Domain Adaptation of Thai Word Segmentation Models using Stacked Ensemble
Peerat Limkonchotiwat, Wannaphong Phatthiyaphaibun, Raheem Sarwar, Ekapol Chuangsuwanich, Sarana Nutanong
Phonology, Morphology and Word Segmentation Short Paper
Abstract:
Like many Natural Language Processing tasks, Thai word segmentation is domain-dependent. Researchers have been relying on transfer learning to adapt an existing model to a new domain. However, this approach is inapplicable to cases where we can interact with only input and output layers of the models, also known as "black boxes''. We propose a filter-and-refine solution based on the stacked-ensemble learning paradigm to address this black-box limitation. We conducted extensive experimental studies comparing our method against state-of-the-art models and transfer learning. Experimental results show that our proposed solution is an effective domain adaptation method and has a similar performance as the transfer learning method.
Connected Papers in EMNLP2020
Similar Papers
PERL: Pivot-based Domain Adaptation for Pre-trained Deep Contextualized Embedding Models
Roi Reichart, Eyal Ben David, Carmel Rabinovitz,

Improving Text Generation with Student-Forcing Optimal Transport
Jianqiao Li, Chunyuan Li, Guoyin Wang, Hao Fu, Yuhchen Lin, Liqun Chen, Yizhe Zhang, Chenyang Tao, Ruiyi Zhang, Wenlin Wang, Dinghan Shen, Qian Yang, Lawrence Carin,
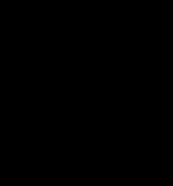
Multi-Stage Pre-training for Low-Resource Domain Adaptation
Rong Zhang, Revanth Gangi Reddy, Md Arafat Sultan, Vittorio Castelli, Anthony Ferritto, Radu Florian, Efsun Sarioglu Kayi, Salim Roukos, Avi Sil, Todd Ward,

A Joint Multiple Criteria Model in Transfer Learning for Cross-domain Chinese Word Segmentation
Kaiyu Huang, Degen Huang, Zhuang Liu, Fengran Mo,
