Personal Information Leakage Detection in Conversations
Qiongkai Xu, Lizhen Qu, Zeyu Gao, Gholamreza Haffari
Dialog and Interactive Systems Long Paper
You can open the pre-recorded video in a separate window.
Abstract:
The global market size of conversational assistants (chatbots) is expected to grow to USD 9.4 billion by 2024, according to MarketsandMarkets. Despite the wide use of chatbots, leakage of personal information through chatbots poses serious privacy concerns for their users. In this work, we propose to protect personal information by warning users of detected suspicious sentences generated by conversational assistants. The detection task is formulated as an alignment optimization problem and a new dataset PERSONA-LEAKAGE is collected for evaluation. In this paper, we propose two novel constrained alignment models, which consistently outperform baseline methods on Moreover, we conduct analysis on the behavior of recently proposed personalized chit-chat dialogue systems. The empirical results show that those systems suffer more from personal information disclosure than the widely used Seq2Seq model and the language model. In those cases, a significant number of information leaking utterances can be detected by our models with high precision.
NOTE: Video may display a random order of authors.
Correct author list is at the top of this page.
Connected Papers in EMNLP2020
Similar Papers
Spot The Bot: A Robust and Efficient Framework for the Evaluation of Conversational Dialogue Systems
Jan Deriu, Don Tuggener, Pius von Däniken, Jon Ander Campos, Alvaro Rodrigo, Thiziri Belkacem, Aitor Soroa, Eneko Agirre, Mark Cieliebak,
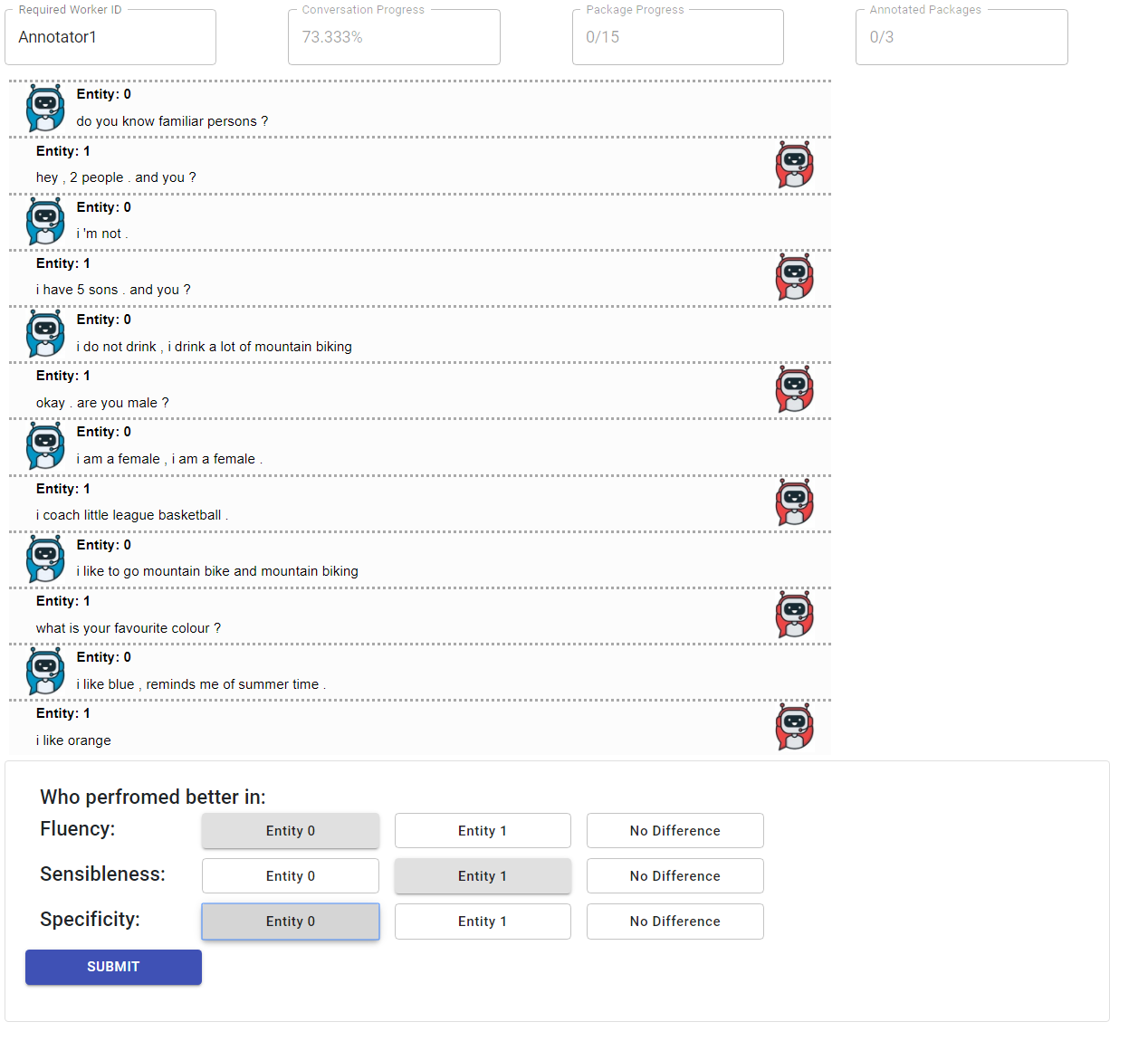
MedFilter: Improving Extraction of Task-relevant Utterances through Integration of Discourse Structure and Ontological Knowledge
Sopan Khosla, Shikhar Vashishth, Jill Fain Lehman, Carolyn Rose,
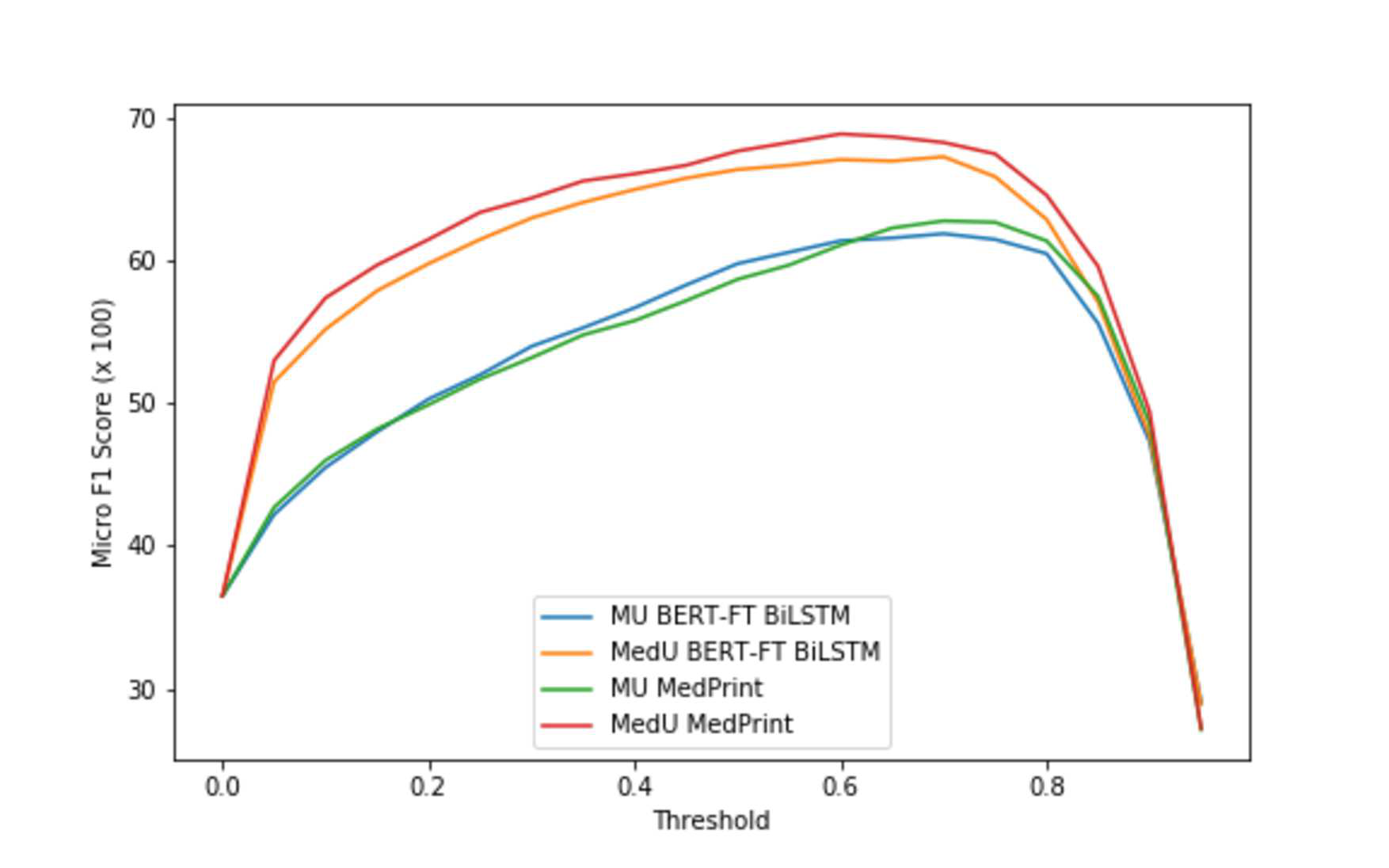
Queens are Powerful too: Mitigating Gender Bias in Dialogue Generation
Emily Dinan, Angela Fan, Adina Williams, Jack Urbanek, Douwe Kiela, Jason Weston,
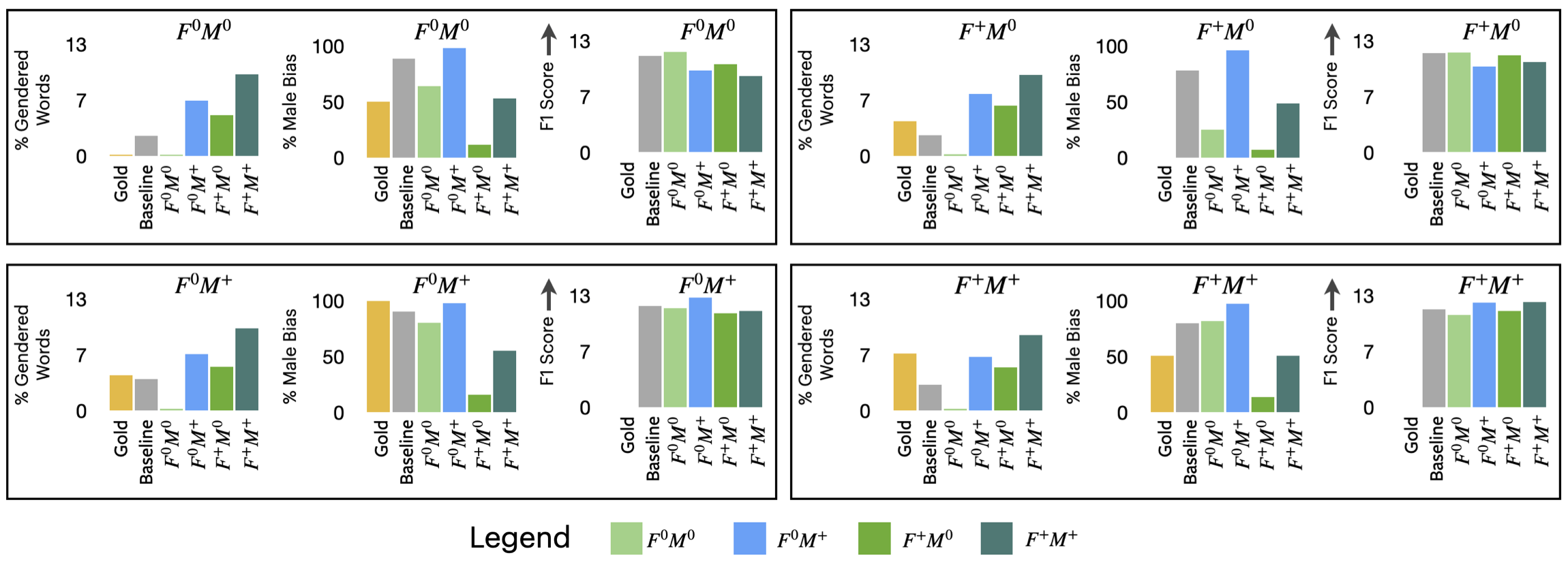