The Secret is in the Spectra: Predicting Cross-lingual Task Performance with Spectral Similarity Measures
Haim Dubossarsky, Ivan Vulić, Roi Reichart, Anna Korhonen
Machine Translation and Multilinguality Long Paper
You can open the pre-recorded video in a separate window.
Abstract:
Performance in cross-lingual NLP tasks is impacted by the (dis)similarity of languages at hand: e.g., previous work has suggested there is a connection between the expected success of bilingual lexicon induction (BLI) and the assumption of (approximate) isomorphism between monolingual embedding spaces. In this work we present a large-scale study focused on the correlations between monolingual embedding space similarity and task performance, covering thousands of language pairs and four different tasks: BLI, parsing, POS tagging and MT. We hypothesize that statistics of the spectrum of each monolingual embedding space indicate how well they can be aligned. We then introduce several isomorphism measures between two embedding spaces, based on the relevant statistics of their individual spectra. We empirically show that (1) language similarity scores derived from such spectral isomorphism measures are strongly associated with performance observed in different cross-lingual tasks, and (2) our spectral-based measures consistently outperform previous standard isomorphism measures, while being computationally more tractable and easier to interpret. Finally, our measures capture complementary information to typologically driven language distance measures, and the combination of measures from the two families yields even higher task performance correlations.
NOTE: Video may display a random order of authors.
Correct author list is at the top of this page.
Connected Papers in EMNLP2020
Similar Papers
LAReQA: Language-Agnostic Answer Retrieval from a Multilingual Pool
Uma Roy, Noah Constant, Rami Al-Rfou, Aditya Barua, Aaron Phillips, Yinfei Yang,

Probing Pretrained Language Models for Lexical Semantics
Ivan Vulić, Edoardo Maria Ponti, Robert Litschko, Goran Glavaš, Anna Korhonen,
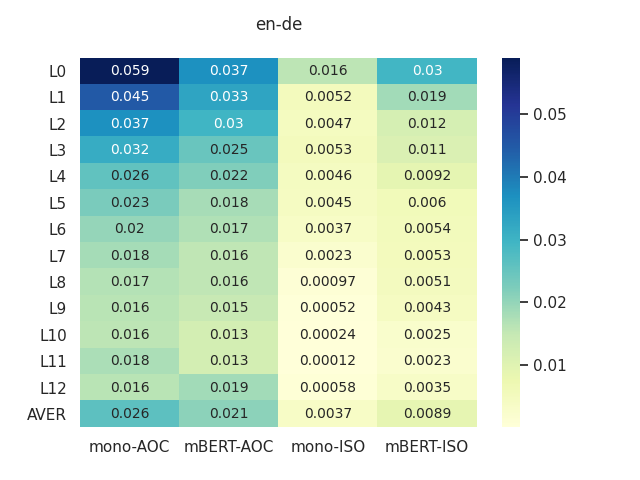