Dynamic Context Selection for Document-level Neural Machine Translation via Reinforcement Learning
Xiaomian Kang, Yang Zhao, Jiajun Zhang, Chengqing Zong
Machine Translation and Multilinguality Long Paper
You can open the pre-recorded video in a separate window.
Abstract:
Document-level neural machine translation has yielded attractive improvements. However, majority of existing methods roughly use all context sentences in a fixed scope. They neglect the fact that different source sentences need different sizes of context. To address this problem, we propose an effective approach to select dynamic context so that the document-level translation model can utilize the more useful selected context sentences to produce better translations. Specifically, we introduce a selection module that is independent of the translation module to score each candidate context sentence. Then, we propose two strategies to explicitly select a variable number of context sentences and feed them into the translation module. We train the two modules end-to-end via reinforcement learning. A novel reward is proposed to encourage the selection and utilization of dynamic context sentences. Experiments demonstrate that our approach can select adaptive context sentences for different source sentences, and significantly improves the performance of document-level translation methods.
NOTE: Video may display a random order of authors.
Correct author list is at the top of this page.
Connected Papers in EMNLP2020
Similar Papers
Pronoun-Targeted Fine-tuning for NMT with Hybrid Losses
Prathyusha Jwalapuram, Shafiq Joty, Youlin Shen,

CSP:Code-Switching Pre-training for Neural Machine Translation
Zhen Yang, Bojie Hu, Ambyera Han, Shen Huang, Qi Ju,

Token-level Adaptive Training for Neural Machine Translation
Shuhao Gu, Jinchao Zhang, Fandong Meng, Yang Feng, Wanying Xie, Jie Zhou, Dong Yu,
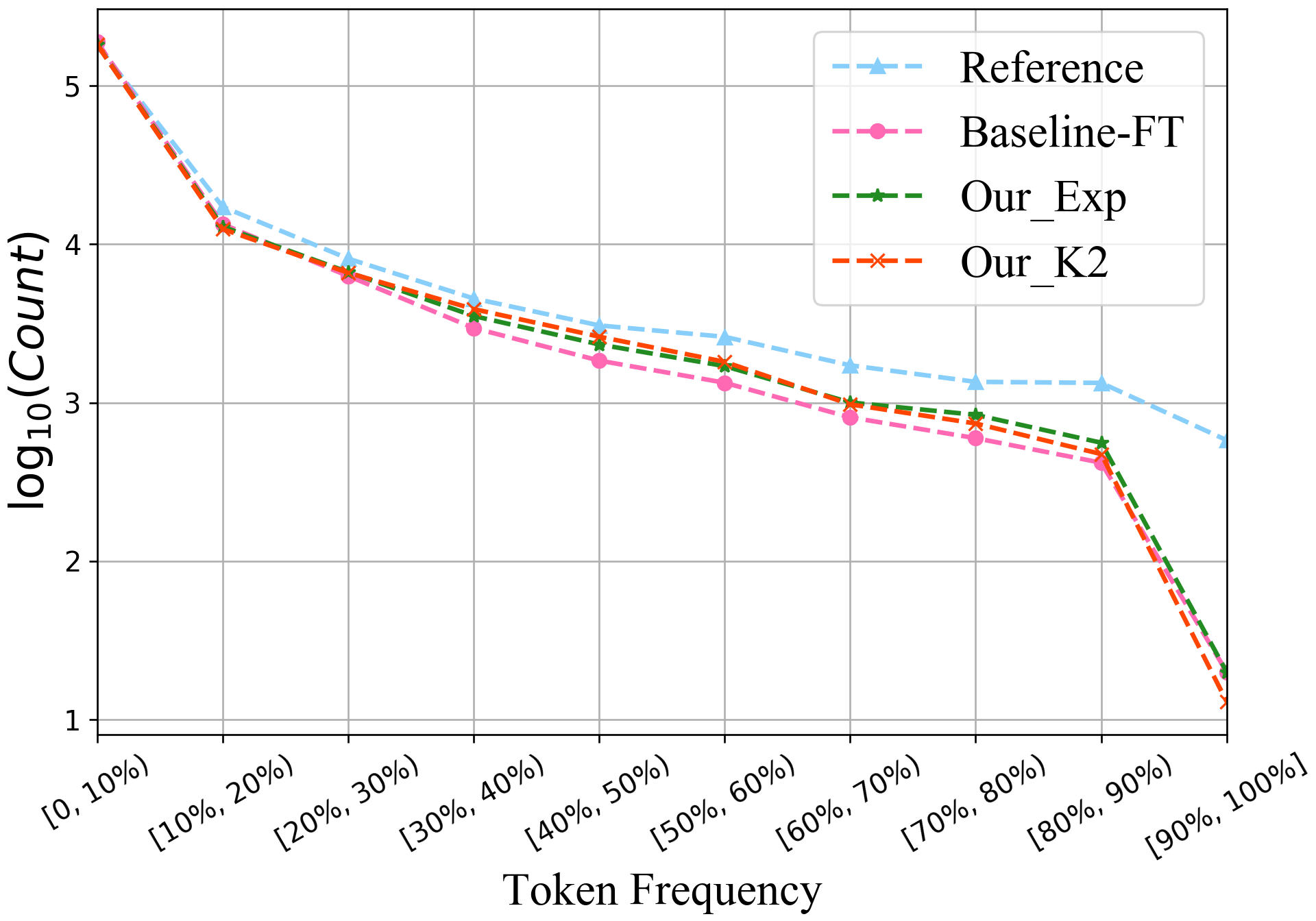
Q-learning with Language Model for Edit-based Unsupervised Summarization
Ryosuke Kohita, Akifumi Wachi, Yang Zhao, Ryuki Tachibana,
