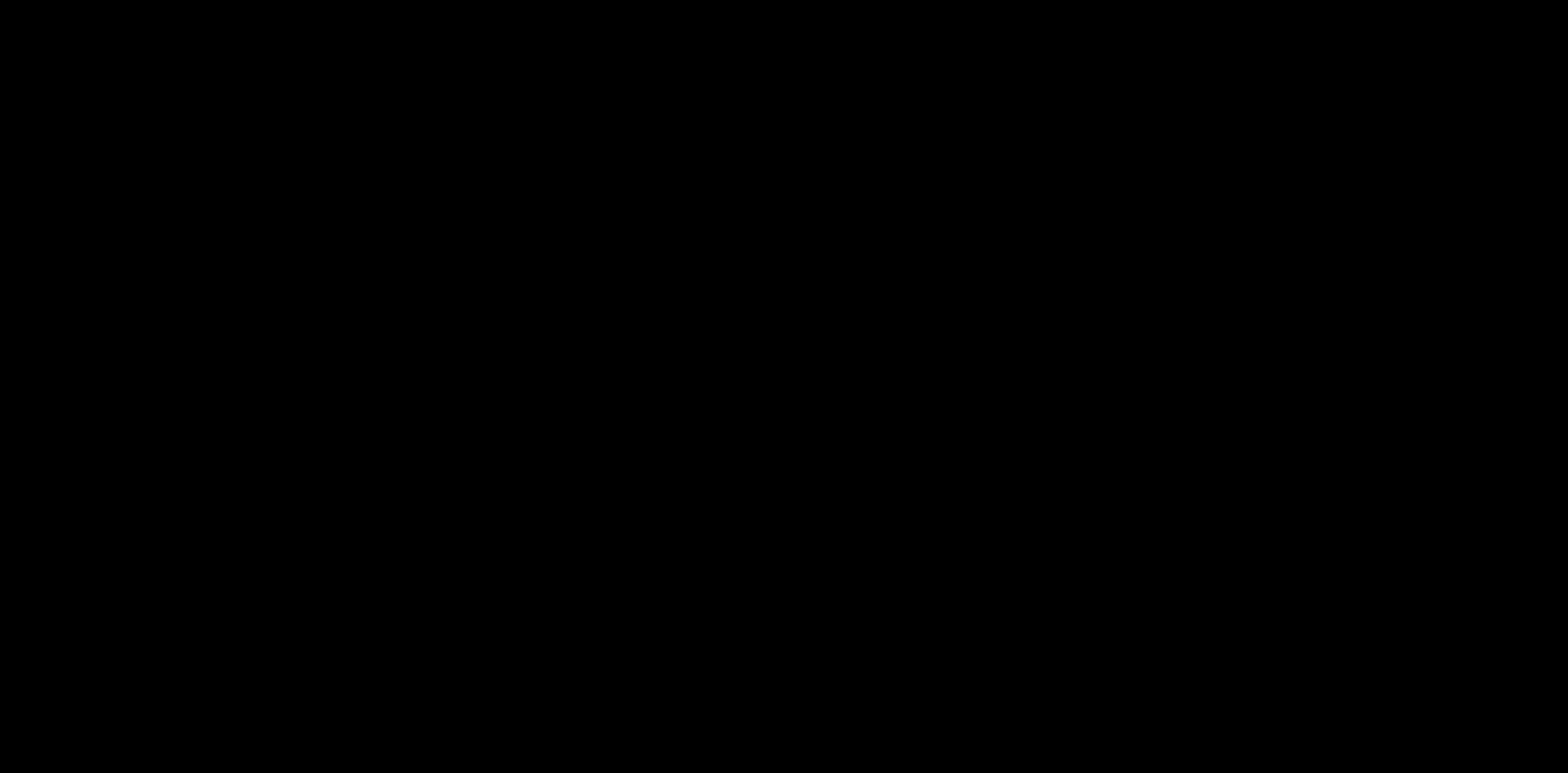