SentiLARE: Sentiment-Aware Language Representation Learning with Linguistic Knowledge
Pei Ke, Haozhe Ji, Siyang Liu, Xiaoyan Zhu, Minlie Huang
Sentiment Analysis, Stylistic Analysis, and Argument Mining Long Paper
You can open the pre-recorded video in a separate window.
Abstract:
Most of the existing pre-trained language representation models neglect to consider the linguistic knowledge of texts, which can promote language understanding in NLP tasks. To benefit the downstream tasks in sentiment analysis, we propose a novel language representation model called SentiLARE, which introduces word-level linguistic knowledge including part-of-speech tag and sentiment polarity (inferred from SentiWordNet) into pre-trained models. We first propose a context-aware sentiment attention mechanism to acquire the sentiment polarity of each word with its part-of-speech tag by querying SentiWordNet. Then, we devise a new pre-training task called label-aware masked language model to construct knowledge-aware language representation. Experiments show that SentiLARE obtains new state-of-the-art performance on a variety of sentiment analysis tasks.
NOTE: Video may display a random order of authors.
Correct author list is at the top of this page.
Connected Papers in EMNLP2020
Similar Papers
Weakly-Supervised Aspect-Based Sentiment Analysis via Joint Aspect-Sentiment Topic Embedding
Jiaxin Huang, Yu Meng, Fang Guo, Heng Ji, Jiawei Han,

Inducing Target-Specific Latent Structures for Aspect Sentiment Classification
Chenhua Chen, Zhiyang Teng, Yue Zhang,
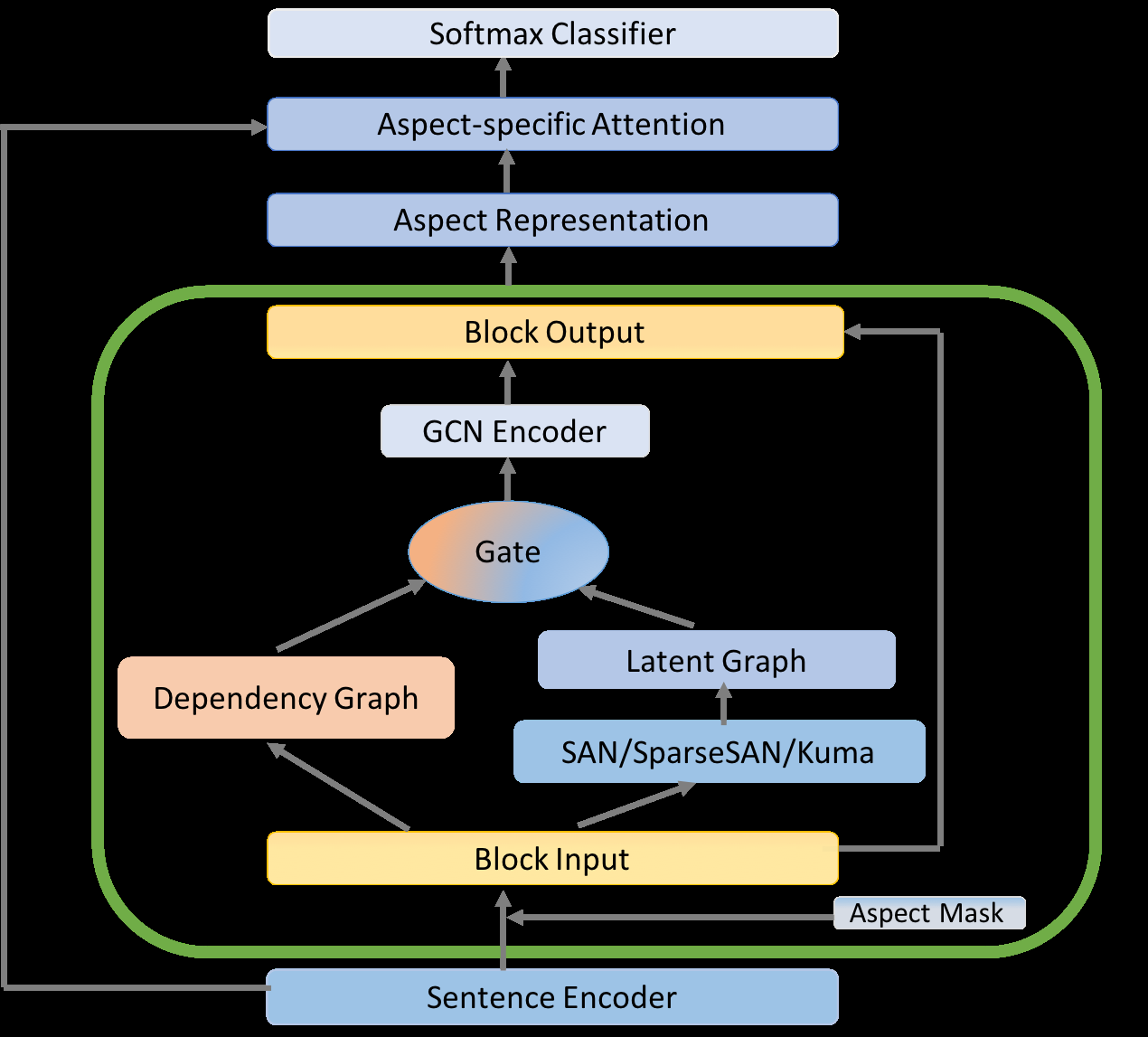
Modeling Content Importance for Summarization with Pre-trained Language Models
Liqiang Xiao, Lu Wang, Hao He, Yaohui Jin,
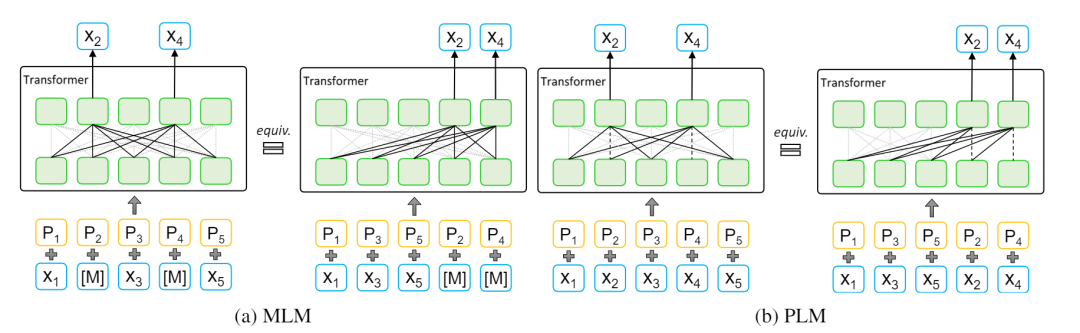