Exploring and Evaluating Attributes, Values, and Structures for Entity Alignment
Zhiyuan Liu, Yixin Cao, Liangming Pan, Juanzi Li, Zhiyuan Liu, Tat-Seng Chua
Information Extraction Long Paper
You can open the pre-recorded video in a separate window.
Abstract:
Entity alignment (EA) aims at building a unified Knowledge Graph (KG) of rich content by linking the equivalent entities from various KGs. GNN-based EA methods present promising performance by modeling the KG structure defined by relation triples. However, attribute triples can also provide crucial alignment signal but have not been well explored yet. In this paper, we propose to utilize an attributed value encoder and partition the KG into subgraphs to model the various types of attribute triples efficiently. Besides, the performances of current EA methods are overestimated because of the name-bias of existing EA datasets. To make an objective evaluation, we propose a hard experimental setting where we select equivalent entity pairs with very different names as the test set. Under both the regular and hard settings, our method achieves significant improvements (5.10% on average Hits@1 in DBP15k) over 12 baselines in cross-lingual and monolingual datasets. Ablation studies on different subgraphs and a case study about attribute types further demonstrate the effectiveness of our method. Source code and data can be found at \url{https://github.com/thunlp/explore-and-evaluate}.
NOTE: Video may display a random order of authors.
Correct author list is at the top of this page.
Connected Papers in EMNLP2020
Similar Papers
Message Passing for Hyper-Relational Knowledge Graphs
Mikhail Galkin, Priyansh Trivedi, Gaurav Maheshwari, Ricardo Usbeck, Jens Lehmann,
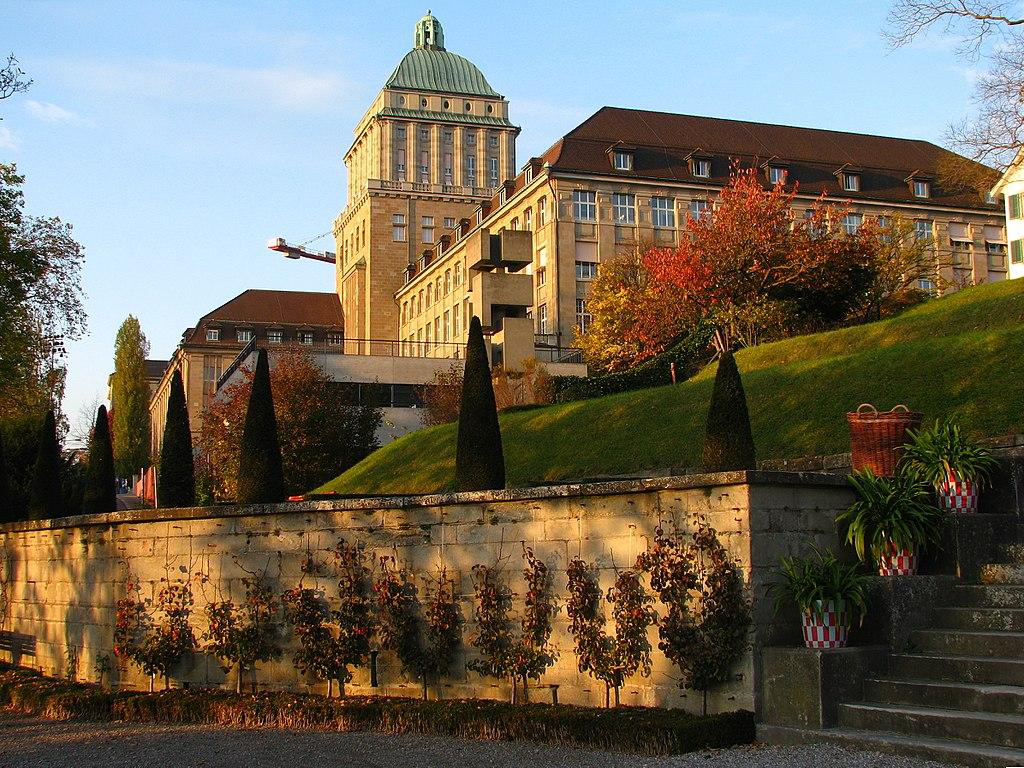
Scalable Zero-shot Entity Linking with Dense Entity Retrieval
Ledell Wu, Fabio Petroni, Martin Josifoski, Sebastian Riedel, Luke Zettlemoyer,
