Learning to Ignore: Long Document Coreference with Bounded Memory Neural Networks
Shubham Toshniwal, Sam Wiseman, Allyson Ettinger, Karen Livescu, Kevin Gimpel
Discourse and Pragmatics Short Paper
You can open the pre-recorded video in a separate window.
Abstract:
Long document coreference resolution remains a challenging task due to the large memory and runtime requirements of current models. Recent work doing incremental coreference resolution using just the global representation of entities shows practical benefits but requires keeping all entities in memory, which can be impractical for long documents. We argue that keeping all entities in memory is unnecessary, and we propose a memory-augmented neural network that tracks only a small bounded number of entities at a time, thus guaranteeing a linear runtime in length of document. We show that (a) the model remains competitive with models with high memory and computational requirements on OntoNotes and LitBank, and (b) the model learns an efficient memory management strategy easily outperforming a rule-based strategy
NOTE: Video may display a random order of authors.
Correct author list is at the top of this page.
Connected Papers in EMNLP2020
Similar Papers
Coreferential Reasoning Learning for Language Representation
Deming Ye, Yankai Lin, Jiaju Du, Zhenghao Liu, Peng Li, Maosong Sun, Zhiyuan Liu,

Distilling Multiple Domains for Neural Machine Translation
Anna Currey, Prashant Mathur, Georgiana Dinu,
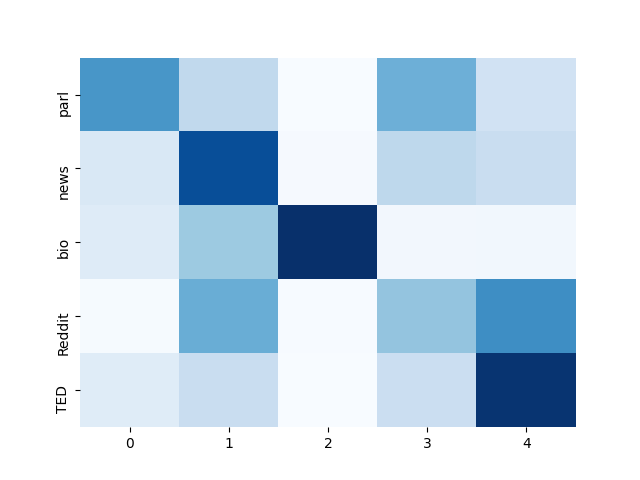