Character-level Representations Improve DRS-based Semantic Parsing Even in the Age of BERT
Rik van Noord, Antonio Toral, Johan Bos
Semantics: Sentence-level Semantics, Textual Inference and Other areas Long Paper
You can open the pre-recorded video in a separate window.
Abstract:
We combine character-level and contextual language model representations to improve performance on Discourse Representation Structure parsing. Character representations can easily be added in a sequence-to-sequence model in either one encoder or as a fully separate encoder, with improvements that are robust to different language models, languages and data sets. For English, these improvements are larger than adding individual sources of linguistic information or adding non-contextual embeddings. A new method of analysis based on semantic tags demonstrates that the character-level representations improve performance across a subset of selected semantic phenomena.
NOTE: Video may display a random order of authors.
Correct author list is at the top of this page.
Connected Papers in EMNLP2020
Similar Papers
Multitask Learning for Cross-Lingual Transfer of Broad-coverage Semantic Dependencies
Maryam Aminian, Mohammad Sadegh Rasooli, Mona Diab,

Uncertainty-Aware Semantic Augmentation for Neural Machine Translation
Xiangpeng Wei, Heng Yu, Yue Hu, Rongxiang Weng, Luxi Xing, Weihua Luo,
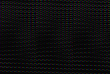
Coreferential Reasoning Learning for Language Representation
Deming Ye, Yankai Lin, Jiaju Du, Zhenghao Liu, Peng Li, Maosong Sun, Zhiyuan Liu,
