SelfORE: Self-supervised Relational Feature Learning for Open Relation Extraction
Xuming Hu, Lijie Wen, Yusong Xu, Chenwei Zhang, Philip Yu
Information Extraction Long Paper
You can open the pre-recorded video in a separate window.
Abstract:
Open relation extraction is the task of extracting open-domain relation facts from natural language sentences. Existing works either utilize heuristics or distant-supervised annotations to train a supervised classifier over pre-defined relations, or adopt unsupervised methods with additional assumptions that have less discriminative power. In this work, we propose a self-supervised framework named SelfORE, which exploits weak, self-supervised signals by leveraging large pretrained language model for adaptive clustering on contextualized relational features, and bootstraps the self-supervised signals by improving contextualized features in relation classification. Experimental results on three datasets show the effectiveness and robustness of SelfORE on open-domain Relation Extraction when comparing with competitive baselines.
NOTE: Video may display a random order of authors.
Correct author list is at the top of this page.
Connected Papers in EMNLP2020
Similar Papers
Learning from Context or Names? An Empirical Study on Neural Relation Extraction
Hao Peng, Tianyu Gao, Xu Han, Yankai Lin, Peng Li, Zhiyuan Liu, Maosong Sun, Jie Zhou,
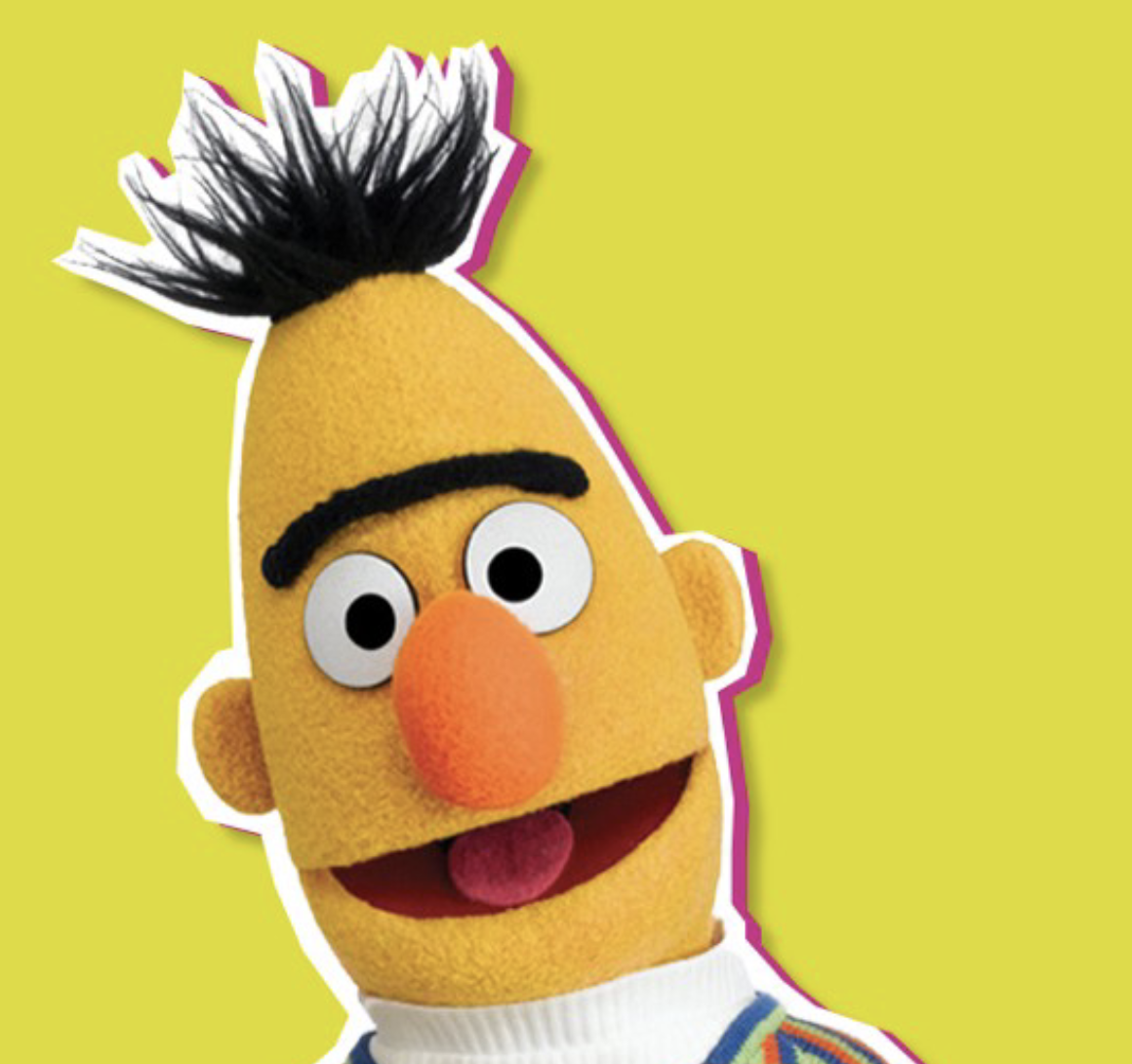
Self-Supervised Knowledge Triplet Learning for Zero-Shot Question Answering
Pratyay Banerjee, Chitta Baral,

Self-Supervised Meta-Learning for Few-Shot Natural Language Classification Tasks
Trapit Bansal, Rishikesh Jha, Tsendsuren Munkhdalai, Andrew McCallum,
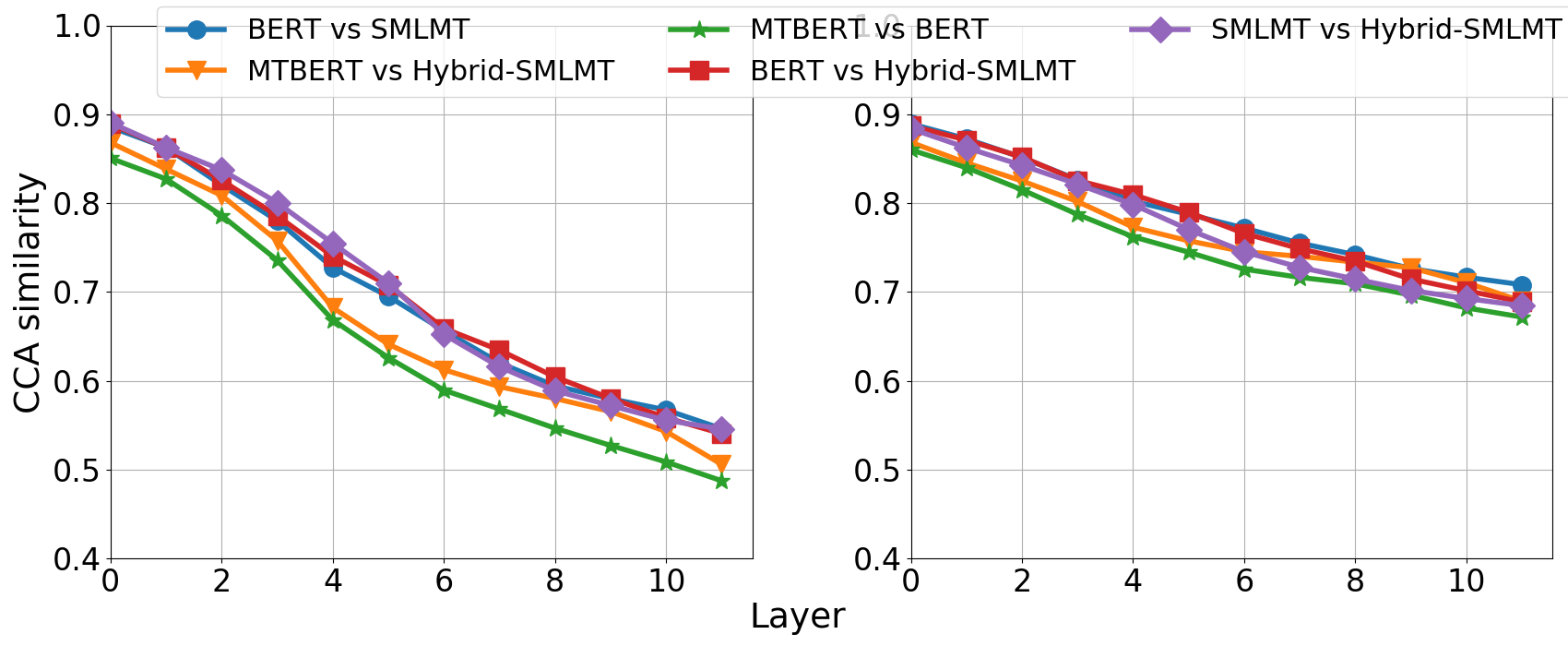
OpenUE: An Open Toolkit of Universal Extraction from Text
Ningyu Zhang, Shumin Deng, Zhen Bi, Haiyang Yu, Jiacheng Yang, Mosha Chen, Fei Huang, Wei Zhang, Huajun Chen,
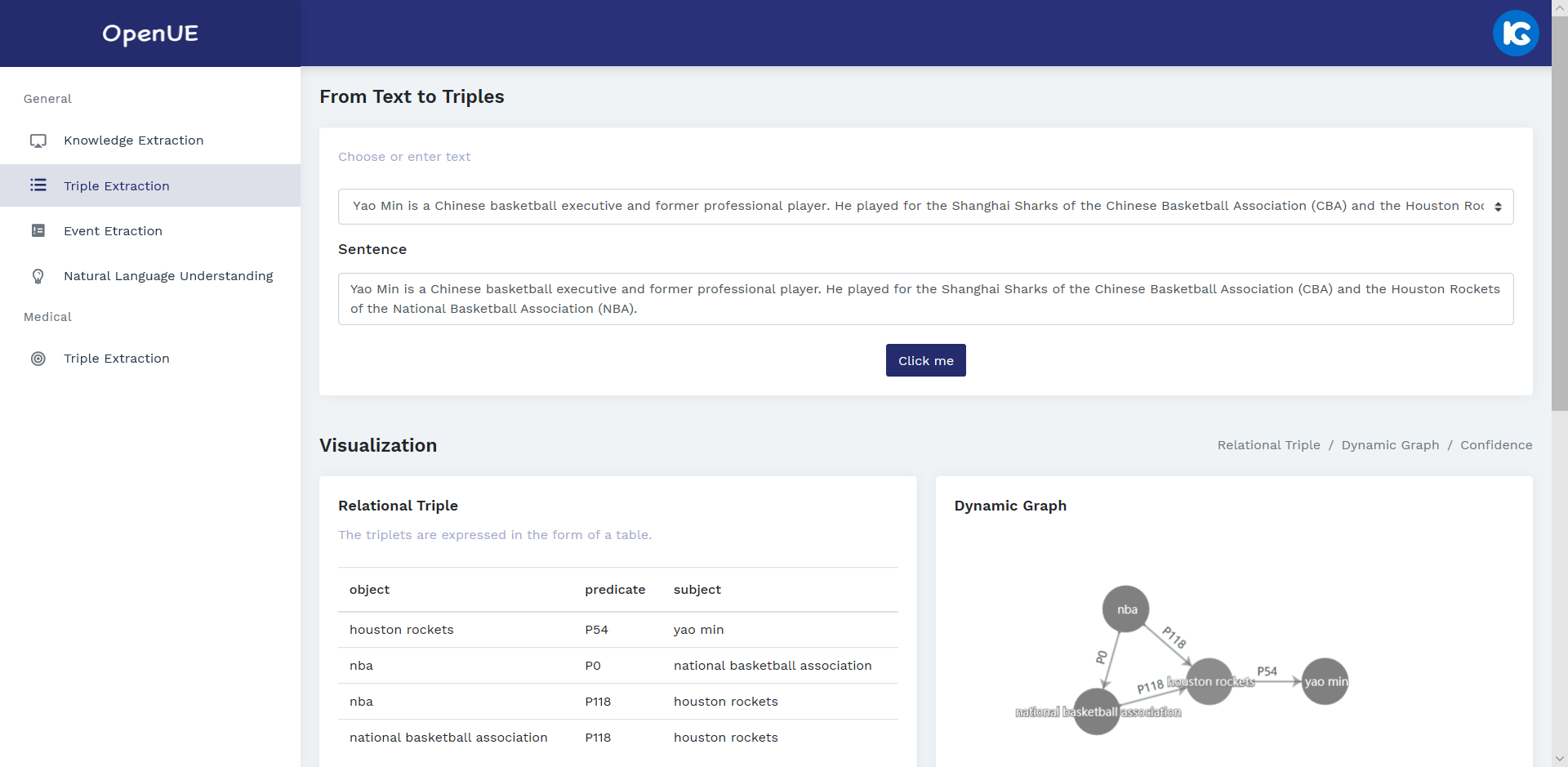