De-Biased Court's View Generation with Causality
Yiquan Wu, Kun Kuang, Yating Zhang, Xiaozhong Liu, Changlong Sun, Jun Xiao, Yueting Zhuang, Luo Si, Fei Wu
Language Generation Long Paper
You can open the pre-recorded video in a separate window.
Abstract:
Court's view generation is a novel but essential task for legal AI, aiming at improving the interpretability of judgment prediction results and enabling automatic legal document generation. While prior text-to-text natural language generation (NLG) approaches can be used to address this problem, neglecting the confounding bias from the data generation mechanism can limit the model performance, and the bias may pollute the learning outcomes. In this paper, we propose a novel Attentional and Counterfactual based Natural Language Generation (AC-NLG) method, consisting of an attentional encoder and a pair of innovative counterfactual decoders. The attentional encoder leverages the plaintiff's claim and fact description as input to learn a claim-aware encoder from which the claim-related information in fact description can be emphasized. The counterfactual decoders are employed to eliminate the confounding bias in data and generate judgment-discriminative court's views (both supportive and non-supportive views) by incorporating with a synergistic judgment predictive model. Comprehensive experiments show the effectiveness of our method under both quantitative and qualitative evaluation metrics.
NOTE: Video may display a random order of authors.
Correct author list is at the top of this page.
Connected Papers in EMNLP2020
Similar Papers
Fact or Fiction: Verifying Scientific Claims
David Wadden, Shanchuan Lin, Kyle Lo, Lucy Lu Wang, Madeleine van Zuylen, Arman Cohan, Hannaneh Hajishirzi,
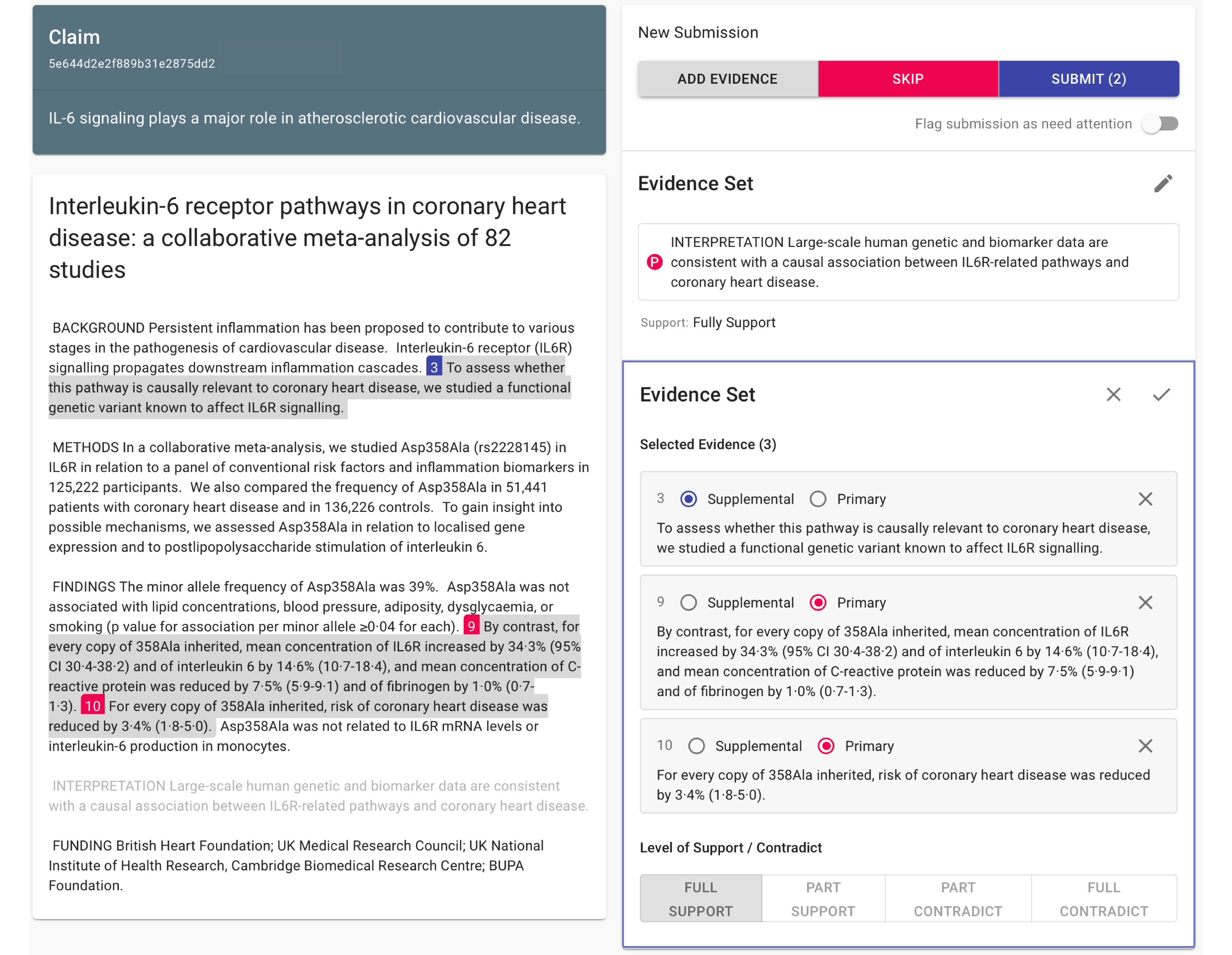
Counterfactual Generator: A Weakly-Supervised Method for Named Entity Recognition
Xiangji Zeng, Yunliang Li, Yuchen Zhai, Yin Zhang,
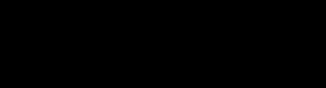
Exploring Logically Dependent Multi-task Learning with Causal Inference
Wenqing Chen, Jidong Tian, Liqiang Xiao, Hao He, Yaohui Jin,
