Planning and Generating Natural and Diverse Disfluent Texts as Augmentation for Disfluency Detection
Jingfeng Yang, Diyi Yang, Zhaoran Ma
NLP Applications Long Paper
You can open the pre-recorded video in a separate window.
Abstract:
Existing approaches to disfluency detection heavily depend on human-annotated data. Numbers of data augmentation methods have been proposed to alleviate the dependence on labeled data. However, current augmentation approaches such as random insertion or repetition fail to resemble training corpus well and usually resulted in unnatural and limited types of disfluencies. In this work, we propose a simple Planner-Generator based disfluency generation model to generate natural and diverse disfluent texts as augmented data, where the Planner decides on where to insert disfluent segments and the Generator follows the prediction to generate corresponding disfluent segments. We further utilize this augmented data for pretraining and leverage it for the task of disfluency detection. Experiments demonstrated that our two-stage disfluency generation model outperforms existing baselines; those disfluent sentences generated significantly aided the task of disfluency detection and led to state-of-the-art performance on Switchboard corpus.
NOTE: Video may display a random order of authors.
Correct author list is at the top of this page.
Connected Papers in EMNLP2020
Similar Papers
ToTTo: A Controlled Table-To-Text Generation Dataset
Ankur Parikh, Xuezhi Wang, Sebastian Gehrmann, Manaal Faruqui, Bhuwan Dhingra, Diyi Yang, Dipanjan Das,
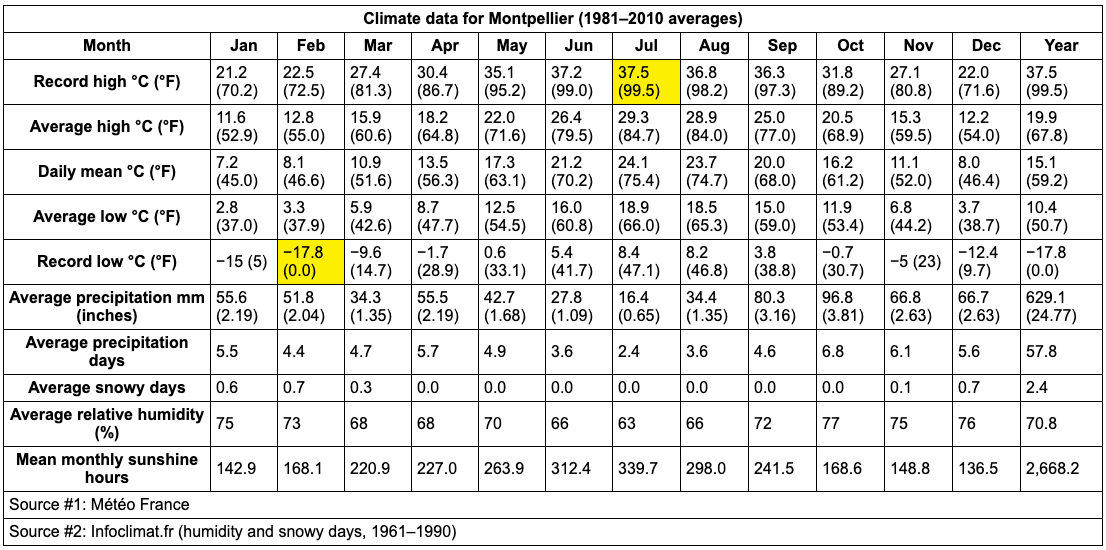